Dual-Range Context Aggregation for Efficient Semantic Segmentation in Remote Sensing Images
IEEE Geoscience and Remote Sensing Letters(2023)
摘要
Although introducing self-attention mechanisms is beneficial to establish long-range dependencies and explore global context information in the task of remote sensing image semantic segmentation, it results in expensive computation and large memory cost. In this letter, we address this dilemma by proposing a lightweight dual-range context aggregation network (LDCANet) for efficient remote sensing image semantic segmentation. First, a dual-range context aggregation module (DCAM) is designed to aggregate the local features and the global semantic context acquired by convolutions and self-attention, respectively, where self-attention is implemented easily by applying two cascaded linear layers to reduce the computational complexity. Furthermore, a simple and lightweight decoder is employed to combine information from different levels, in which a multilayer perceptron (MLP)-based efficient linear block (ELB) is proposed to yield a strong and efficient representation. Experiments conducted on the International Society for Photogrammetry and Remote Sensing (ISPRS) Vaihingen dataset and the Gaofen Image dataset (GID) prove that our LDCANet achieves an excellent trade-off between segmentation accuracy and computational efficiency. In particular, our method achieves 74.12% mean intersection over union (mIoU) on the ISPRS Vaihingen dataset and 61.42% mIoU on the GID with only 4.98-M parameter size.
更多查看译文
关键词
Convolutional neural networks,lightweight network,multilayer perceptron (MLP),remote sensing,semantic segmentation
AI 理解论文
溯源树
样例
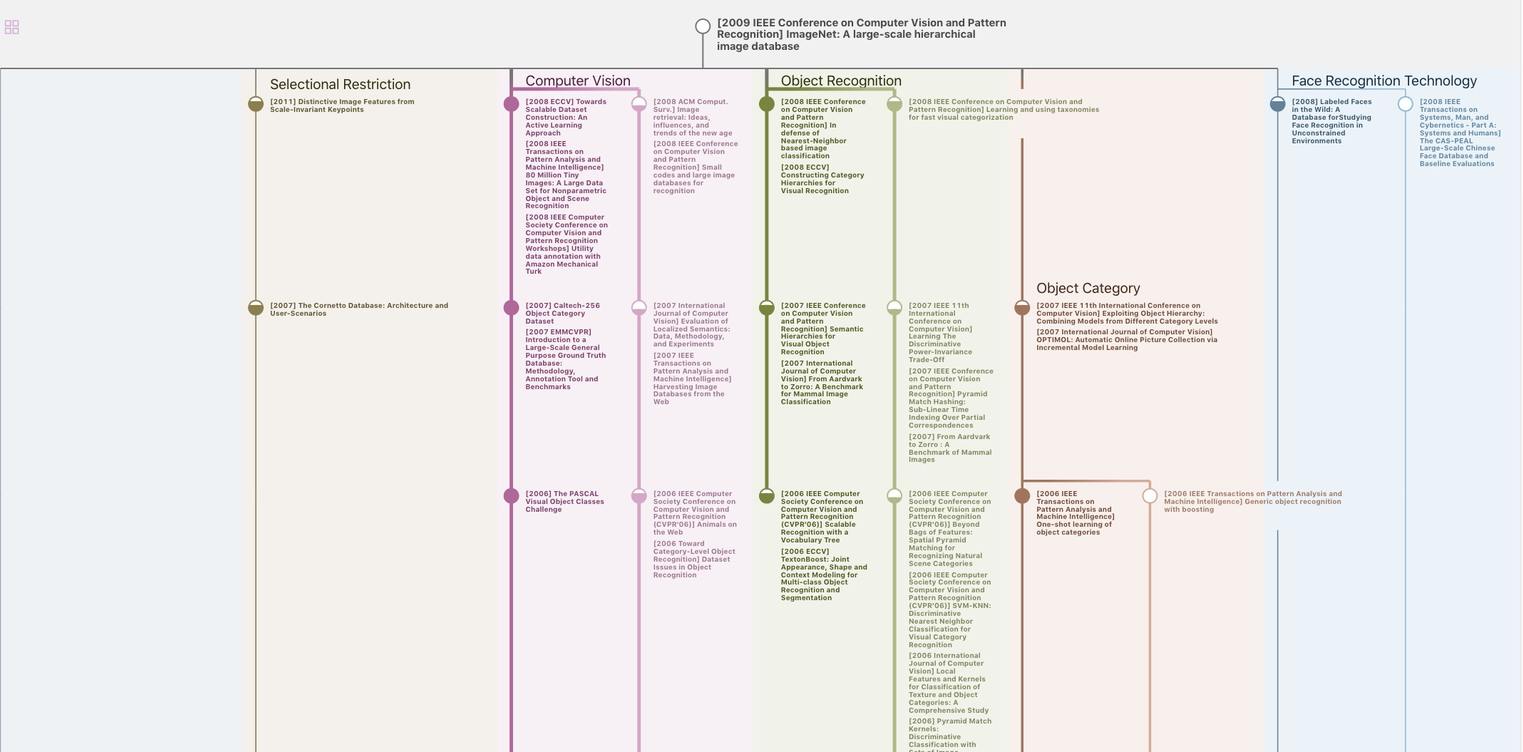
生成溯源树,研究论文发展脉络
Chat Paper
正在生成论文摘要