Hypernetworks Build Implicit Neural Representations of Sounds
MACHINE LEARNING AND KNOWLEDGE DISCOVERY IN DATABASES: RESEARCH TRACK, ECML PKDD 2023, PT IV(2023)
摘要
Implicit Neural Representations (INRs) are nowadays used to represent multimedia signals across various real-life applications, including image super-resolution, image compression, or 3D rendering. Existing methods that leverage INRs are predominantly focused on visual data, as their application to other modalities, such as audio, is nontrivial due to the inductive biases present in architectural attributes of image-based INR models. To address this limitation, we introduce HyperSound, the first meta-learning approach to produce INRs for audio samples that leverages hypernetworks to generalize beyond samples observed in training. Our approach reconstructs audio samples with quality comparable to other state-of-the-art models and provides a viable alternative to contemporary sound representations used in deep neural networks for audio processing, such as spectrograms. Our code is publicly available at https://github.com/WUT-AI/hypersound.
更多查看译文
关键词
Machine Learning,Hypernetworks,Audio Processing,Implicit Neural Representations
AI 理解论文
溯源树
样例
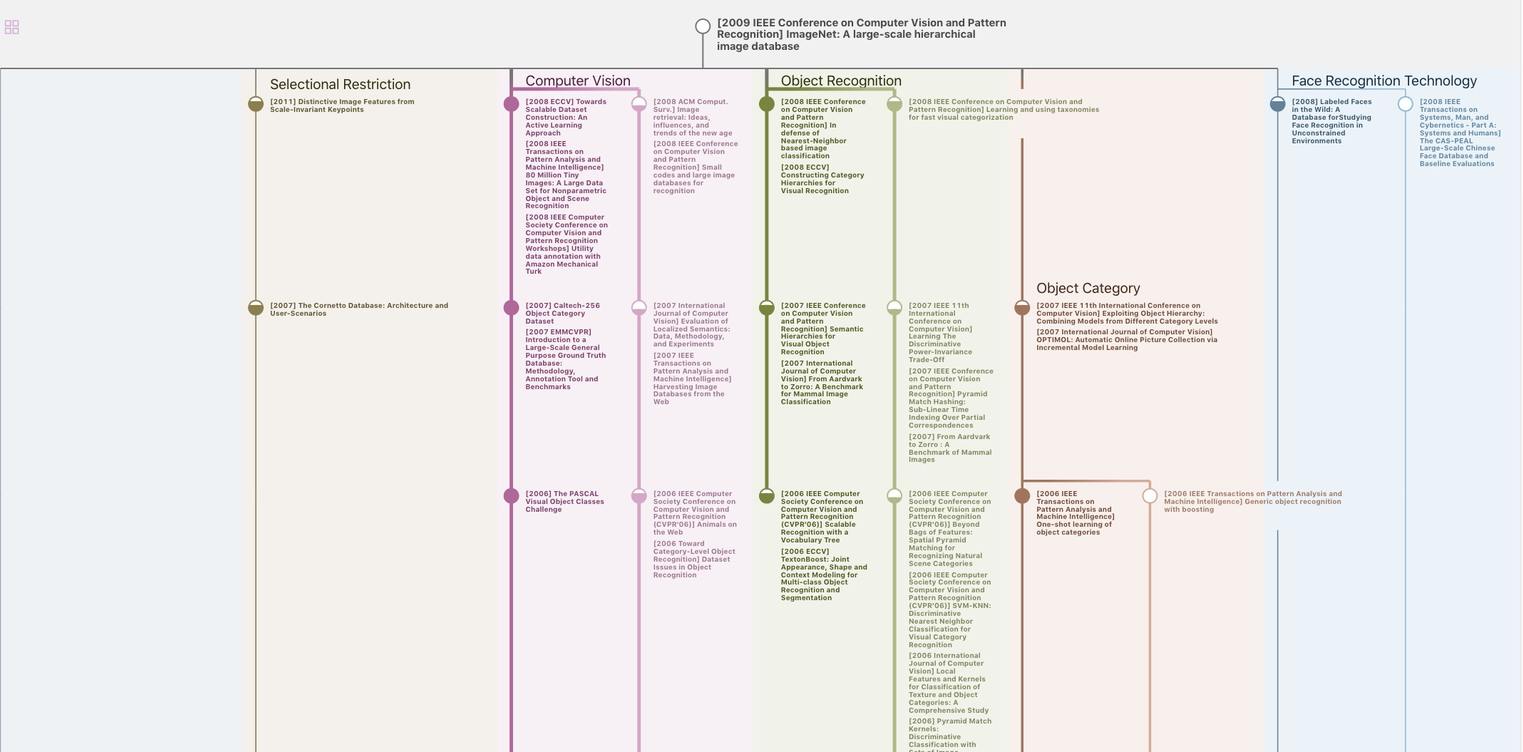
生成溯源树,研究论文发展脉络
Chat Paper
正在生成论文摘要