Deep learning model integrating positron emission tomography and clinical data for prognosis prediction in non-small cell lung cancer patients
BMC Bioinform.(2023)
摘要
Background Lung cancer is the leading cause of cancer-related deaths worldwide. The majority of lung cancers are non-small cell lung cancer (NSCLC), accounting for approximately 85% of all lung cancer types. The Cox proportional hazards model (CPH), which is the standard method for survival analysis, has several limitations. The purpose of our study was to improve survival prediction in patients with NSCLC by incorporating prognostic information from F-18 fluorodeoxyglucose positron emission tomography (FDG PET) images into a traditional survival prediction model using clinical data. Results The multimodal deep learning model showed the best performance, with a C-index and mean absolute error of 0.756 and 399 days under a five-fold cross-validation, respectively, followed by ResNet3D for PET (0.749 and 405 days) and CPH for clinical data (0.747 and 583 days). Conclusion The proposed deep learning-based integrative model combining the two modalities improved the survival prediction in patients with NSCLC.
更多查看译文
关键词
Deep learning,FDG PET,Lung cancer,Multimodal learning,Survival prediction
AI 理解论文
溯源树
样例
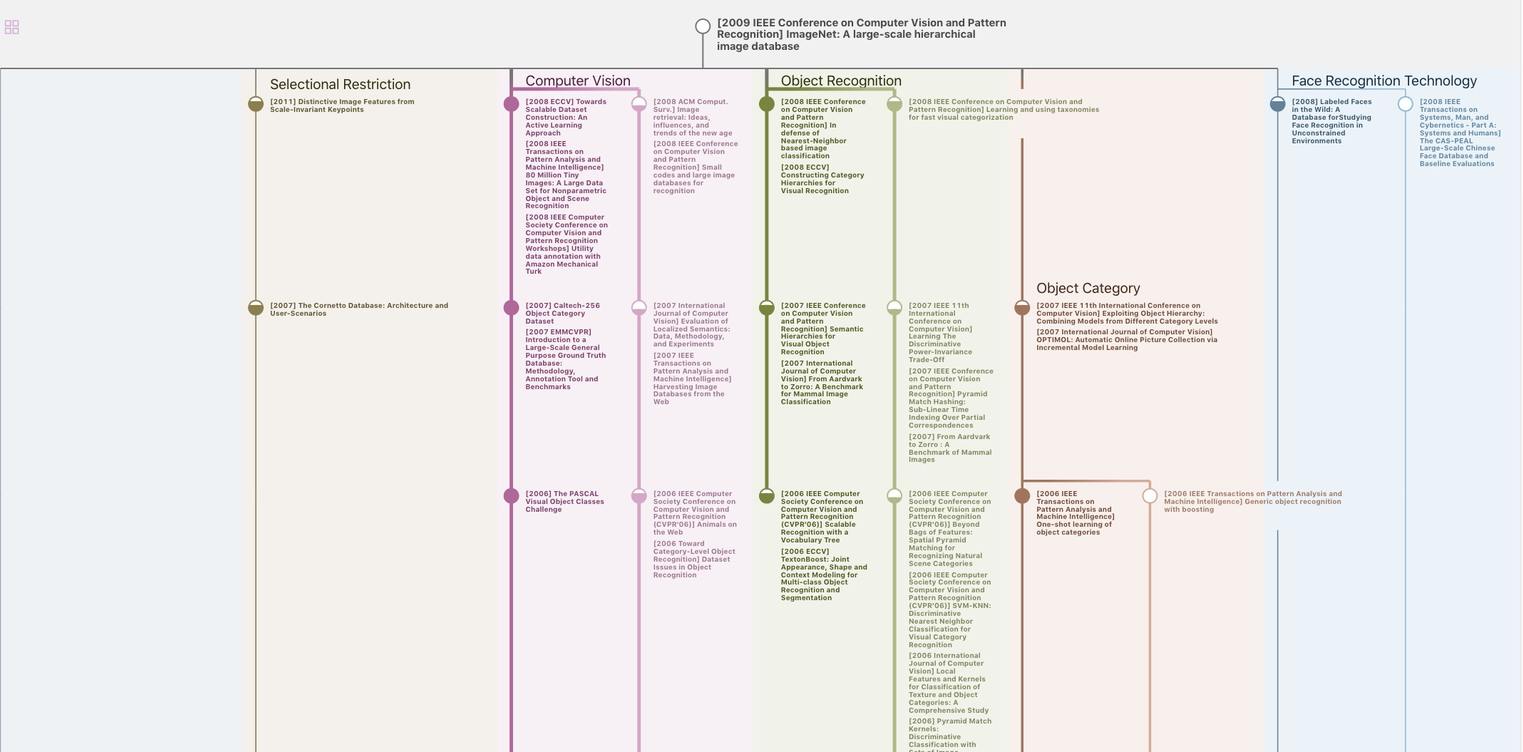
生成溯源树,研究论文发展脉络
Chat Paper
正在生成论文摘要