Learning How to Infer Partial MDPs for In-Context Adaptation and Exploration
arXiv (Cornell University)(2023)
Abstract
To generalize across tasks, an agent should acquire knowledge from past tasks that facilitate adaptation and exploration in future tasks. We focus on the problem of in-context adaptation and exploration, where an agent only relies on context, i.e., history of states, actions and/or rewards, rather than gradient-based updates. Posterior sampling (extension of Thompson sampling) is a promising approach, but it requires Bayesian inference and dynamic programming, which often involve unknowns (e.g., a prior) and costly computations. To address these difficulties, we use a transformer to learn an inference process from training tasks and consider a hypothesis space of partial models, represented as small Markov decision processes that are cheap for dynamic programming. In our version of the Symbolic Alchemy benchmark, our method's adaptation speed and exploration-exploitation balance approach those of an exact posterior sampling oracle. We also show that even though partial models exclude relevant information from the environment, they can nevertheless lead to good policies.
MoreTranslated text
Key words
partial mdps,exploration,adaptation,learning,in-context
AI Read Science
Must-Reading Tree
Example
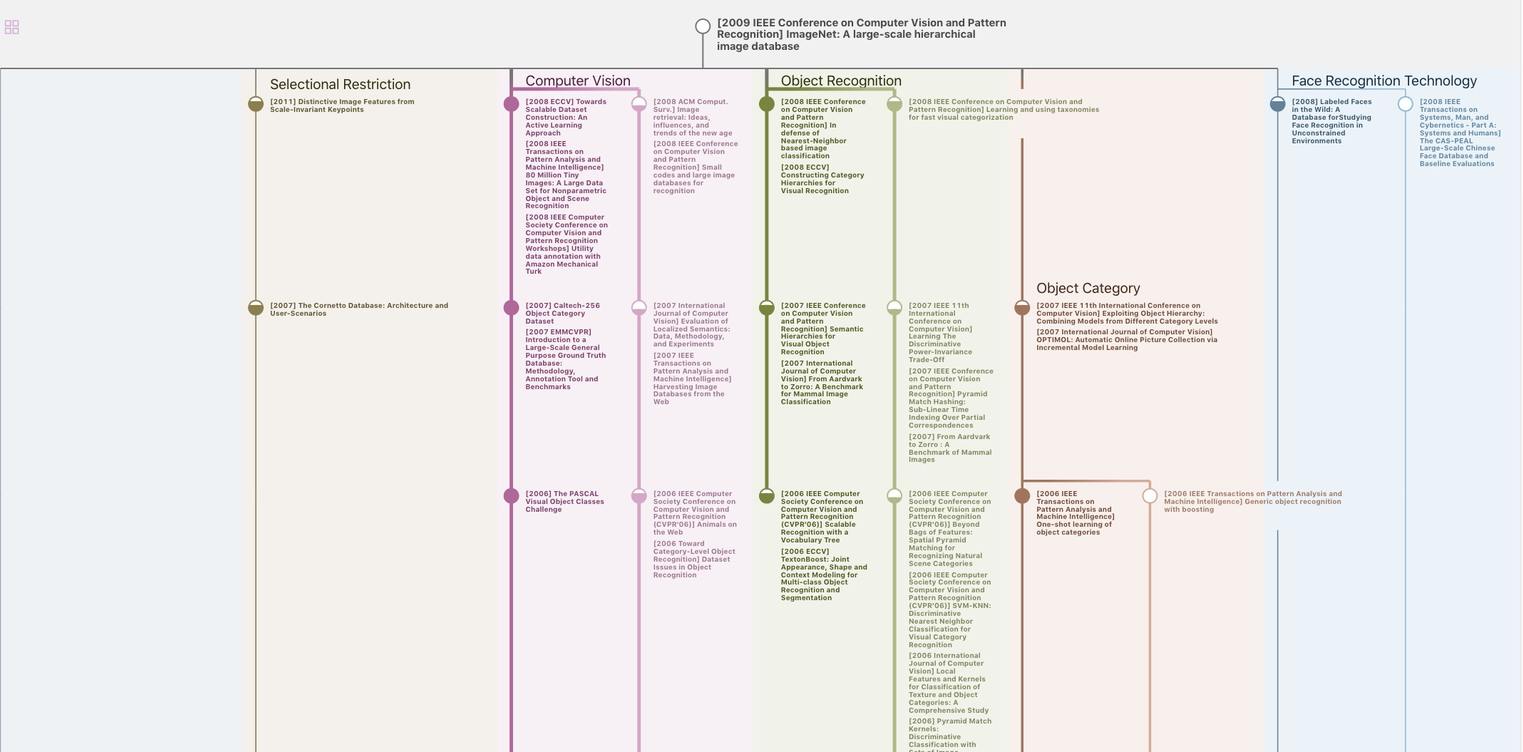
Generate MRT to find the research sequence of this paper
Chat Paper
Summary is being generated by the instructions you defined