Explainable Artificial Intelligence Applied to Deep Reinforcement Learning Controllers for Photovoltaic Maximum Power Point Tracking
2022 International Conference on Future Trends in Smart Communities (ICFTSC)(2022)
摘要
Deep Reinforcement Learning (DRL) algorithms have been applied to extract maximum power from photovoltaic (PV) modules under a variety of environmental conditions. However, it is difficult for a human to explain how a DRL-based maximum power point tracking (MPPT) controller works as it consists of Neural Networks (NNs) that are generally complex and non-linear. Various Explainable Artificial Intelligence (XAI) techniques have been proposed to interpret NNs in power system applications, but MPPT controllers have yet to be analyzed. This paper presents the application of XAI techniques to the DRL agents for MPPT. Two distinct DRL agents were developed, one with and one without the information of the converter's duty cycle, using the Deep Deterministic Policy Gradient (DDPG) algorithm and analyzed using XAI techniques, namely Local Interpretable Model-agnostic Explanations (LIME) and Shapley Additive Explanations (SHAP). The results reveal that the converter's input power is the most crucial information for the DRL agents when the converter is operating away from the maximum power point. When the converter approaches operation at the maximum power point, the DRL agents are significantly dependent on the power differential of the converter across time. If the information about the converter's duty cycle is available, the DRL agents are significantly reliant on the converter's duty cycle and disregard other observations for decision-making.
更多查看译文
关键词
Explainable Artificial Intelligence,Interpretable Machine Learning,Deep Reinforcement Learning,Maximum Power Point Tracking,Photovoltaic Systems
AI 理解论文
溯源树
样例
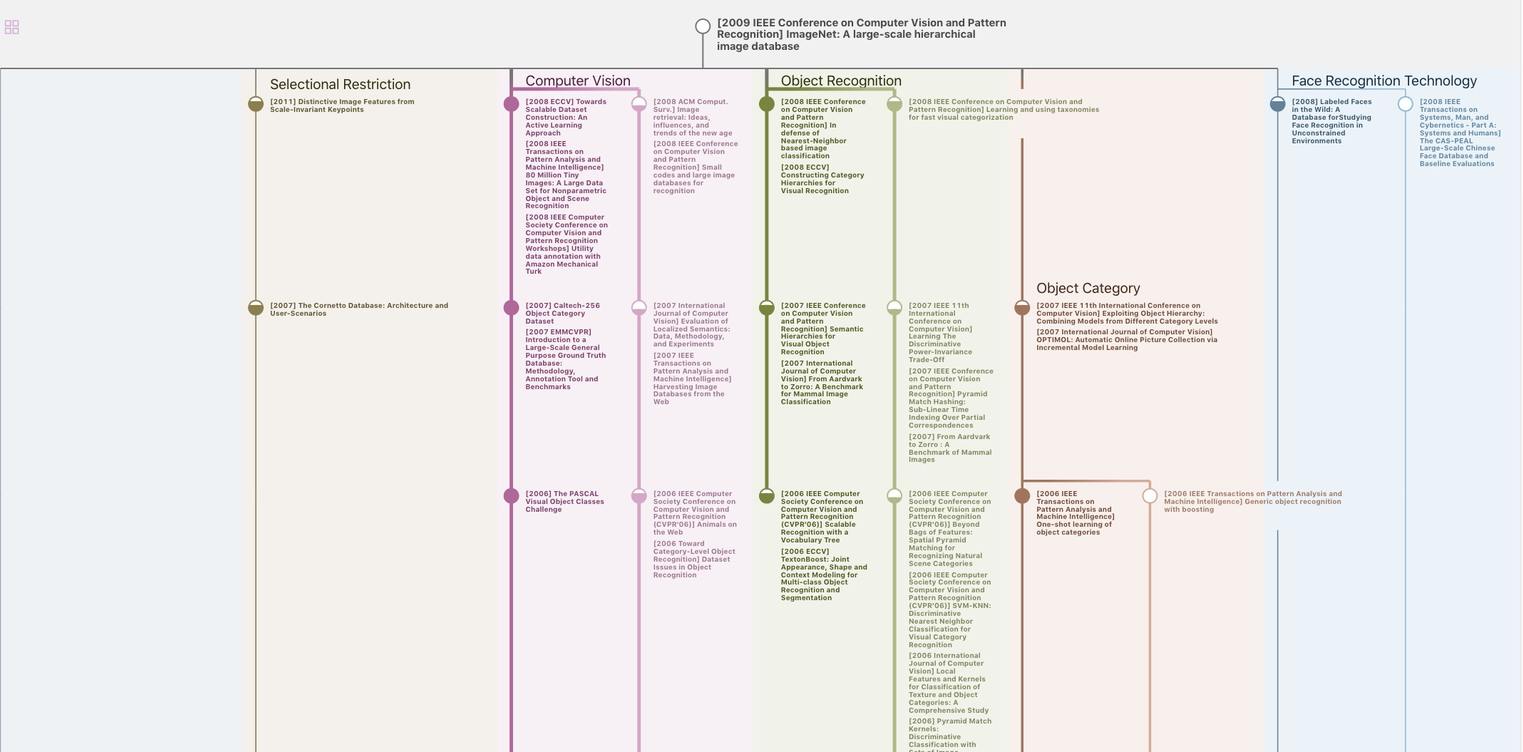
生成溯源树,研究论文发展脉络
Chat Paper
正在生成论文摘要