A Machine Learning-Based Method for Downscaling All-Sky Downward Surface Shortwave Radiation Over Complex Terrain
IEEE Geoscience and Remote Sensing Letters(2023)
摘要
In regions with complex terrain, high-spatial-resolution downward surface shortwave radiation (DSSR) is critical for monitoring mountain ecological processes and for environmental management. However, currently available DSSR products are often too coarse (from a kilometer to tens of kilometers) to capture the spatial heterogeneity of DSSR in topographically complex regions. To address this issue, this study proposes a new downscaling method for all-sky instantaneous DSSR, employing a machine learning (ML) method, top-of-atmosphere reflectance, and topographic data. The method is used to downscale the 5-km Himawari-8 (H-8) DSSR product to the Sentinel 10 m scale. A region of Southwest China was chosen as a case study. Validated by field measurements from nine stations in 2020, the downscaled DSSR showed improvements in the mean bias error (MBE), mean absolute error (MAE), and root-mean-square error (RMSE) of 32.74%, 9.31%, and 6.34%, respectively, when compared with the original product. The downscaled DSSR can be generated in all-sky conditions. In general, this method successfully captures high-resolution DSSR over complex terrain and should be helpful for related studies.
更多查看译文
关键词
Complex terrain,downscaling,downward surface shortwave radiation (DSSR),Himawari-8,random forest (RF),Sentinel-2
AI 理解论文
溯源树
样例
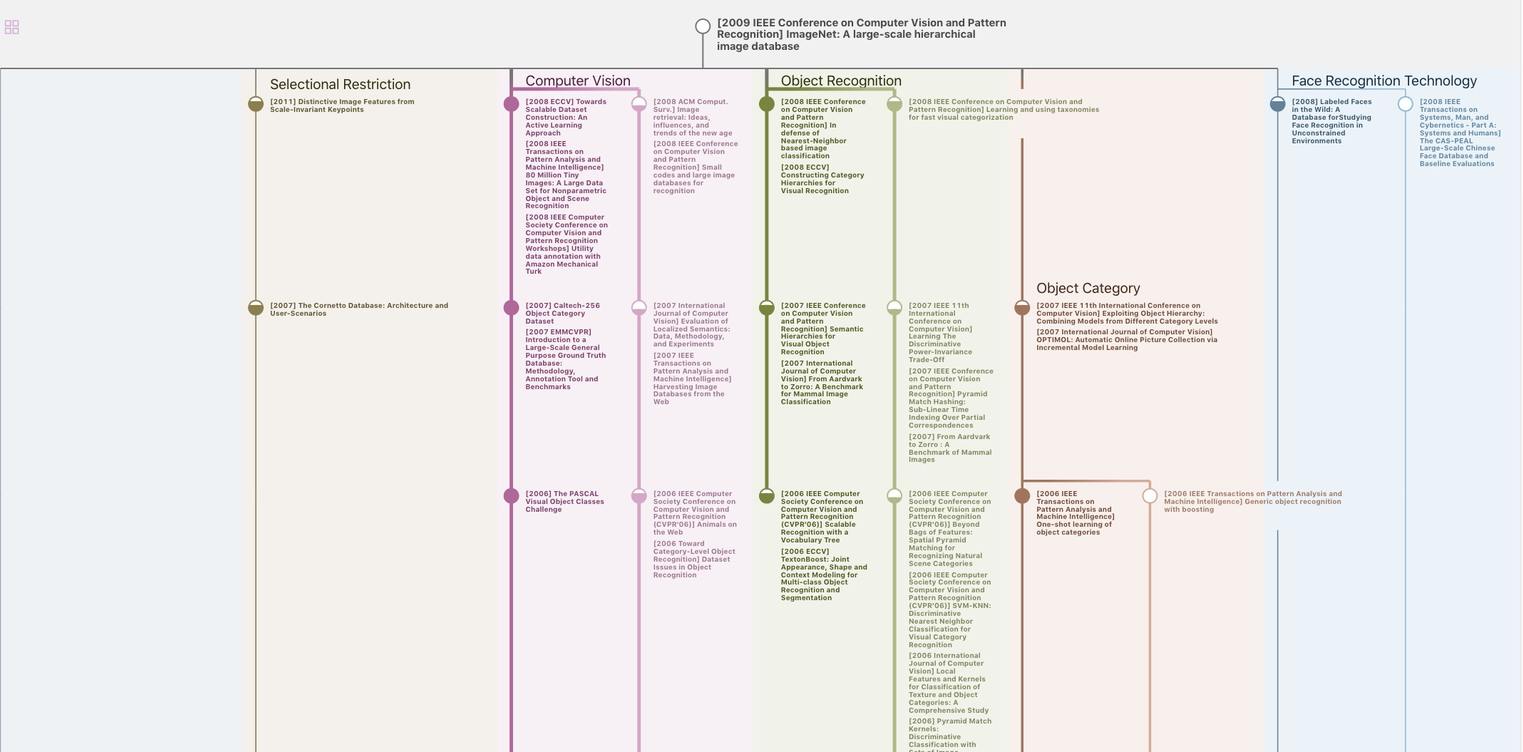
生成溯源树,研究论文发展脉络
Chat Paper
正在生成论文摘要