Predicting the performance of hybrid ventilation in buildings using a multivariate attention-based biLSTM Encoder-Decoder neural network
arxiv(2023)
摘要
Hybrid ventilation is an energy-efficient solution to provide fresh air for most climates, given that it has a reliable control system. To operate such systems optimally, a high-fidelity control-oriented modesl is required. It should enable near-real time forecast of the indoor air temperature based on operational conditions such as window opening and HVAC operating schedules. However, physics-based control-oriented models (i.e., white-box models) are labour-intensive and computationally expensive. Alternatively, black-box models based on artificial neural networks can be trained to be good estimators for building dynamics. This paper investigates the capabilities of a deep neural network (DNN), which is a multivariate multi-head attention-based long short-term memory (LSTM) encoder-decoder neural network, to predict indoor air temperature when windows are opened or closed. Training and test data are generated from a detailed multi-zone office building model (EnergyPlus). Pseudo-random signals are used for the indoor air temperature setpoints and window opening instances. The results indicate that the DNN is able to accurately predict the indoor air temperature of five zones whenever windows are opened or closed. The prediction error plateaus after the 24th step ahead prediction (6 hr ahead prediction).
更多查看译文
关键词
hybrid ventilation,buildings,attention-based,encoder-decoder
AI 理解论文
溯源树
样例
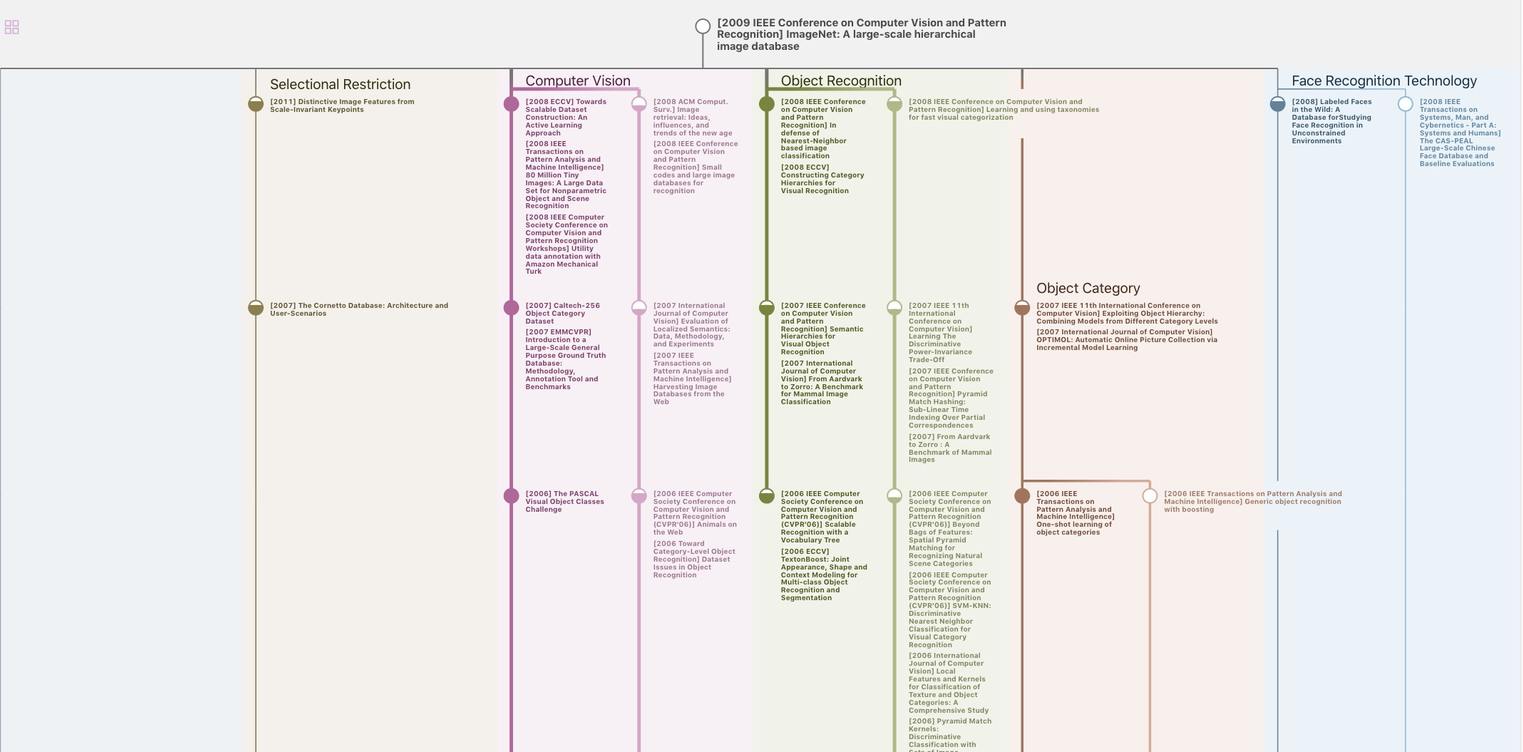
生成溯源树,研究论文发展脉络
Chat Paper
正在生成论文摘要