A Semi-Supervised Method for Multimodal Classification of Consumer Videos
International Journal of Information and Communication Technology Research(2013)
摘要
In large databases, lack of labeled training data leads to major difficulties in classification process. Semi-supervised algorithms are employed to suppress this problem. Video databases are the epitome for such a scenario. Fortunately, graph-based methods have shown to form promising platforms for semi-supervised video classification. Based on multimodal characteristics of video data, different features (SIFT, STIP, and MFCC) have been utilized to build the graph. In this paper, we have proposed a new classification method which fuses the results of manifold regularization over different graphs. Our method acts like a co-training method that tries to find the correct label for unlabeled data during its iterations. But, unlike other co-training methods, it takes into account the unlabeled data in the classification process. After manifold regularization, data fusion is doneby a ranking method which improves the algorithm to become competitive with supervised methods. Our experimental results, run on the CCV database, show the efficiency of the proposed classification method.
更多查看译文
关键词
semi-supervised learning,co-training,video classification,multimodal features
AI 理解论文
溯源树
样例
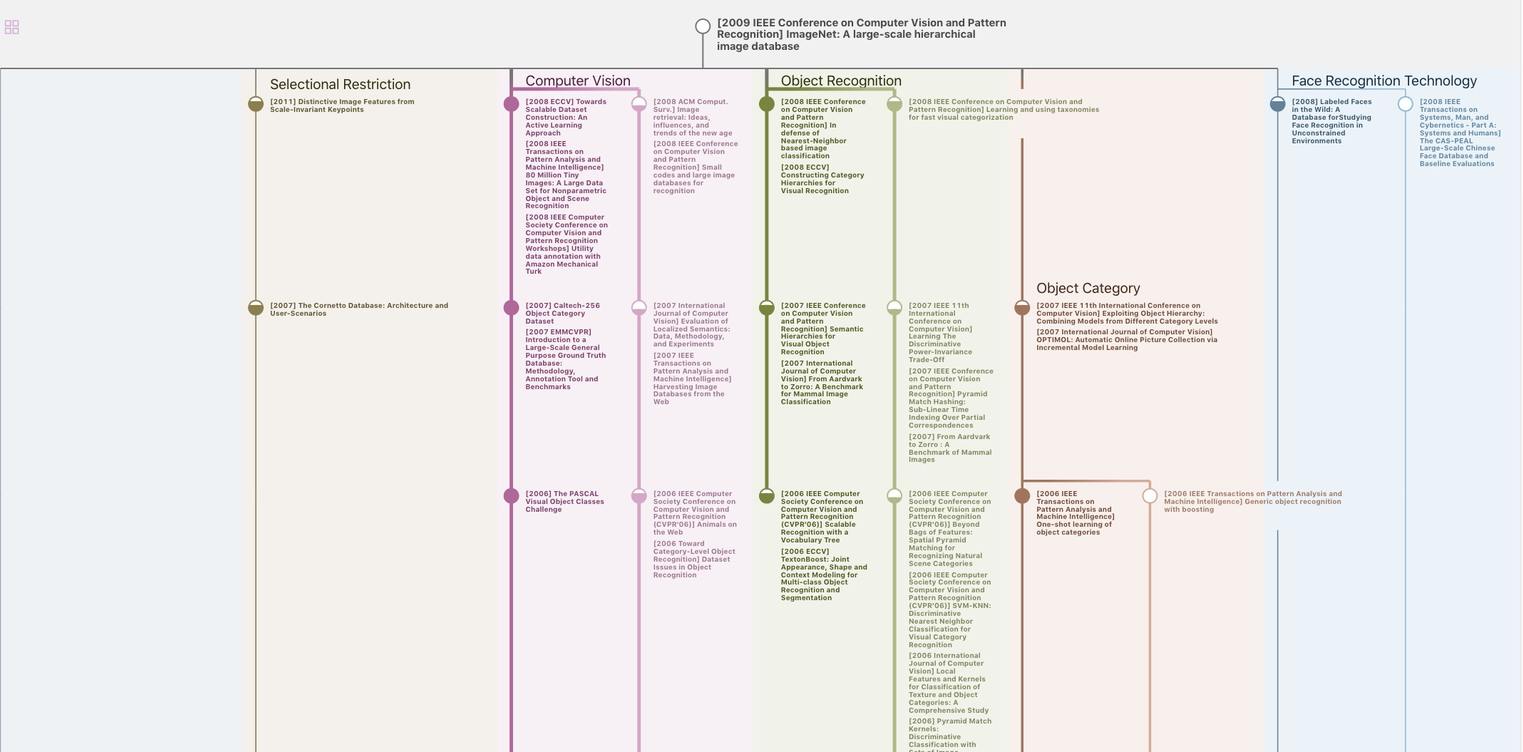
生成溯源树,研究论文发展脉络
Chat Paper
正在生成论文摘要