Model Personalization with Static and Dynamic Patients' Data
2022 IEEE International Conference on Data Mining Workshops (ICDMW)(2022)
摘要
Recent progress in machine learning has been ac-companied by the availability of large amount of data in many do-mains. The clinical domain is no exception, comprehensive patient information covering their whole treatment journeys is stored in Electronic Health Records (EHRs). Still, there is a strong heterogeneity among patients that is difficult to capture using standard supervised machine learning tools. Few-shot learning methods, developed for limited data regimes, are foremost trained to quickly adapt to new tasks. In the clinical setting a task is a patient, adapting to the task encompasses optimizing predictions for the patient at hand, and thus personalizing predictions. In this work, we utilize MAML, a meta-learning approach for few-shot learning, for personalizing models in a challenging setting. We assume patients' data to comprise a static part and a dynamic part. Our goal is to investigate how well models trained to quickly adapt to specific patients perform compared to models trained in a conventional manner in this setting. Additionally, we study how static patients' data can be leveraged to acquire personalized models early on, and how these models adapt as more dynamic data are available. Our experiments show that models learned by MAML do indeed make better predictions when adapted to specific patients, and that leveraging static attributes for personalization does lead to an improved performance at best and does not hurt performance at worst. Our findings suggest that few-shot learning has a promising potential in challenging clinical settings, where only limited information are available about patients and where personalized medicine is beneficial.
更多查看译文
关键词
few-shot learning,representation learning
AI 理解论文
溯源树
样例
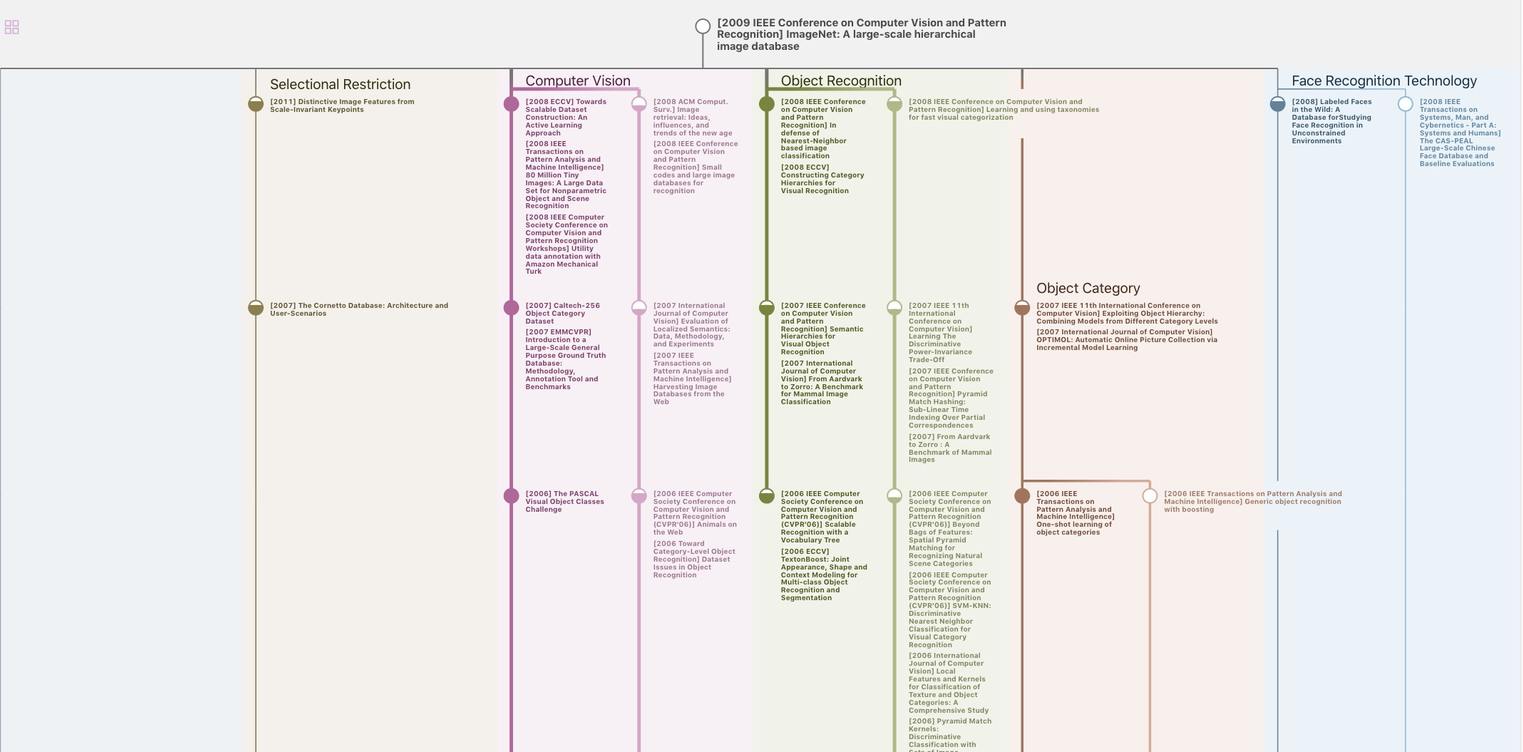
生成溯源树,研究论文发展脉络
Chat Paper
正在生成论文摘要