Towards Scalable EM-based Anomaly Detection For Embedded Devices Through Synthetic Fingerprinting
arxiv(2023)
摘要
Embedded devices are omnipresent in modern networks including the ones operating inside critical environments. However, due to their constrained nature, novel mechanisms are required to provide external, and non-intrusive anomaly detection. Among such approaches, one that has gained traction is based on the analysis of the electromagnetic (EM) signals that get emanated during a device's operation. However, one of the most neglected challenges of this approach is the requirement for manually gathering and fingerprinting the signals that correspond to each execution path of the software/firmware. Indeed, even simple programs are comprised of hundreds if not thousands of branches thus, making the fingerprinting stage an extremely time-consuming process that involves the manual labor of a human specialist. To address this issue, we propose a framework for generating synthetic EM signals directly from the machine code. The synthetic signals can be used to train a Machine Learning based (ML) system for anomaly detection. The main advantage of the proposed approach is that it completely removes the need for an elaborate and error-prone fingerprinting stage, thus, dramatically increasing the scalability of the corresponding protection mechanisms. The experimental evaluations indicate that our method provides high detection accuracy (above 90% AUC score) when employed for the detection of injection attacks. Moreover, the proposed methodology inflicts only a small penalty (-1.3%) in accuracy for the detection of the injection of as little as four malicious instructions when compared to the same methods if real signals were to be used.
更多查看译文
关键词
synthetic fingerprinting,embedded devices,anomaly detection,em-based
AI 理解论文
溯源树
样例
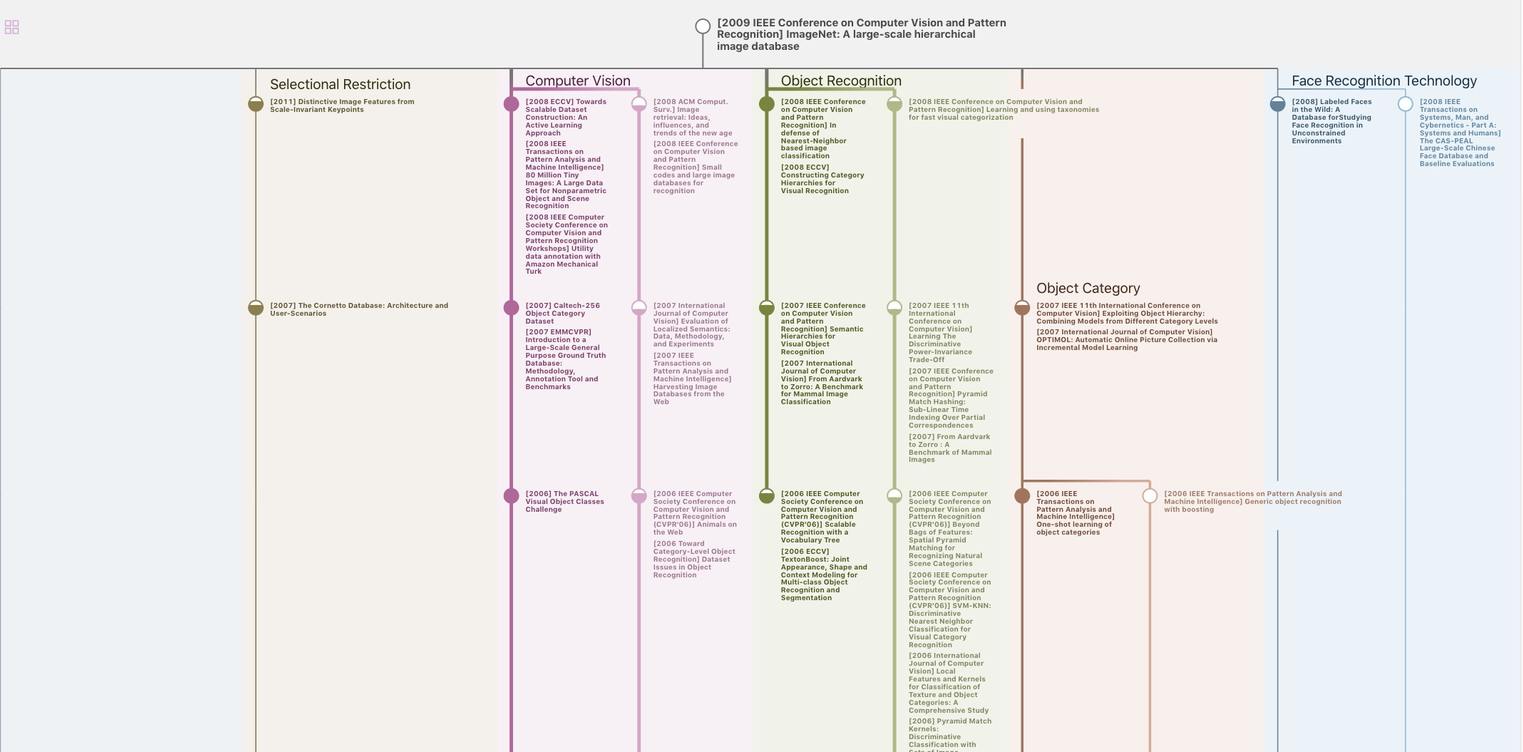
生成溯源树,研究论文发展脉络
Chat Paper
正在生成论文摘要