Domain Generalization by Functional Regression
NUMERICAL FUNCTIONAL ANALYSIS AND OPTIMIZATION(2024)
摘要
The problem of domain generalization is to learn, given data from different source distributions, a model that can be expected to generalize well on new target distributions which are only seen through unlabeled samples. In this paper, we study domain generalization as a problem of functional regression. Our concept leads to a new algorithm for learning a linear operator from marginal distributions of inputs to the corresponding conditional distributions of outputs given inputs. Our algorithm allows a source distribution-dependent construction of reproducing kernel Hilbert spaces for prediction, and, satisfies finite sample error bounds for the idealized risk. Numerical implementations and source code are available1.
更多查看译文
关键词
Domain generalization,finite sample bounds,function-to-function regression,operator learning,reproducing kernel Hilbert space
AI 理解论文
溯源树
样例
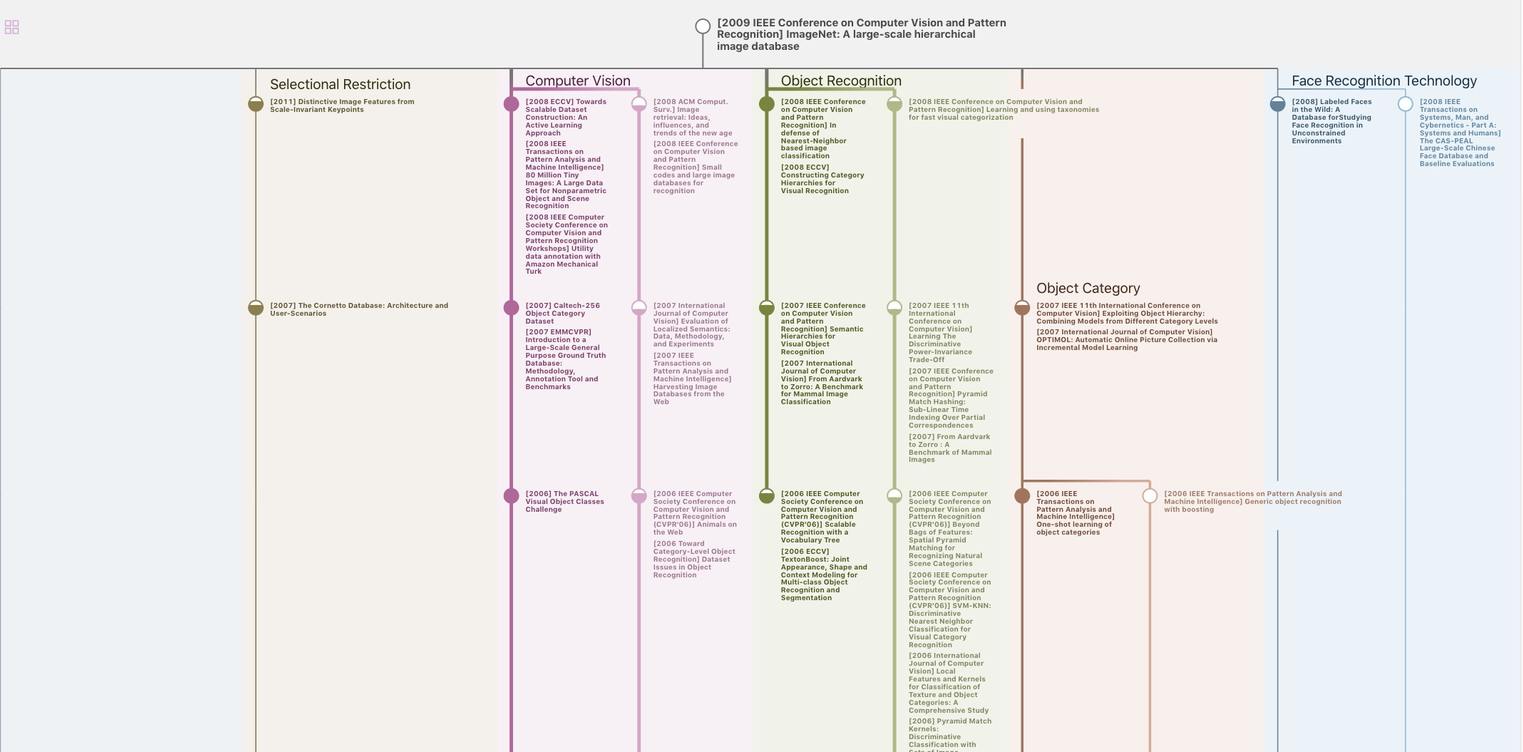
生成溯源树,研究论文发展脉络
Chat Paper
正在生成论文摘要