Combating Exacerbated Heterogeneity for Robust Decentralized Models
ICLR 2023(2023)
摘要
The emerging privacy and security issues in real-world applications motivate us to pursue the adversarially robust federated models. However, the straightforward combination between adversarial training and federated learning in one framework, usually induces the undesired robustness deterioration. We discover that the attribution behind this phenomenon is the generated adversarial data could exacerbate the data heterogeneity among local clients, making the wrapped federated learning perform poorly. To deal with this problem, we propose a novel framework termed as Slack Federated Adversarial Training (SFAT), assigning the client-wise slack during aggregation to combat the intensified heterogeneity. Theoretically, we analyze the convergence of the proposed method to properly relax the objective when combining federated learning and adversarial training. Experimentally, we verify the rationality and effectiveness of SFAT on various benchmarked and real-world datasets with different adversarial training and federated optimization methods.
更多查看译文
AI 理解论文
溯源树
样例
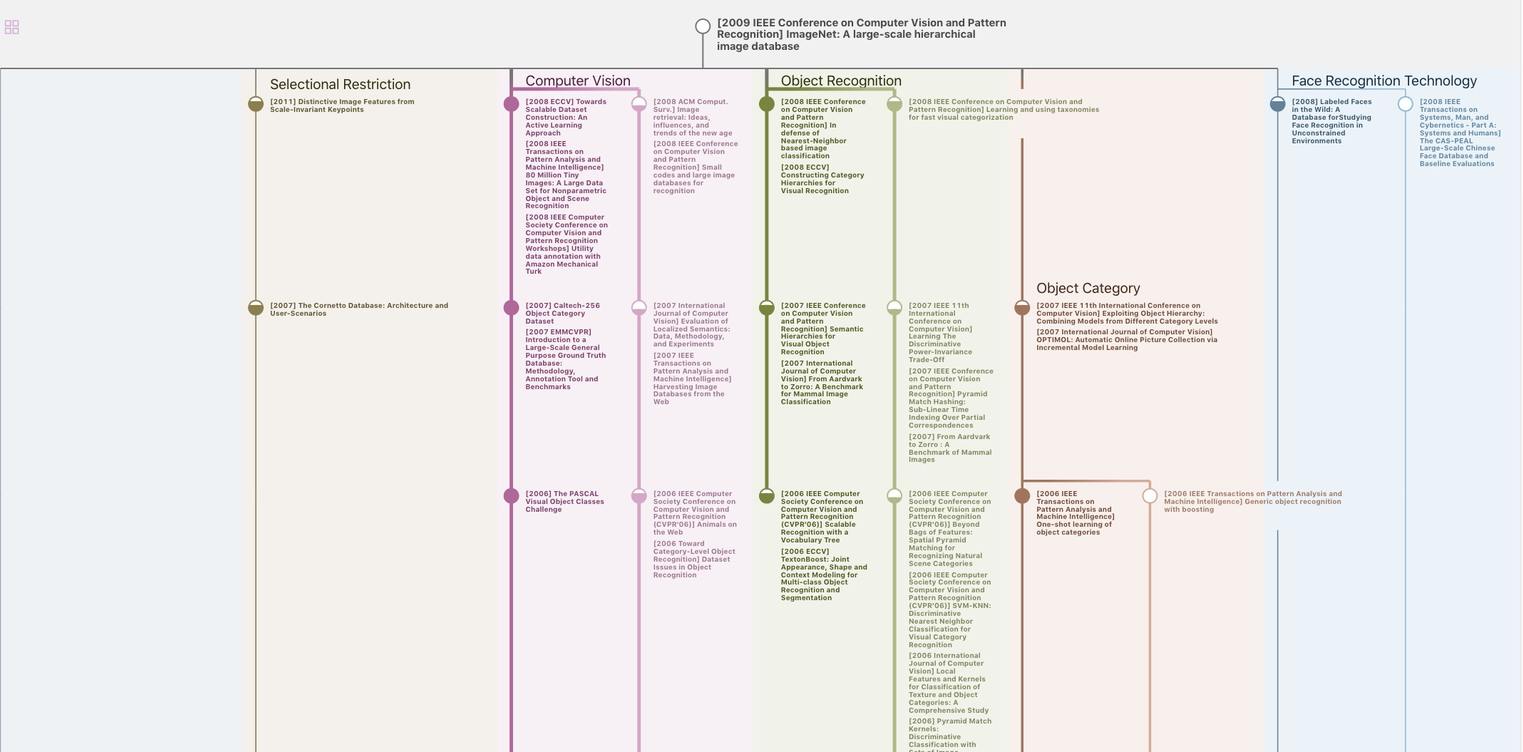
生成溯源树,研究论文发展脉络
Chat Paper
正在生成论文摘要