Hybrid Contrastive Constraints for Multi-Scenario Ad Ranking
PROCEEDINGS OF THE 32ND ACM INTERNATIONAL CONFERENCE ON INFORMATION AND KNOWLEDGE MANAGEMENT, CIKM 2023(2023)
摘要
Multi-scenario ad ranking aims at leveraging the data from multiple domains or channels for training a unified ranking model to improve the performance at each individual scenario. Although the research on this task has made important progress, it still lacks the consideration of cross-scenario relations, thus leading to limitation in learning capability and difficulty in interrelation modeling. In this paper, we propose a Hybrid Contrastive Constrained approach (HC2) for multi-scenario ad ranking. To enhance the modeling of data interrelation, we elaborately design a hybrid contrastive learning approach to capture commonalities and differences among multiple scenarios. The core of our approach consists of two elaborated contrastive losses, namely generalized and individual contrastive loss, which aim at capturing common knowledge and scenario-specific knowledge, respectively. To adapt contrastive learning to the complex multi-scenario setting, we propose a series of important improvements. For generalized contrastive loss, we enhance contrastive learning by extending the contrastive samples (label-aware and diffusion noise enhanced contrastive samples) and reweighting the contrastive samples (reciprocal similarity weighting). For individual contrastive loss, we use the strategies of dropout-based augmentation and cross-scenario encoding for generating meaningful positive and negative contrastive samples, respectively. Extensive experiments on both offline evaluation and online test have demonstrated the effectiveness of the proposed HC2 by comparing it with a number of competitive baselines.
更多查看译文
关键词
Online Advertising,Advertisement Ranking,Multi-Scenario Ad Ranking,Contrastive Learning
AI 理解论文
溯源树
样例
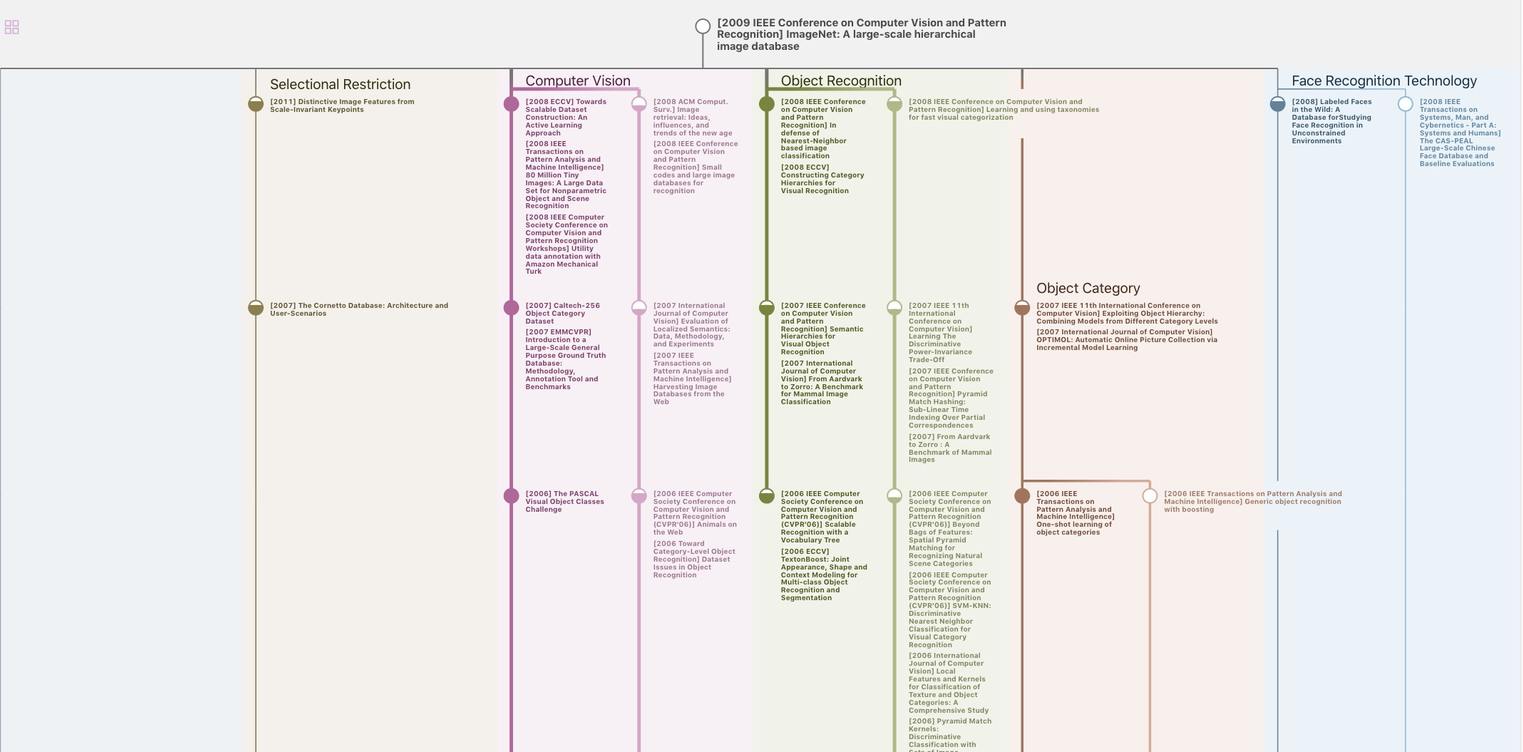
生成溯源树,研究论文发展脉络
Chat Paper
正在生成论文摘要