Discovery of new STAT3 inhibitors as anticancer agents using ligand-receptor contact fingerprints and docking-augmented machine learning.
RSC advances(2023)
摘要
STAT3 belongs to a family of seven vital transcription factors. High levels of STAT3 are detected in several types of cancer. Hence, STAT3 inhibition is considered a promising therapeutic anti-cancer strategy. In this work, we used multiple docked poses of STAT3 inhibitors to augment training data for machine learning QSAR modeling. Ligand-Receptor Contact Fingerprints and scoring values were implemented as descriptor variables. Escalating docking-scoring consensus levels were scanned against orthogonal machine learners, and the best learners (Random Forests and XGBoost) were coupled with genetic algorithm and Shapley additive explanations (SHAP) to identify critical descriptors that determine anti-STAT3 bioactivity to be translated into pharmacophore model(s). Two successful pharmacophores were deduced and subsequently used for screening against the National Cancer Institute (NCI) database. A total of 26 hits were evaluated for their anti-STAT3 bioactivities. Out of which, three hits of novel chemotypes, showed cytotoxic IC values in the nanomolar range (35 nM to 6.7 μM). However, two are potent dihydrofolate reductase (DHFR) inhibitors and therefore should have significant indirect STAT3 inhibitory effects. The third hit (cytotoxic IC = 0.44 μM) is purely direct STAT3 inhibitor (devoid of DHFR activity) and caused, at its cytotoxic IC, more than two-fold reduction in the expression of STAT3 downstream genes (c-Myc and Bcl-xL). The presented work indicates that the concept of data augmentation using multiple docked poses is a promising strategy for generating valid machine learning models capable of discriminating active from inactive compounds.
更多查看译文
关键词
new stat3 inhibitors,anticancer agents,ligand-receptor,docking-augmented
AI 理解论文
溯源树
样例
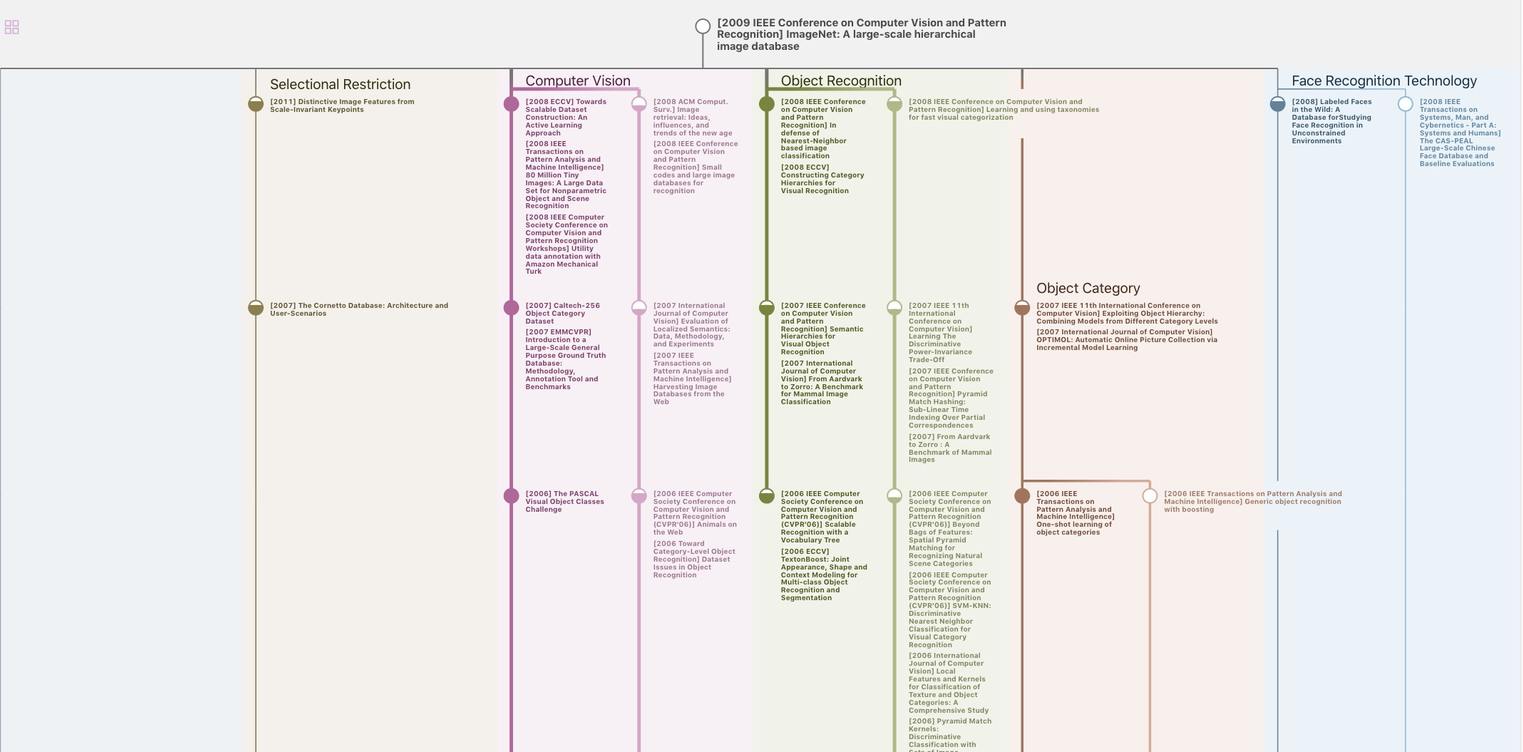
生成溯源树,研究论文发展脉络
Chat Paper
正在生成论文摘要