Evidential Uncertainty and Diversity Guided Active Learning for Scene Graph Generation
ICLR 2023(2023)
摘要
Scene Graph Generation (SGG) has already shown its great potential in various downstream tasks, but it comes at the price of a prohibitively expensive annotation process. To reduce the annotation cost, we propose using Active Learning (AL) for sampling the most informative data. However, directly porting current AL methods to the SGG task poses the following challenges: 1) unreliable uncertainty estimates, and 2) data bias problems. To deal with these challenges, we propose EDAL (\textbf{E}vidential Uncertainty and \textbf{D}iversity Guided Deep \textbf{A}ctive \textbf{L}earning), a novel AL framework tailored for the SGG task. For challenge 1), we start with Evidential Deep Learning (EDL) coupled with a global relationship mining approach to estimate uncertainty, which can effectively overcome the perturbations of open-set relationships and background-relationships to obtain reliable uncertainty estimates. To address challenge 2), we seek the diversity-based method and design the Context Blocking Module (CBM) and Image Blocking Module (IBM) to alleviate context-level bias and image-level bias, respectively. Experiments show that our AL framework can approach the performance of a fully supervised SGG model with only about $10\%$ annotation cost. Furthermore, our ablation studies indicate that introducing AL into the SGG will face many challenges not observed in other vision tasks that are successfully overcome by our new modules.
更多查看译文
关键词
Active learning,Scene graph generation,Uncertainty estimation
AI 理解论文
溯源树
样例
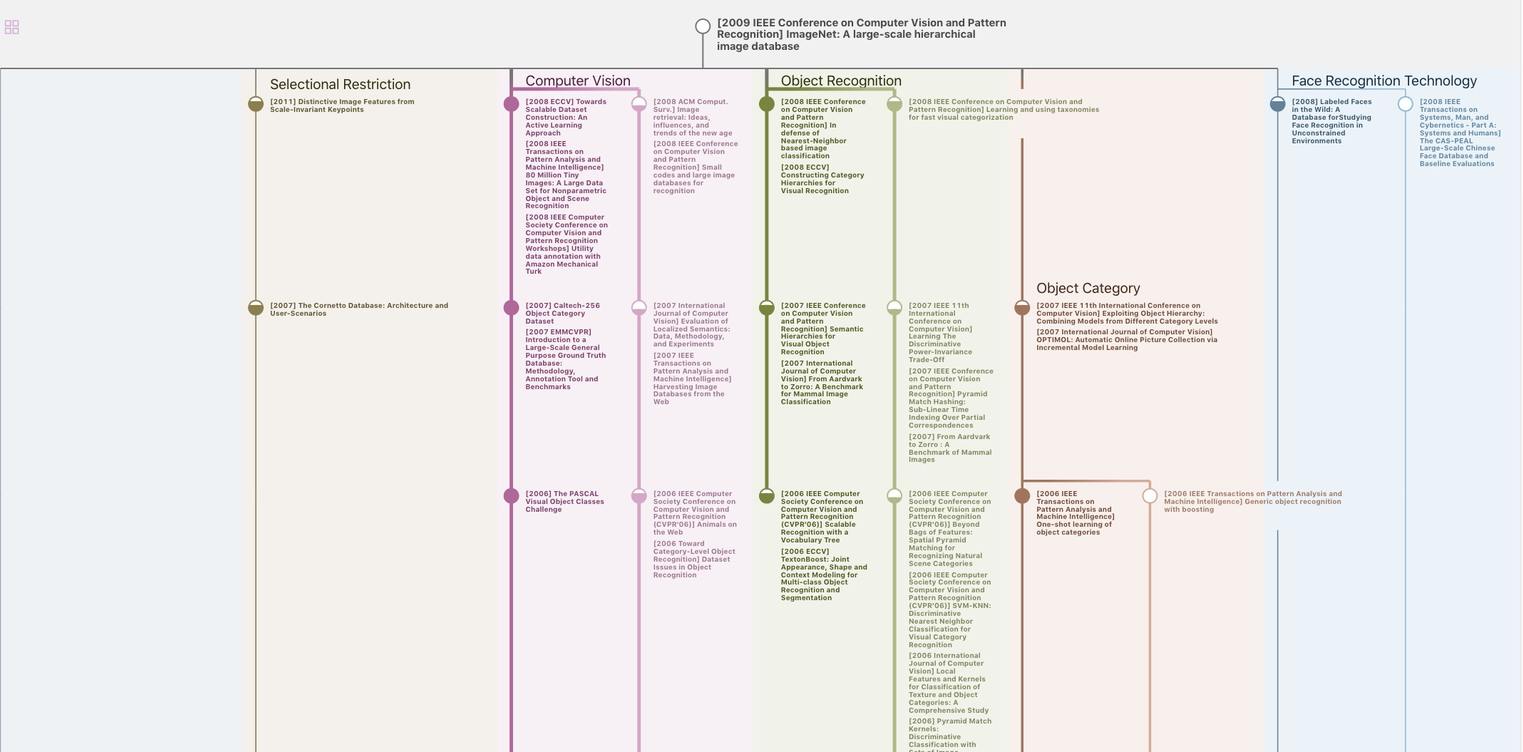
生成溯源树,研究论文发展脉络
Chat Paper
正在生成论文摘要