Probabilistic cell/domain-type assignment of spatial transcriptomics data with SpatialAnno
Nucleic acids research(2023)
摘要
In the analysis of both single-cell RNA sequencing (scRNA-seq) and spatially resolved transcriptomics (SRT) data, classifying cells/spots into cell/domain types is an essential analytic step for many secondary analyses. Most of the existing annotation methods have been developed for scRNA-seq datasets without any consideration of spatial information. Here, we present SpatialAnno, an efficient and accurate annotation method for spatial transcriptomics datasets, with the capability to effectively leverage a large number of non-marker genes as well as “qualitative” information about marker genes without using a reference dataset. Uniquely, SpatialAnno estimates low-dimensional embeddings for a large number of non-marker genes via a factor model while promoting spatial smoothness among neighboring spots via a Potts model. Using both simulated and four real spatial transcriptomics datasets from the 10x Visium, ST, Slide-seqV1/2, and seqFISH platforms, we showcase the method’s improved spatial annotation accuracy, including its robustness to the inclusion of marker genes for irrelevant cell/domain types and to various degrees of marker gene misspecification. SpatialAnno is computationally scalable and applicable to SRT datasets from different platforms. Furthermore, the estimated embeddings for cellular biological effects facilitate many downstream analyses.
### Competing Interest Statement
The authors have declared no competing interest.
更多查看译文
关键词
spatial transcriptomics data,probabilistic cell/domain-type
AI 理解论文
溯源树
样例
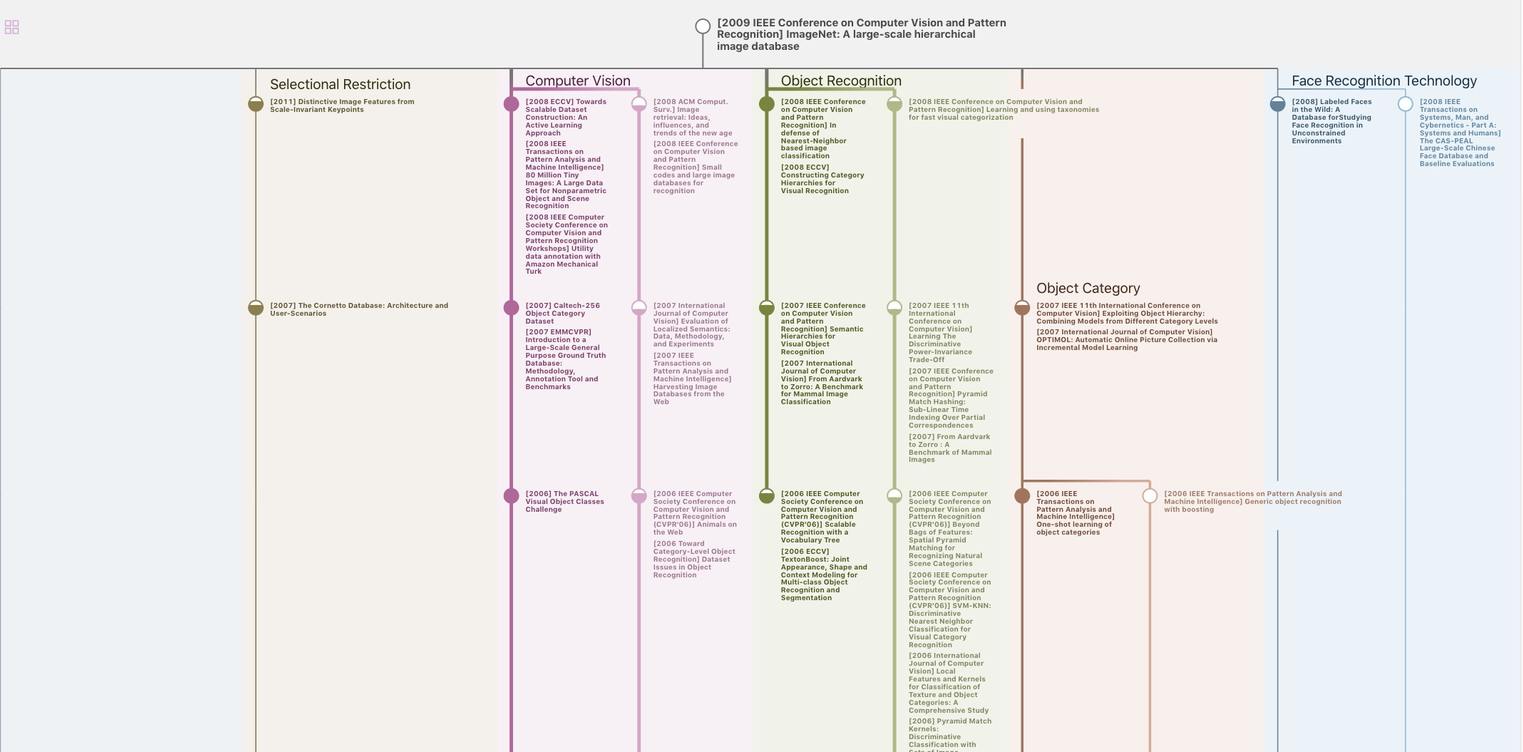
生成溯源树,研究论文发展脉络
Chat Paper
正在生成论文摘要