Controlling Sequential Hybrid Evolutionary Algorithm by Q-Learning [Research Frontier] [Research Frontier]
IEEE Computational Intelligence Magazine(2023)
摘要
Many state-of-the-art evolutionary algorithms (EAs) can be categorized as sequential hybrid EAs, in which various EAs are sequentially executed. The timing to switch from one EA to another is critical to the performance of the hybrid EA because the switching time determines the allocation of computational resources and thereby it helps balance exploration and exploitation. In this article, a framework for adaptive parameter control for hybrid EAs is proposed, in which the switching time is controlled by a learned agent rather than a manually designed scheme. First the framework is applied to an adaptive differential evolution algorithm, LSHADE, to control when to use the scheme to reduce the population. Then the framework is applied to the algorithm that won the CEC 2018 competition, i.e., the hybrid sampling evolution strategy (HSES), to control when to switch from the univariate sampling phase to the Covariance Matrix Adaptation Evolution Strategy phase. The agents for parameter control in LSHADE and HSES are trained by using Q-learning and deep Q-learning to obtain the learned algorithms Q-LSHADE and DQ-HSES. The results of experiments on the CEC 2014 and 2018 test suites show that the learned algorithms significantly outperform their counterparts and some state-of-the-art EAs.
更多查看译文
关键词
Q-learning,Sociology,Switches,Evolutionary computation,Control systems,Timing,Resource management
AI 理解论文
溯源树
样例
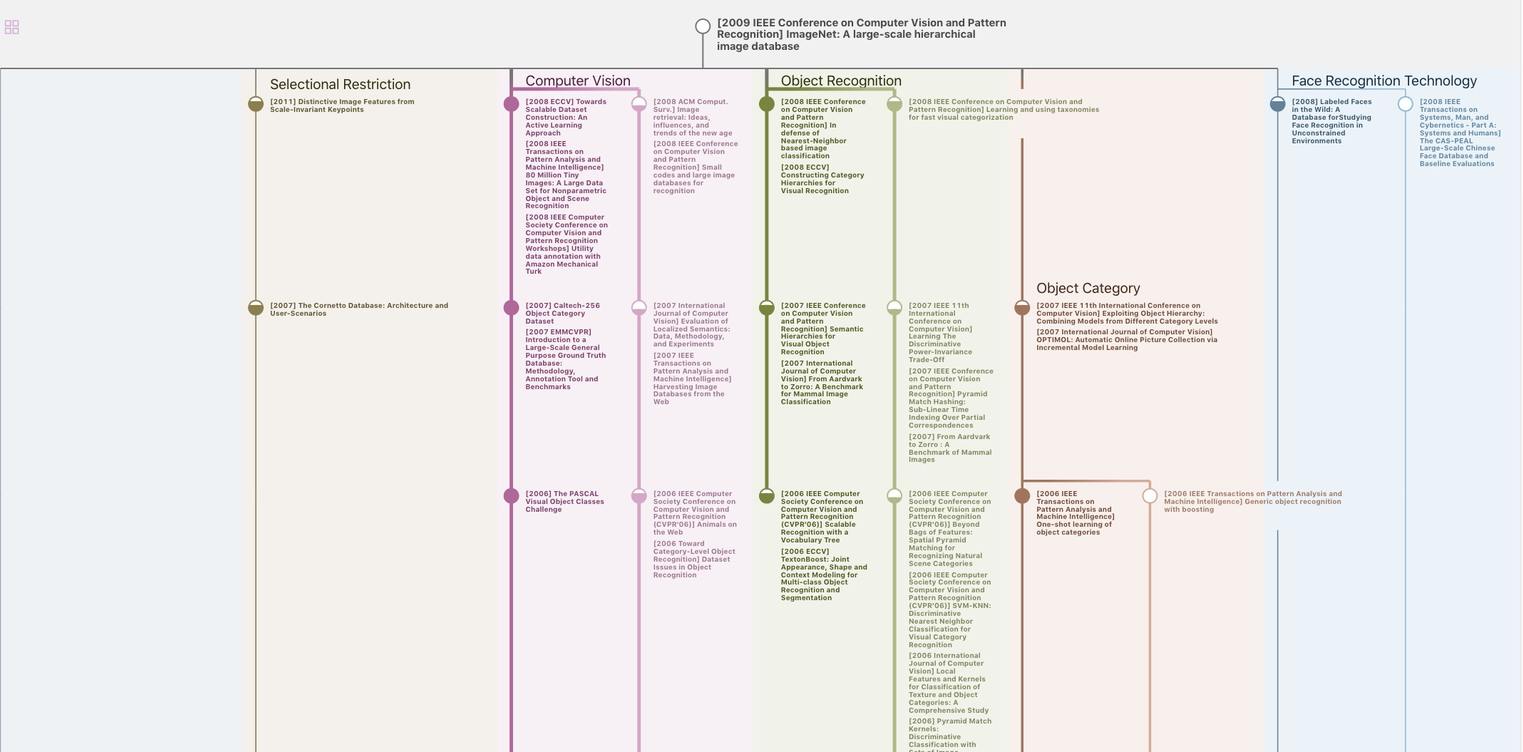
生成溯源树,研究论文发展脉络
Chat Paper
正在生成论文摘要