Calibrating Transformers via Sparse Gaussian Processes
ICLR 2023(2023)
摘要
Transformer models have achieved profound success in prediction tasks in a wide range of applications in natural language processing, speech recognition and computer vision. Extending Transformer’s success to safety-critical domains requires calibrated uncertainty estimation which remains under-explored. To address this, we propose Sparse Gaussian Process attention (SGPA), which performs Bayesian inference directly in the output space of multi-head attention blocks (MHAs) in transformer to calibrate its uncertainty. It replaces the scaled dot-product operation with a valid kernel and uses sparse Gaussian processes (SGP) techniques to approximate the posterior processes of MHA outputs. Empirically, on a suite of prediction tasks on text, images and graphs, SGPA-based Transformers achieve competitive predictive accuracy, while noticeably improving both in-distribution calibration and out-of-distribution robustness and detection.
更多查看译文
关键词
Transformers,Gaussian processes,Bayesian neural networks,uncertainty estimation,variational inference
AI 理解论文
溯源树
样例
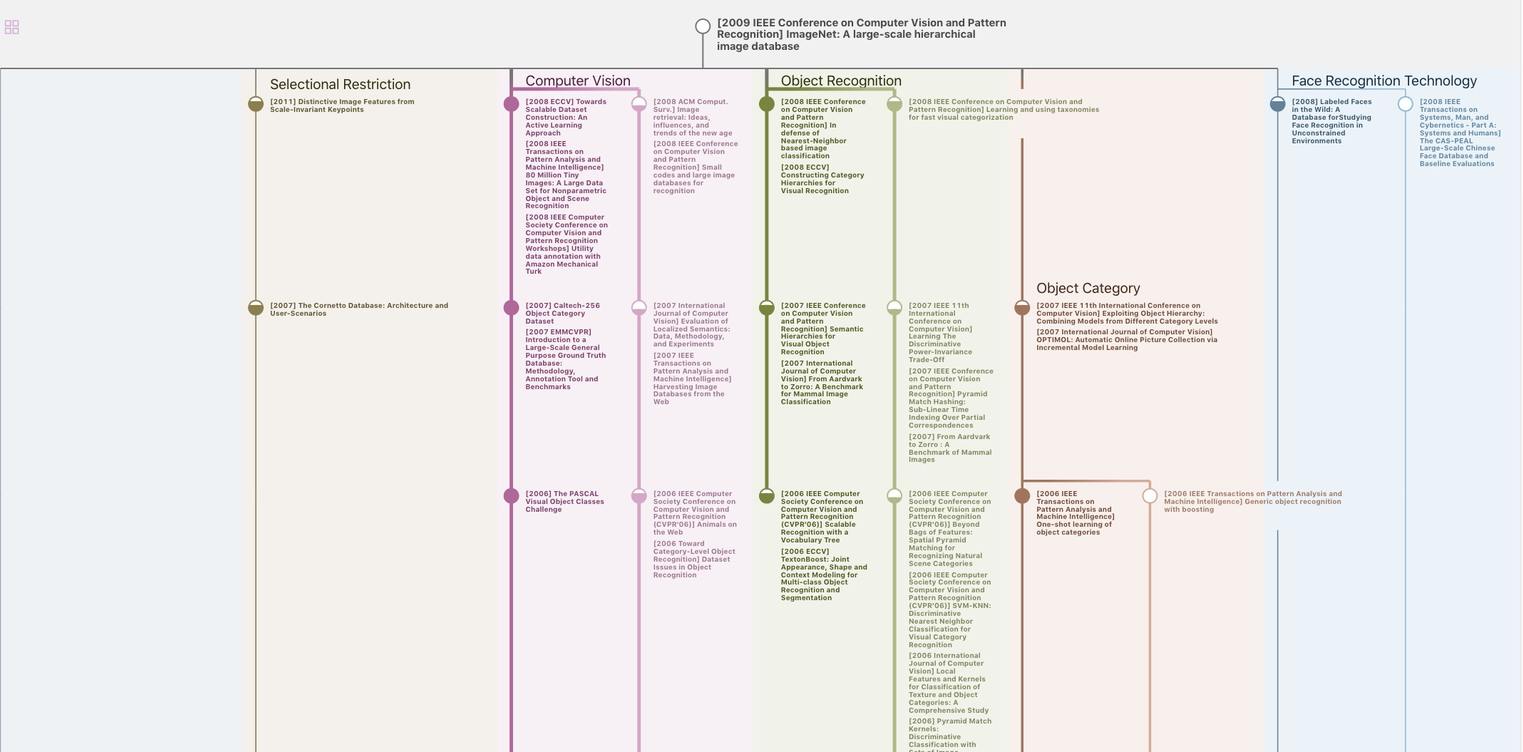
生成溯源树,研究论文发展脉络
Chat Paper
正在生成论文摘要