Linking Datasets on Organizations Using Half A Billion Open Collaborated Records: Perils and Opportunities
CoRR(2020)
摘要
Scholars studying organizations often work with multiple datasets lacking shared unique identifiers or covariates. In such situations, researchers may turn to approximate string matching methods to combine datasets. String matching, although useful, faces fundamental challenges. String distance metrics are not optimized for string matching -- they do not explicitly maximize linkage performance. Moreover, many entities have multiple names that are dissimilar (e.g., "Fannie Mae" and "Federal National Mortgage Association"). This paper introduces data from a prominent employment-related networking site (LinkedIn) as a tool to help to address this problem. We propose interconnected approaches to leveraging the massive amount of information from LinkedIn regarding organizational name-to-name links. The first approach builds a machine learning model for predicting matches from character strings, treating the trillion user-contributed organizational name pairs as a training corpus: this approach constructs a matching metric that explicitly maximizes match probabilities. A second approach extracts plausible name matches using graph theoretic information contained in the LinkedIn data. A third approach combines the machine learning and network methods. We document substantial improvements over fuzzy matching in organization name matching exercises while discussing limitations and ways future methodologists may further make use of this unique data source. We make our methods accessible in an open-source R package available at github.com/cjerzak/LinkOrgs-software.
更多查看译文
AI 理解论文
溯源树
样例
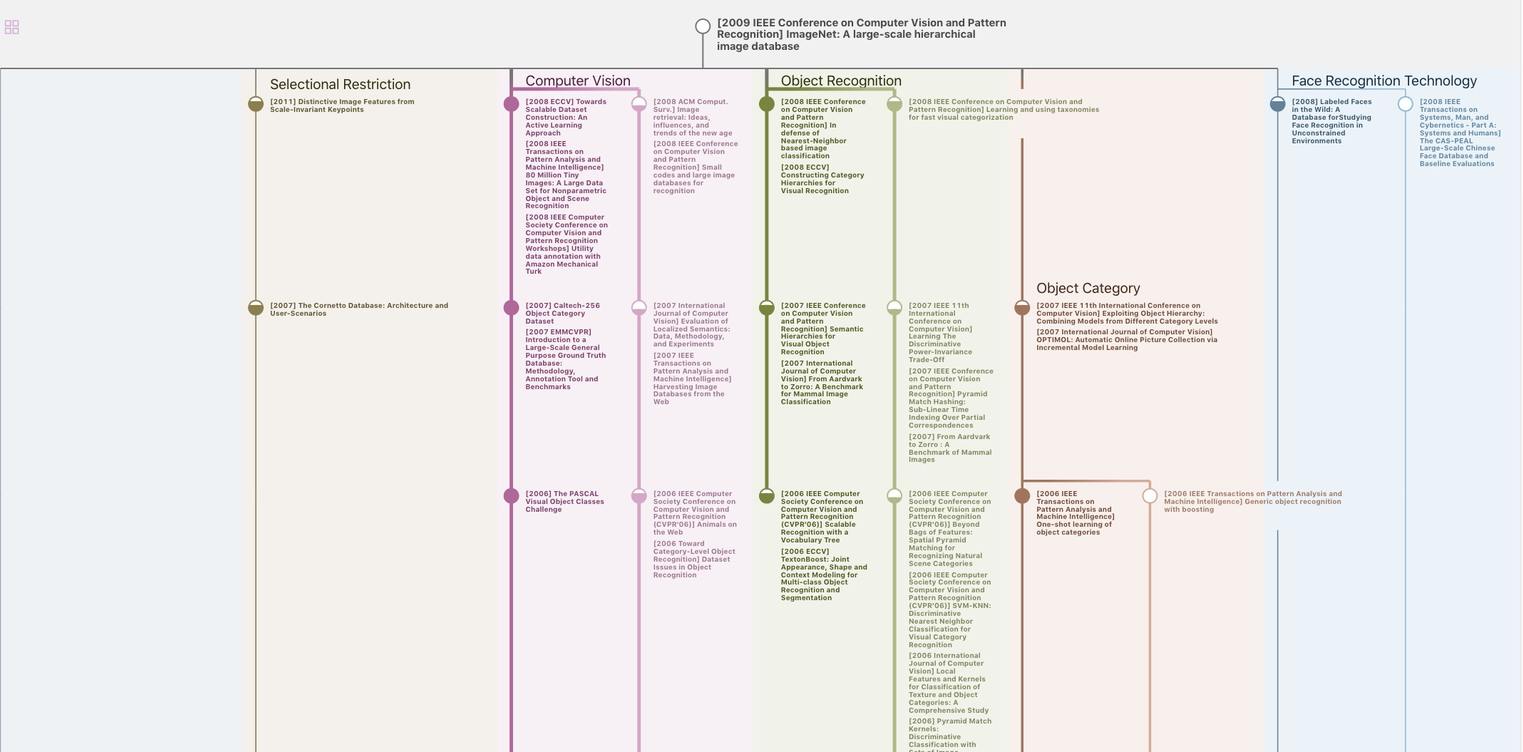
生成溯源树,研究论文发展脉络
Chat Paper
正在生成论文摘要