FastPillars: A Deployment-friendly Pillar-based 3D Detector
arxiv(2023)
摘要
The deployment of 3D detectors strikes one of the major challenges in real-world self-driving scenarios. Existing BEV-based (i.e., Bird Eye View) detectors favor sparse convolution (known as SPConv) to speed up training and inference, which puts a hard barrier for deployment especially for on-device applications. In this paper, we tackle the problem of efficient 3D object detection from LiDAR point clouds with deployment in mind. To reduce computational burden, we propose a pillar-based 3D detector with high performance from an industry perspective, termed FastPillars. Compared with previous methods, we introduce a more effective Max-and-Attention pillar encoding (MAPE) module, and redesigning a powerful and lightweight backbone CRVNet imbued with Cross Stage Partial network (CSP) in a reparameterization style, forming a compact feature representation framework. Extensive experiments demonstrate that our FastPillars surpasses the state-of-the-art 3D detectors regarding both on-device speed and performance. Specifically, FastPillars can be effectively deployed through TensorRT, obtaining real-time performance (24FPS) on a single RTX3070Ti GPU with 64.6 mAP on the nuScenes test set. Our code is publicly available at: https://github.com/StiphyJay/FastPillars.
更多查看译文
关键词
3d detector,fastpillars,deployment-friendly,pillar-based
AI 理解论文
溯源树
样例
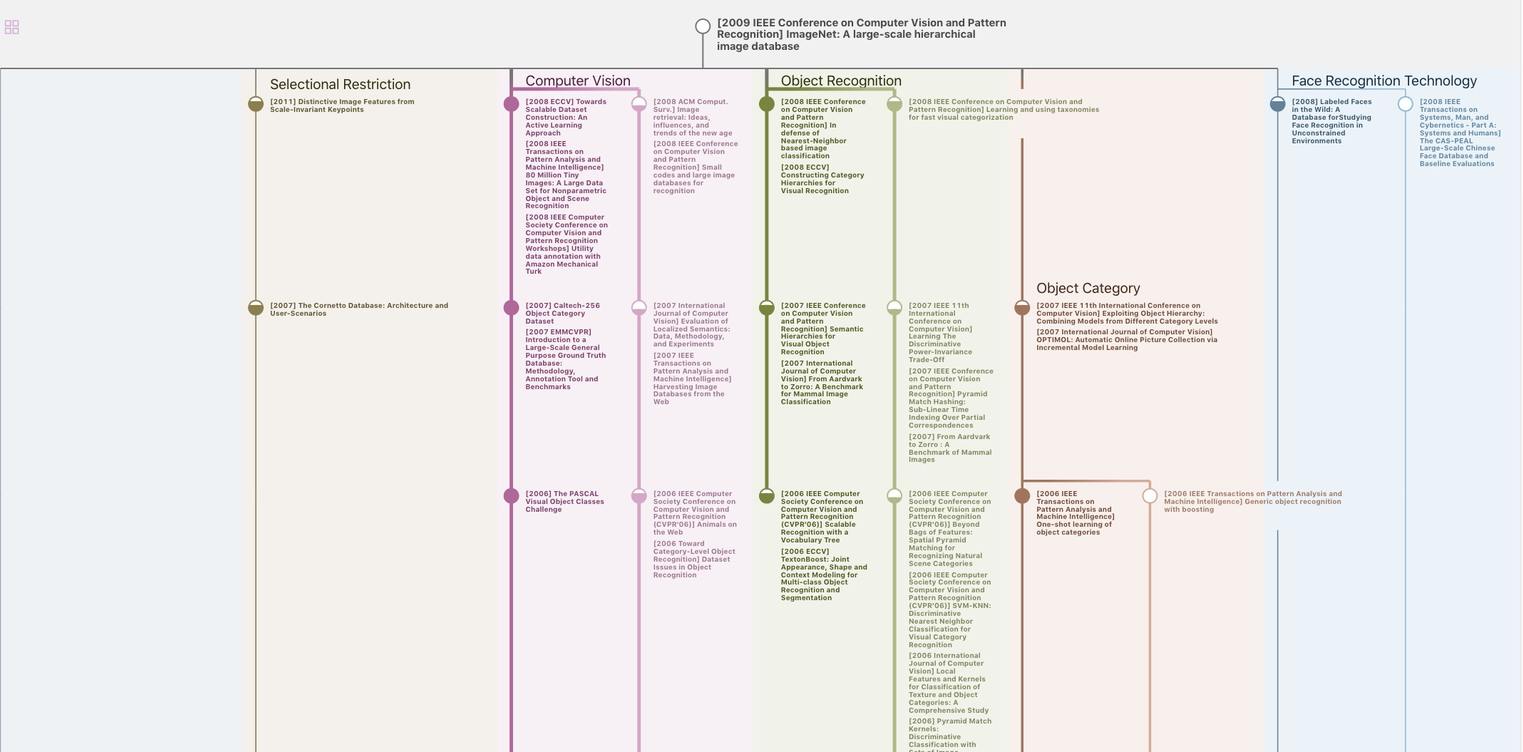
生成溯源树,研究论文发展脉络
Chat Paper
正在生成论文摘要