Surrogate modeling of subgrid-scale effects in idealized atmospheric flows: A deep learned approach using high-resolution simulation data
Authorea (Authorea)(2023)
摘要
We introduce a machine learned surrogate model from high-resolution simulation data to capture the 1 subgrid-scale effects in dry, stratified atmospheric flows. We use deep neural networks (NNs) to model 2 the state differences between a coarse resolution simulation and a high-resolution simulation, both 3 run simultaneously with the coarse resolution simulation forced by the high-resolution simulation. 4 The setup enables the capture of both dissipative and anti-dissipative effects in the state differences. 5 The NN model is able to accurately capture the state differences in offline tests outside the training 6 regime. In online tests intended for production use, the NN coupled coarse simulation has higher 7 accuracy over a significant period of time compared to the coarse-resolution simulation without any 8 correction. We provide evidence to the capability of the NN model to accurately capture high gradient 9 regions in the flow field. With the accumulation of the errors, the NN-coupled simulation becomes 10 computationally unstable after approximately 85 coarse simulation time steps. Insights gained from 11 these surrogate models further pave the way for formulating stable, complex, physics-based NN 12 models which are driven by traditional subgrid-scale turbulence closure models.
更多查看译文
关键词
atmospheric flows,surrogate modeling,simulation,subgrid-scale,high-resolution
AI 理解论文
溯源树
样例
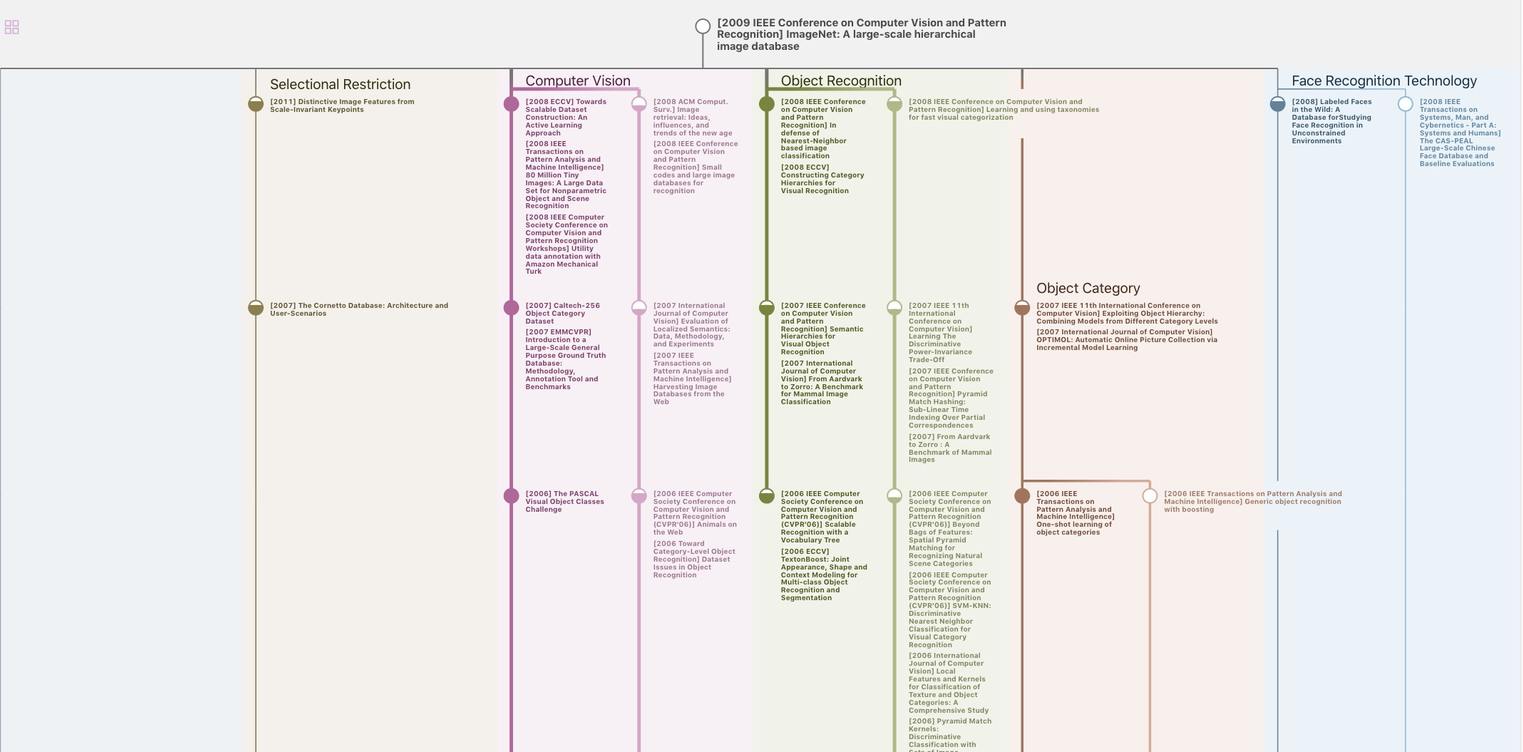
生成溯源树,研究论文发展脉络
Chat Paper
正在生成论文摘要