Class-Aware Tiny Object Recognition over Large-Scale 3D Point Clouds
Neurocomputing(2023)
摘要
Although tremendous strides have been made in object recognition over large-scale 3D point clouds, one of the remaining open challenges is detecting tiny objects. The signal and appearance information of tiny objects are generally sparse and insufficient due to their tiny size, which makes the tiny object difficult to be detected with existing studies both in tiny 2D object recognition and general 3D object recognition since 3D topology relations are lost due to feature projection in tiny 2D object recognition and massive background may dominate the feature learner in general 3D object recognition. To the best of our knowledge, we explore tiny object recognition for the first time over large-scale 3D point clouds by designing deep neural network. To cope with this problem, a novel two-stage approach is proposed in this paper, which can effectively learn an informative and discriminative feature representation and efficiently process large-scale point clouds. Specifically, a class-aware filtering strategy is designed to dispense with the redundant background information. After that, we introduce a novel aggregating and sampling scheme in a supervised manner to progressively increase the receptive field for each 3D point, which can adaptively capture useful signals from tiny objects and learn complex geometric structures. Last, we propose a biased loss function that is inversely correlated to the number of tiny object points, thereby effectively handling the sparsity of tiny objects. Extensive experimental results over two real-world data sets validate the effectiveness and efficiency of the proposed approach.
更多查看译文
关键词
Tiny object recognition,Point cloud analysis,Signal sparsity,Deep neural network
AI 理解论文
溯源树
样例
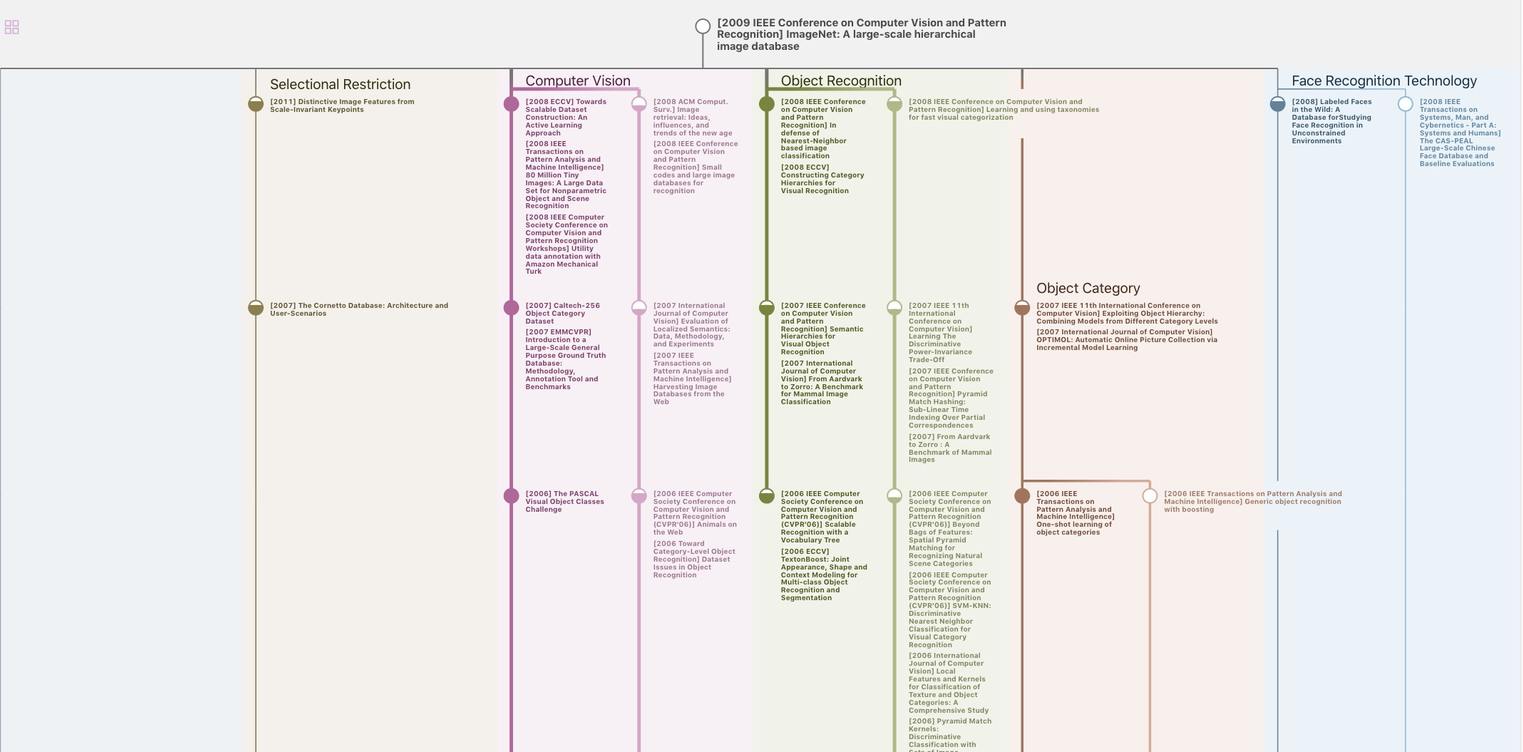
生成溯源树,研究论文发展脉络
Chat Paper
正在生成论文摘要