EEG-Based Emotion Recognition for Hearing Impaired and Normal Individuals With Residual Feature Pyramids Network Based on Time–Frequency–Spatial Features
IEEE Transactions on Instrumentation and Measurement(2023)
摘要
With the development of affective computing, discriminative feature selection is critical for electroencephalography (EEG) emotion recognition. In this article, we fused four EEG feature matrices constructed by the preprocessed signal, the differential entropy (DE), the symmetric difference, and the symmetric quotient based on the International 10–20 system, which integrates time-, frequency-, and spatial-domain information of EEG signals. For the feature classification model, we used the space-to-depth (S2D) layer instead of the convolutional neural network (CNN) as the backbone to reduce the calculation of the model without affecting the classification performance. The residual feature pyramid network (RFPN) was proposed to obtain the correlation of channels, and then, the deep multiscale semantic information of EEG feature maps is captured. The emotion classification strategy was evaluated by DEAP, SEED, SEED-IV, and our hearing-impaired EEG dataset (HIED). The classification accuracies were 93.56% (four-class, DEAP), 96.84% (three-class, SEED), 91.62% (four-class, SEED-IV), and 87.74% (six-class, HIED). Furthermore, we also found that the difference in emotional response between the left and right brain regions of hearing-impaired subjects is more obvious than that of normal subjects.
更多查看译文
关键词
Electroencephalography (EEG),emotion recognition,hearing-impaired subjects,residual feature pyramid network (RFPN),space-to-depth (S2D) layer
AI 理解论文
溯源树
样例
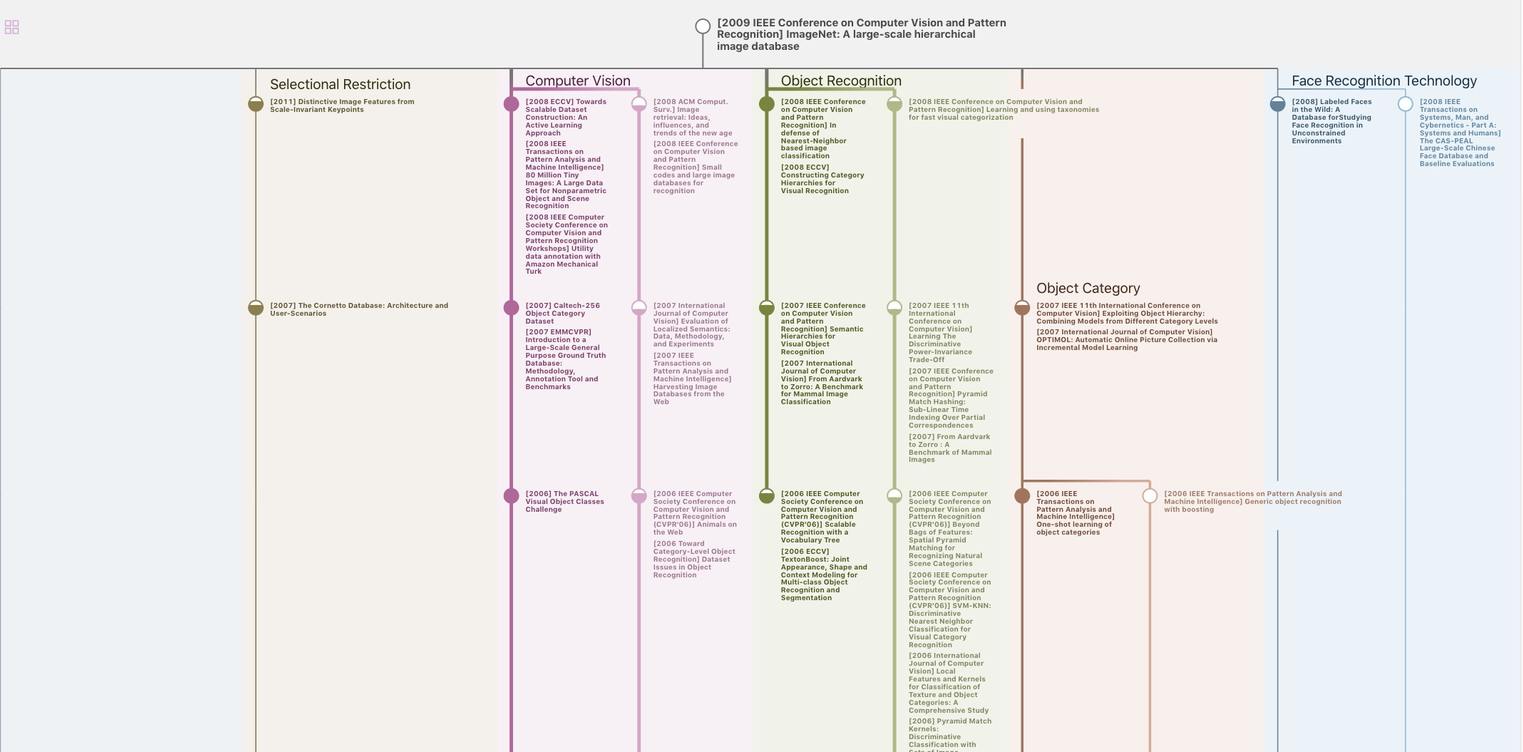
生成溯源树,研究论文发展脉络
Chat Paper
正在生成论文摘要