Energy-based Out-of-Distribution Detection for Graph Neural Networks
ICLR 2023(2023)
摘要
Representation learning on semi-structured data, e.g., graphs, has become a central problem in deep learning community as relational structures are pervasive in real situations and induce data inter-dependence that hinders trivial adaptation of existing approaches in other domains where the inputs are assumed to be i.i.d. sampled. However, current models in this regime mostly focus on improving testing performance of in-distribution data and largely ignores the potential risk w.r.t. out-of-distribution (OOD) testing samples that may cause negative outcome if the model is overconfident in prediction on them. In this paper, we identify a provably effective OOD discriminator based on an energy function directly extracted from a graph neural network trained with standard supervised classification loss. This paves a way for a simple and efficient OOD detection model for GNN-based semi-supervised learning on graphs, which we call GNN-Safe. It also has nice theoretical properties that guarantee an overall distinguishable margin between the detection scores for in-distribution and OOD samples, which, more critically, can be further strengthened by a non-learning-based structured propagation scheme. Extensive experiments over five real-world datasets validate the practical efficacy of the proposed model for detecting various OOD instances that are inter-connected in a graph with up to 17.0% improvement on average AUROC over competitive peer models and without sacrificing in-distribution testing accuracy.
更多查看译文
关键词
graph neural networks,out-of-distribution detection,semi-supervised node classification,energy model
AI 理解论文
溯源树
样例
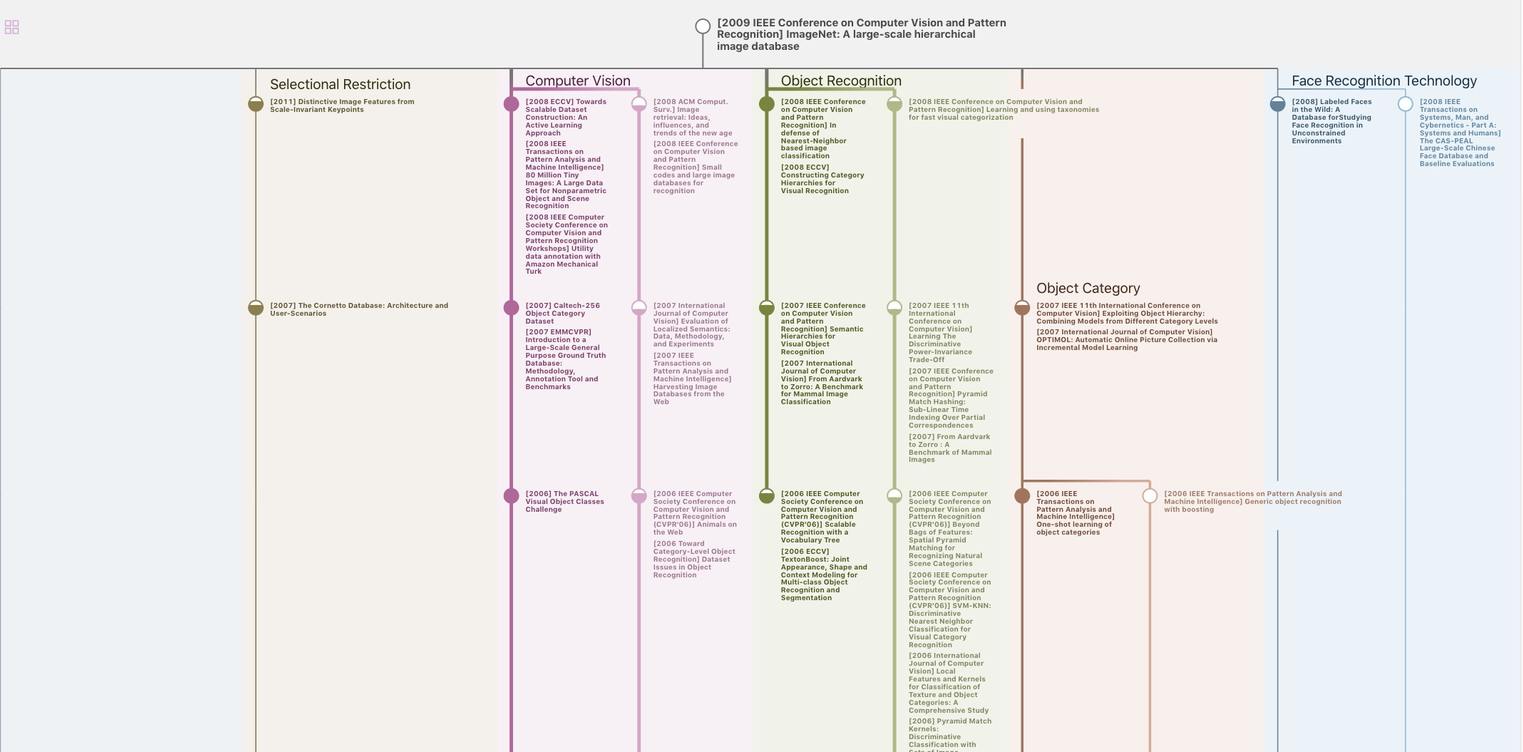
生成溯源树,研究论文发展脉络
Chat Paper
正在生成论文摘要