Comparison of behavioral systems theory and conventional linear models for predicting building zone temperature in long-term in situ measurements
arxiv(2023)
摘要
The potential of Model Predictive Control in buildings has been shown many times, being successfully used to achieve various goals, such as minimizing energy consumption or maximizing thermal comfort. However, mass deployment has thus far failed, in part because of the high engineering cost of obtaining and maintaining a sufficiently accurate model. This can be addressed by using adaptive data-driven approaches. The idea of using behavioral systems theory for this purpose has recently found traction in the academic community. In this study, we compare variations thereof with different amounts of data used, different regularization weights, and different methods of data selection. Autoregressive models with exogenous inputs (ARX) are used as a well-established reference. All methods are evaluated by performing iterative system identification on two long-term data sets from real occupied buildings, neither of which include artificial excitation for the purpose of system identification. We find that: (1) Sufficient prediction accuracy is achieved with all methods. (2) The ARX models perform slightly better, while having the additional advantages of fewer tuning parameters and faster computation. (3) Adaptive and non-adaptive schemes perform similarly. (4) The regularization weights of the behavioral systems theory methods show the expected trade-off characteristic with an optimal middle value. (5) Using the most recent data yields better performance than selecting data with similar weather as the day to be predicted. (6) More data improves the model performance.
更多查看译文
关键词
zone temperature,behavioral systems theory,building,conventional linear models
AI 理解论文
溯源树
样例
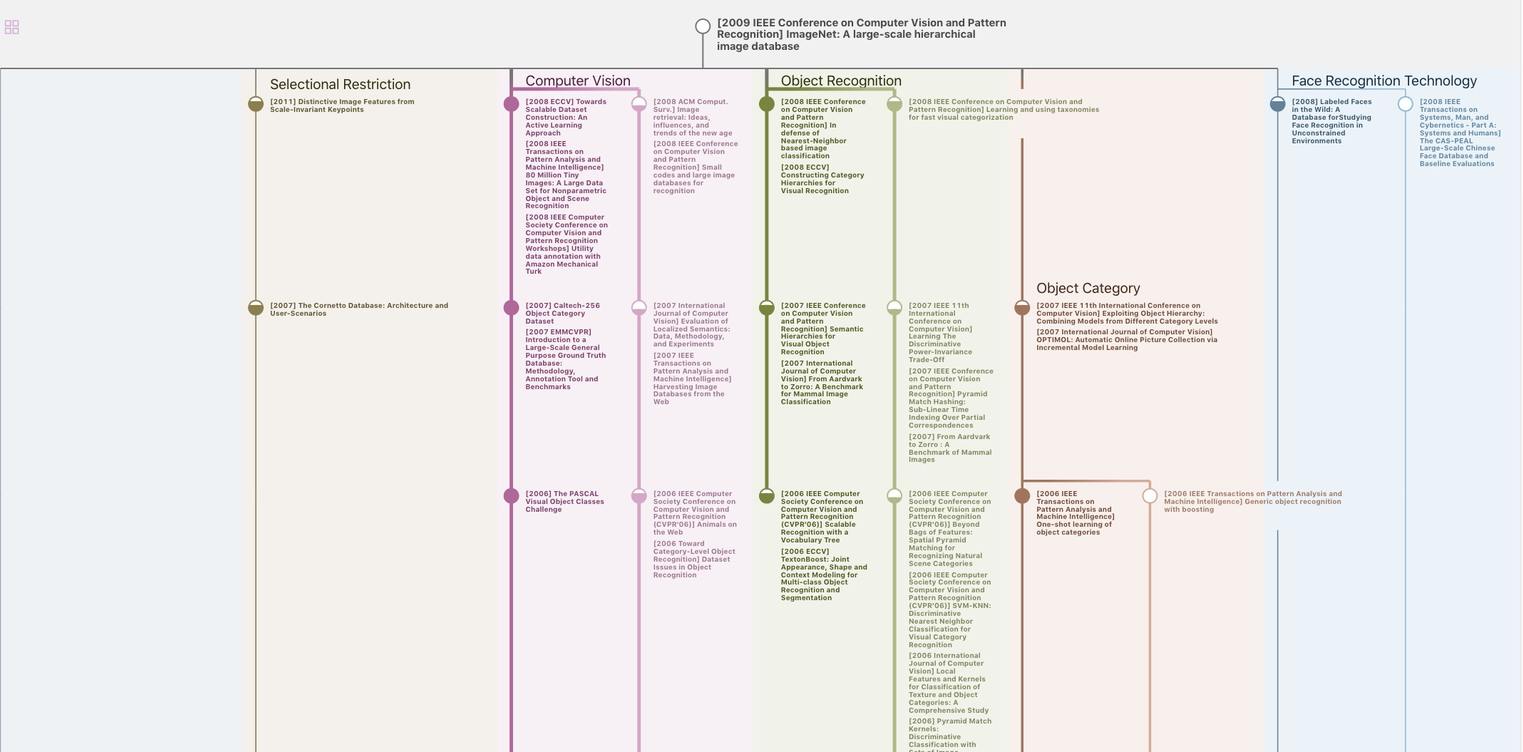
生成溯源树,研究论文发展脉络
Chat Paper
正在生成论文摘要