Estimating Longitudinal Causal Effects with Unobserved Noncompliance Using a Semi-Parametric G-computation Algorithm
arxiv(2023)
摘要
Participant noncompliance, in which participants do not follow their assigned treatment protocol, often obscures the causal relationship between treatment and treatment effect in randomized trials. In the longitudinal setting, the G-computation algorithm can adjust for confounding to estimate causal effects. Typically, G-computation assumes that both 1) compliance is observed; and 2) the densities of the confounders can be correctly specified. We aim to develop a G-computation estimator in the setting where both assumptions are violated. For 1), in place of unobserved compliance, we substitute in probability weights derived from modeling a biomarker associated with compliance. For 2), we fit semiparametric models using predictive mean matching. Specifically, we parametrically specify only the conditional mean of the confounders, and then use predictive mean matching to randomly generate confounder data for G-computation. In both the simulation and application, we compare multiple causal estimators already established in the literature with those derived from our method. For the simulation, we generated data across different sample sizes and levels of confounding. For the application, we apply our method to a trial that sought to evaluate the effect of cigarettes with low nicotine on cigarette consumption (Center for the Evaluation of Nicotine in Cigarettes Project 2 - CENIC-P2).
更多查看译文
关键词
longitudinal causal effects,unobserved noncompliance
AI 理解论文
溯源树
样例
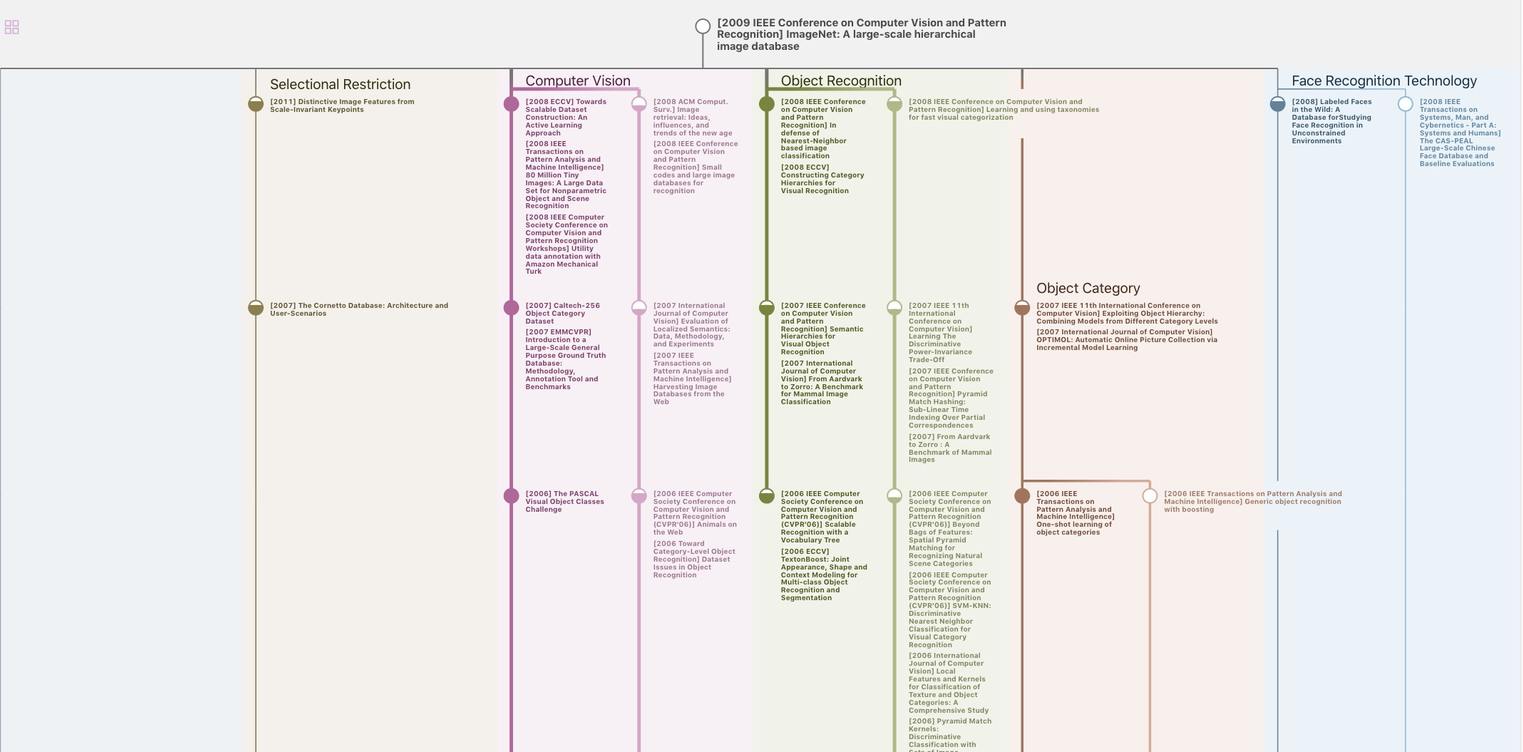
生成溯源树,研究论文发展脉络
Chat Paper
正在生成论文摘要