Combined Deep Priors with Low-Rank Tensor Factorization for Hyperspectral Image Restoration
IEEE geoscience and remote sensing letters(2023)
摘要
Mixed noise pollution severely disturbs hyperspectral image (HSI) processing and applications. Plenty of algorithms have been developed to address this issue via two strategies: model-driven or data-driven strategy. However, model-driven methods exist in the highly time-consuming weakness of iterative optimization and unstable sensitivity of setting parameters. Data-driven methods usually perform poor due to the overfitting effects. To solve these issues, we combine both the deep denoising priors with low-rank tensor factorization (DP-LRTF) for HSI restoration. The proposed method uses Tucker tensor factorization to depict the global spectral low-rank constraint. Then the spectral orthogonal basis and spatial reduced factor are optimized by two deep denoising priors, respectively. Through this integrated strategy, we can simultaneously exploit the intrinsic low-rank property of HSI, and utilize the powerful feature extraction ability by deep learning for HSI restoration. Compared with model-driven and data-driven methods, DP-LRTF outperforms on HSI mixed noise removal and execution efficiency for various simulated/real experiments.
更多查看译文
关键词
Noise reduction,Noise measurement,Tensors,Image restoration,Training,Optimization,Indexes,Deep denoising priors,hyperspectral image (HSI),low-tank,restoration,Tucker-1 tensor factorization
AI 理解论文
溯源树
样例
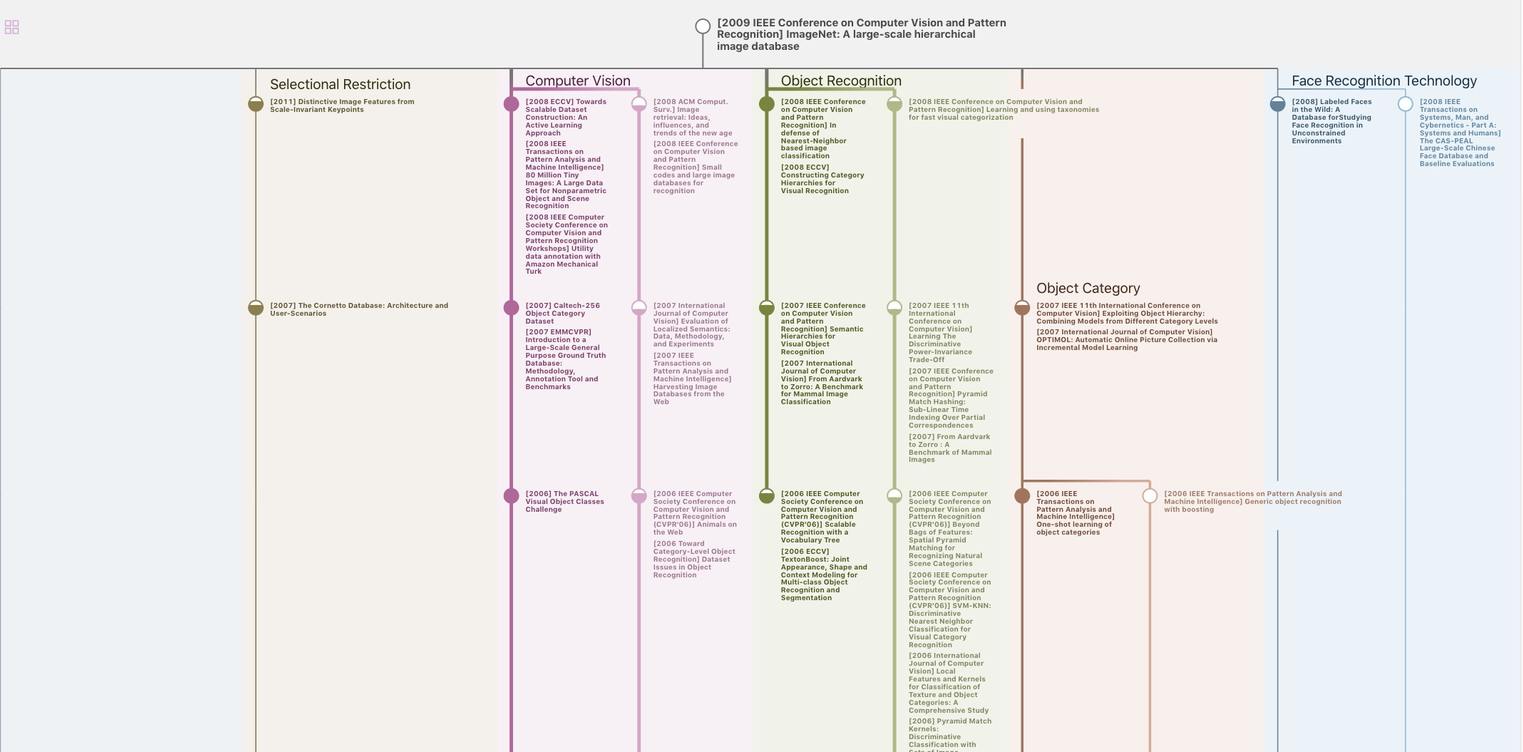
生成溯源树,研究论文发展脉络
Chat Paper
正在生成论文摘要