Creating Probabilistic Forecasts from Arbitrary Deterministic Forecasts using Conditional Invertible Neural Networks
arxiv(2023)
摘要
In various applications, probabilistic forecasts are required to quantify the inherent uncertainty associated with the forecast. However, numerous modern forecasting methods are still designed to create deterministic forecasts. Transforming these deterministic forecasts into probabilistic forecasts is often challenging and based on numerous assumptions that may not hold in real-world situations. Therefore, the present article proposes a novel approach for creating probabilistic forecasts from arbitrary deterministic forecasts. In order to implement this approach, we use a conditional Invertible Neural Network (cINN). More specifically, we apply a cINN to learn the underlying distribution of the data and then combine the uncertainty from this distribution with an arbitrary deterministic forecast to generate accurate probabilistic forecasts. Our approach enables the simple creation of probabilistic forecasts without complicated statistical loss functions or further assumptions. Besides showing the mathematical validity of our approach, we empirically show that our approach noticeably outperforms traditional methods for including uncertainty in deterministic forecasts and generally outperforms state-of-the-art probabilistic forecasting benchmarks.
更多查看译文
关键词
conditional invertible neural networks,arbitrary deterministic forecasts,probabilistic forecasts,neural networks
AI 理解论文
溯源树
样例
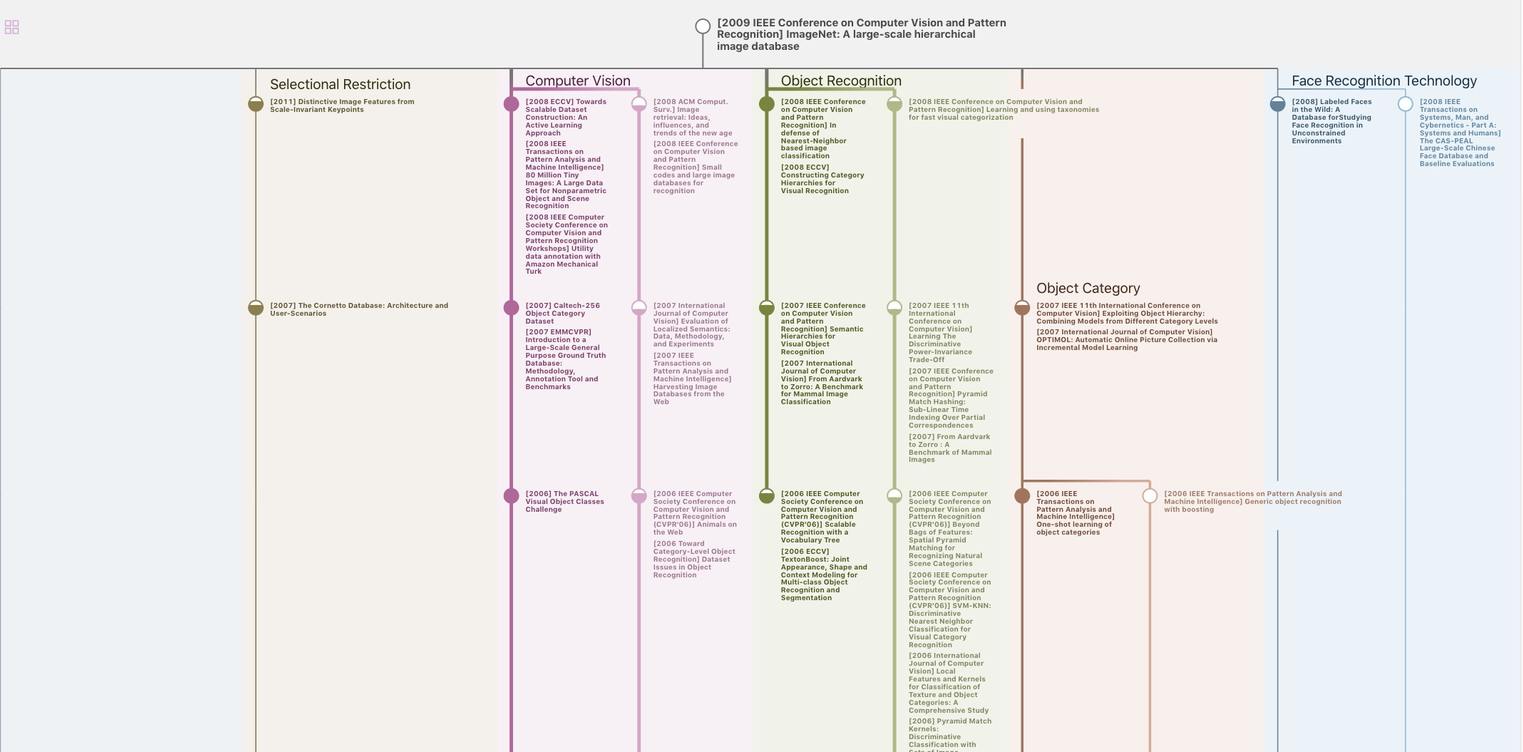
生成溯源树,研究论文发展脉络
Chat Paper
正在生成论文摘要