Expanding Sparse LiDAR Depth and Guiding Stereo Matching for Robust Dense Depth Estimation
IEEE Robotics and Automation Letters(2023)
摘要
Dense depth estimation is an important task for applications, such as object detection, 3-D reconstruction, etc. Stereo matching, as a popular method for dense depth estimation, has been faced with challenges when low textures, occlusions or domain gaps exist. Stereo-LiDAR fusion has recently become a promising way to deal with these challenges. However, due to the sparsity and uneven distribution of the LiDAR depth data, existing stereo-LiDAR fusion methods tend to ignore the data when their density is quite low or they largely differ from the depth predicted from stereo images. To provide a solution to this problem, we propose a stereo-LiDAR fusion method by first expanding the sparse LiDAR depth to a semi-dense depth with RGB image as reference. Then, based on the semi-dense depth, a varying-weight Gaussian guiding method is proposed to deal with the varying reliability of guiding signals. A multi-scale feature extraction and fusion method is further used to enhance the network, which shows superior performance over traditional sparse invariant convolution methods. Experimental results on different public datasets demonstrate our superior accuracy and robustness over the state of the arts.
更多查看译文
关键词
Computer vision for automation,sensor fusion,AI-based methods
AI 理解论文
溯源树
样例
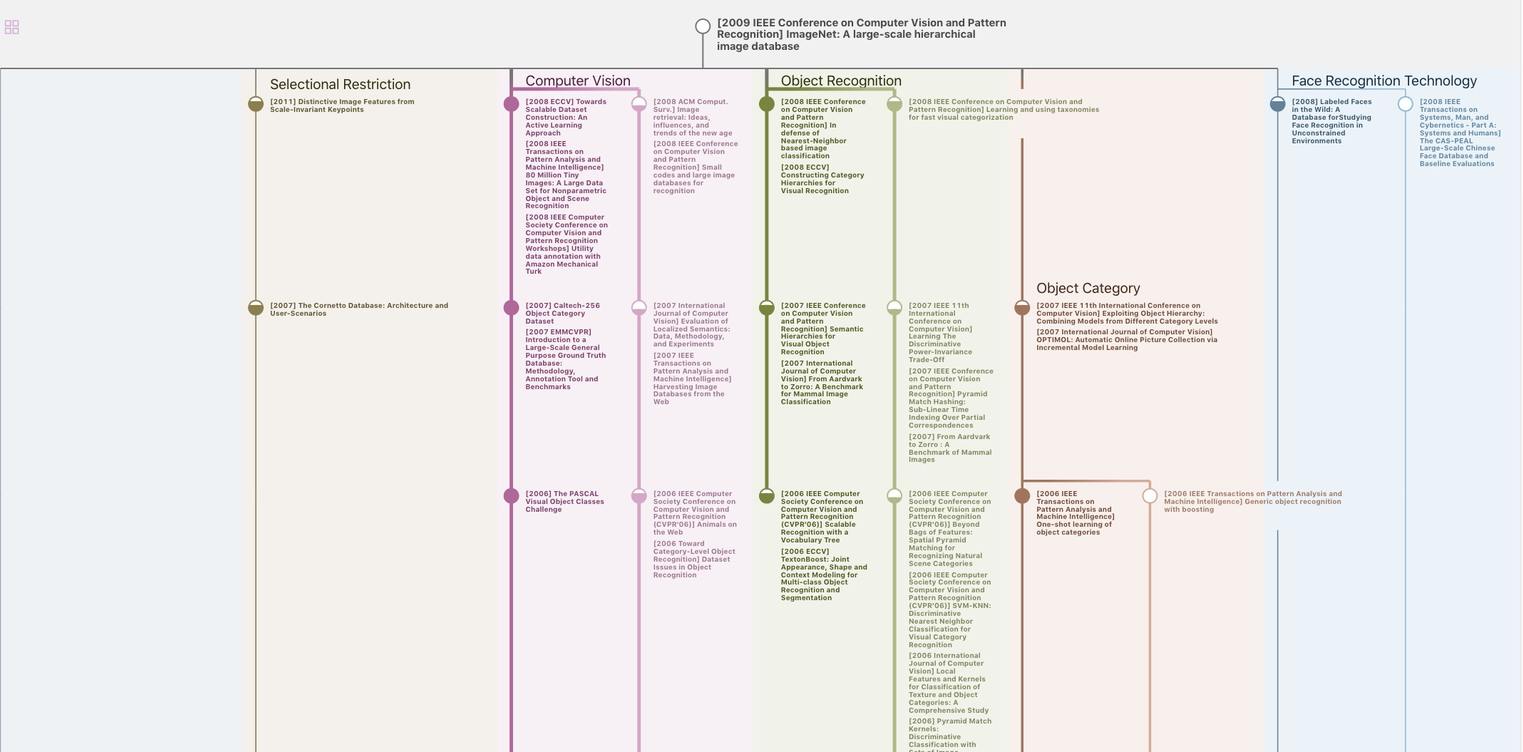
生成溯源树,研究论文发展脉络
Chat Paper
正在生成论文摘要