TILP: Differentiable Learning of Temporal Logical Rules on Knowledge Graphs.
arXiv (Cornell University)(2024)
摘要
Compared with static knowledge graphs, temporal knowledge graphs (tKG), whichcan capture the evolution and change of information over time, are morerealistic and general. However, due to the complexity that the notion of timeintroduces to the learning of the rules, an accurate graph reasoning, e.g.,predicting new links between entities, is still a difficult problem. In thispaper, we propose TILP, a differentiable framework for temporal logical ruleslearning. By designing a constrained random walk mechanism and the introductionof temporal operators, we ensure the efficiency of our model. We presenttemporal features modeling in tKG, e.g., recurrence, temporal order, intervalbetween pair of relations, and duration, and incorporate it into our learningprocess. We compare TILP with state-of-the-art methods on two benchmarkdatasets. We show that our proposed framework can improve upon the performanceof baseline methods while providing interpretable results. In particular, weconsider various scenarios in which training samples are limited, data isbiased, and the time range between training and inference are different. In allthese cases, TILP works much better than the state-of-the-art methods.
更多查看译文
关键词
Temporal Data Mining,Structure Learning,Description Logics,Probabilistic Learning,Graphical Models
AI 理解论文
溯源树
样例
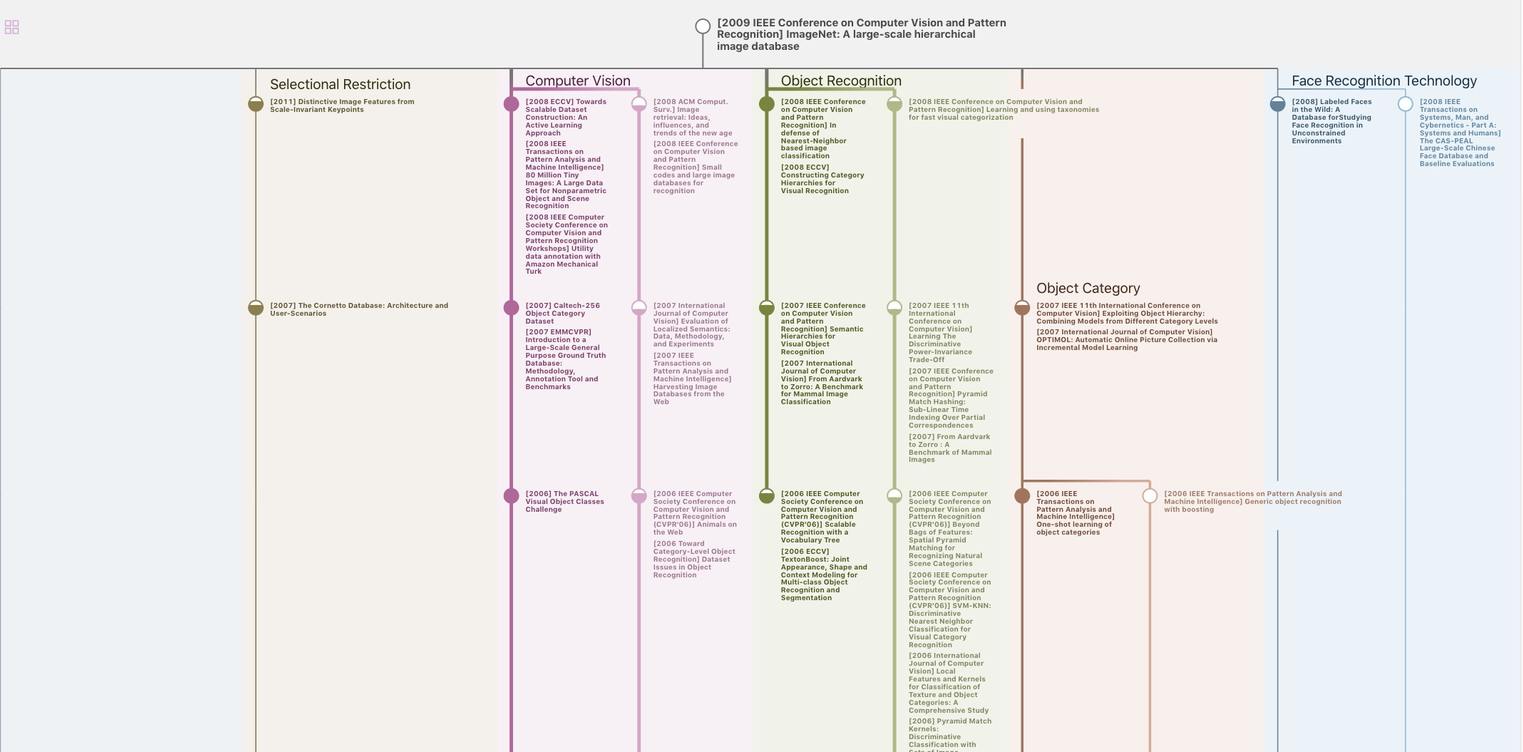
生成溯源树,研究论文发展脉络
Chat Paper
正在生成论文摘要