Dynamic Update-to-Data Ratio: Minimizing World Model Overfitting
ICLR 2023(2023)
摘要
Early stopping based on the validation set performance is a popular approach to find the right balance between under- and overfitting in the context of supervised learning. However, in reinforcement learning, even for supervised sub-problems such as world model learning, early stopping is not applicable as the dataset is continually evolving. As a solution, we propose a new general method that dynamically adjusts the update to data (UTD) ratio during training based on under- and overfitting detection on a small subset of the continuously collected experience not used for training. We apply our method to DreamerV2, a state-of-the-art model-based reinforcement learning algorithm, and evaluate it on the DeepMind Control Suite and the Atari 100k benchmark. The results demonstrate that one can better balance under- and overestimation by adjusting the UTD ratio with our approach compared to the default setting in DreamerV2 and that it is competitive with an extensive hyperparameter search which is not feasible for many applications. Our method eliminates the need to set the UTD hyperparameter by hand and even leads to a higher robustness with regard to other learning-related hyperparameters further reducing the amount of necessary tuning.
更多查看译文
关键词
dynamic,ratio,model,update-to-data
AI 理解论文
溯源树
样例
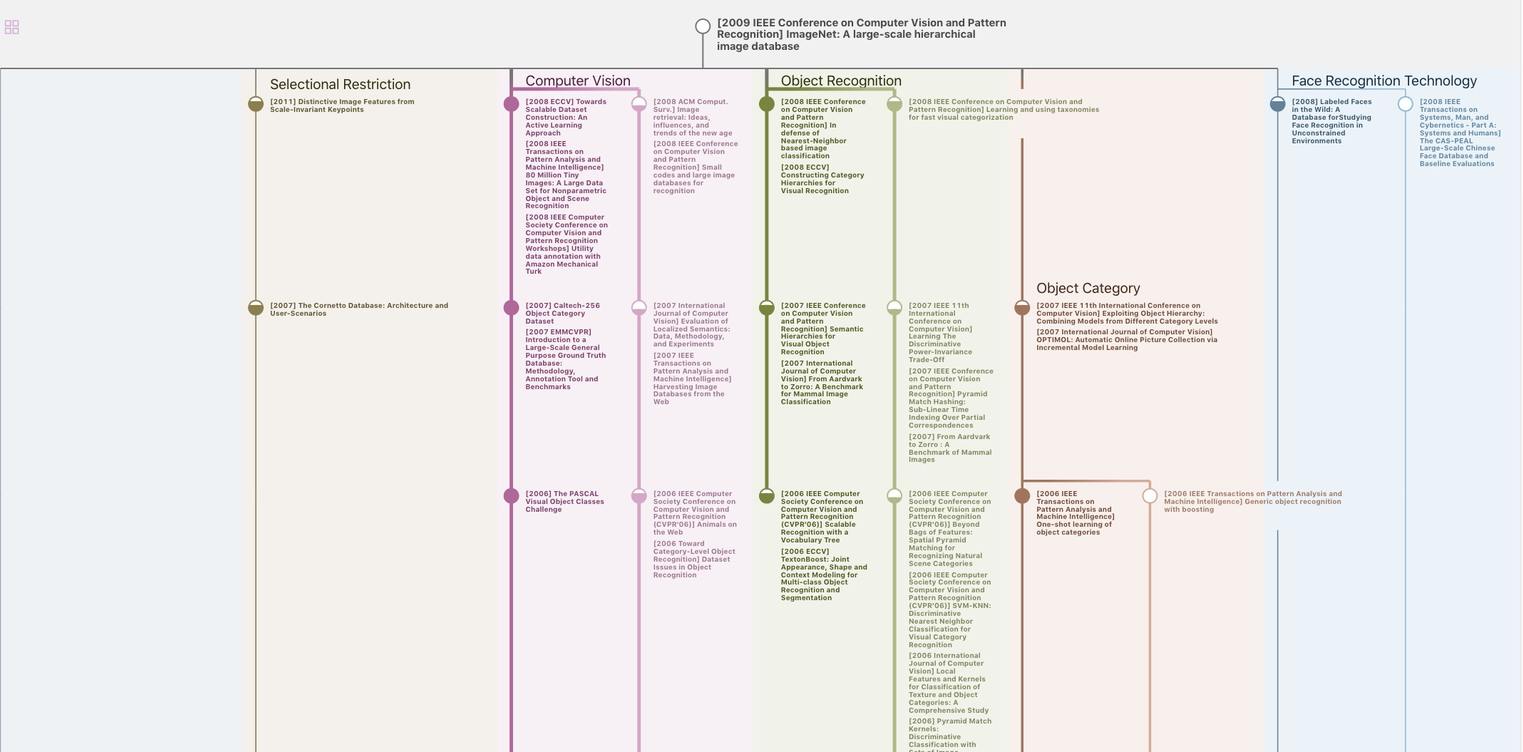
生成溯源树,研究论文发展脉络
Chat Paper
正在生成论文摘要