Multivariate Time-series Imputation with Disentangled Temporal Representations
ICLR 2023(2023)
摘要
Multivariate time series often faces the problem of missing value. Many time series imputation methods have been developed in the literature. However, these methods all rely on an entangled representation to model dynamics of time series, which may fail to fully exploit the multiple factors (e.g., periodic patterns) contained in the time series. Moreover, the entangled representation usually has no semantic meaning, and thus they often lack interpretability. In addition, many recent models are proposed to deal with the whole time series to capture cross-channel correlations and identify temporal dynamics, but they are not scalable to large-scale datasets. Different from existing approaches, we propose TIDER, a novel matrix factorization-based method with disentangled temporal representations that account for multiple factors, namely trend, seasonality, and local bias, to model complex dynamics. The learned disentanglement makes the imputation process more reliable and offers explainability for imputation results. Moreover, TIDER is scalable to large datasets. Empirical results show that our method not only outperforms existing approaches by notable margins on three real-world datasets, but also scales well to large datasets on which existing deep learning based methods struggle. Disentanglement validation experiments further demonstrate the robustness of our model in obtaining accurate and explainable disentangled components.
更多查看译文
关键词
multivariate time-series imputation,disentangled representation
AI 理解论文
溯源树
样例
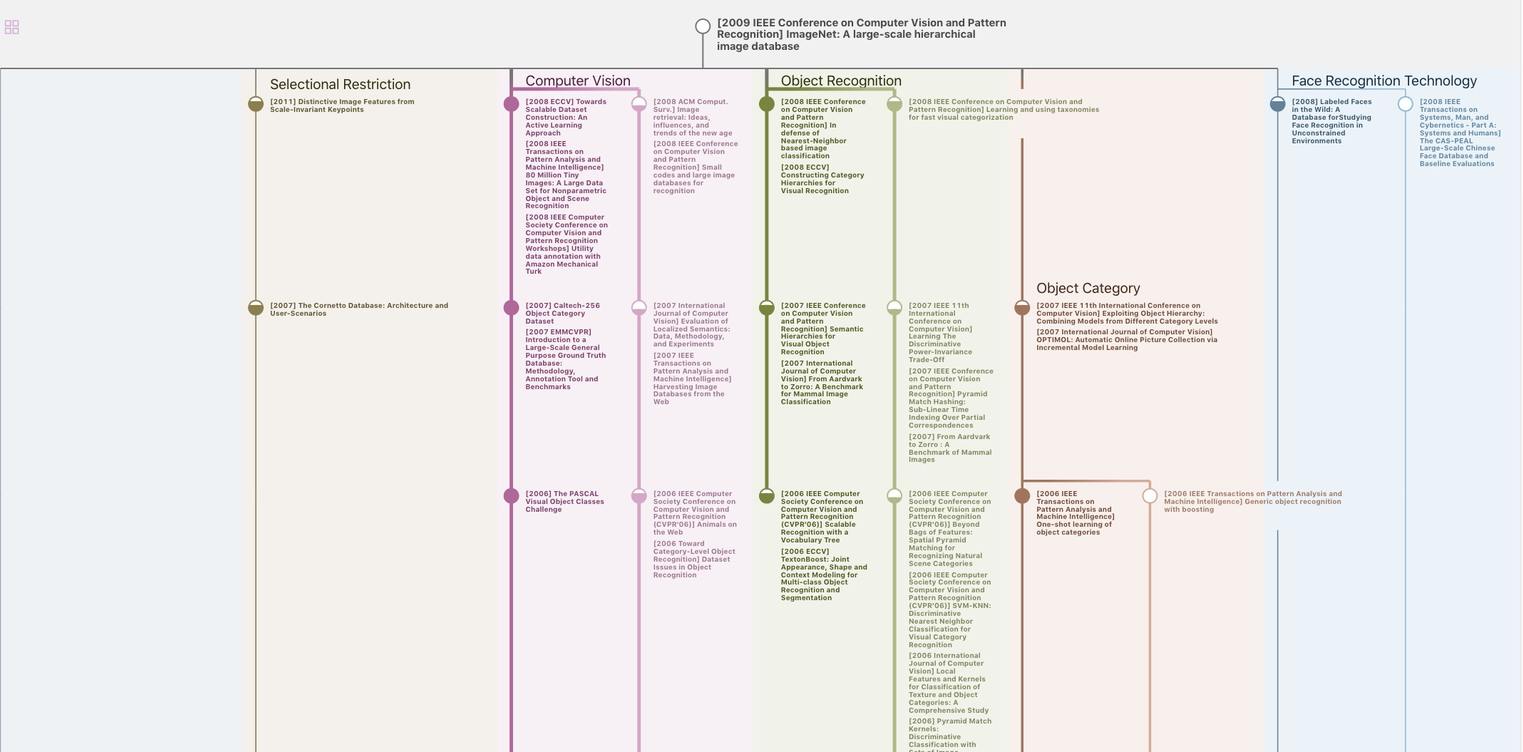
生成溯源树,研究论文发展脉络
Chat Paper
正在生成论文摘要