Nonconvex Distributed Feedback Optimization for Aggregative Cooperative Robotics
arxiv(2023)
摘要
Distributed aggregative optimization is a recently emerged framework in which
the agents of a network want to minimize the sum of local objective functions,
each one depending on the agent decision variable (e.g., the local position of
a team of robots) and an aggregation of all the agents' variables (e.g., the
team barycentre). In this paper, we address a distributed feedback optimization
framework in which agents implement a local (distributed) policy to reach a
steady-state minimizing an aggregative cost function. We propose Aggregative
Tracking Feedback, i.e., a novel distributed feedback optimization law in which
each agent combines a closed-loop gradient flow with a consensus-based dynamic
compensator reconstructing the missing global information. By using tools from
system theory, we prove that Aggregative Tracking Feedback steers the network
to a stationary point of an aggregative optimization problem with (possibly)
nonconvex objective function. The effectiveness of the proposed method is
validated through numerical simulations on a multi-robot surveillance scenario.
更多查看译文
AI 理解论文
溯源树
样例
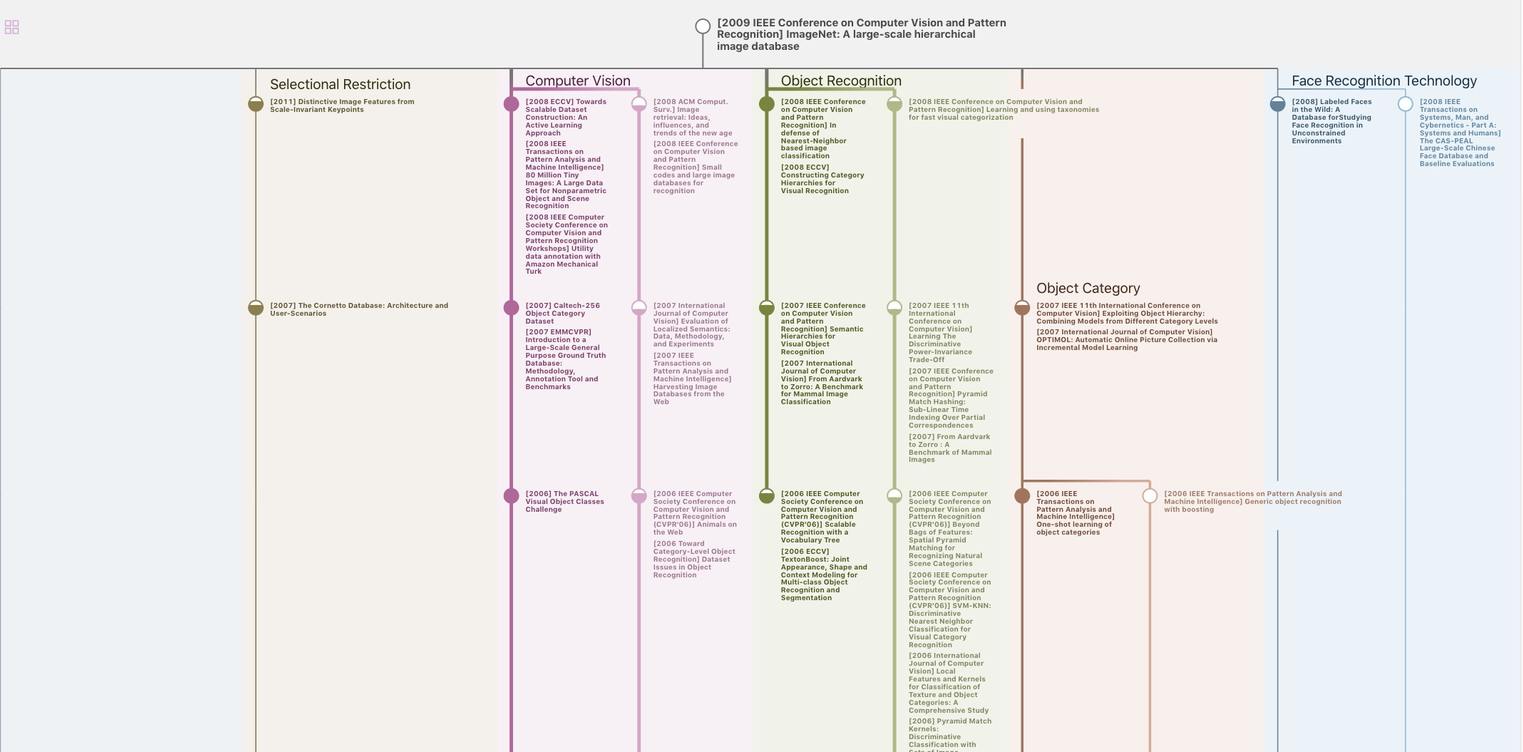
生成溯源树,研究论文发展脉络
Chat Paper
正在生成论文摘要