A High-dimensional Convergence Theorem for U-statistics with Applications to Kernel-based Testing
arXiv (Cornell University)(2023)
Abstract
We prove a convergence theorem for U-statistics of degree two, where the data dimension $d$ is allowed to scale with sample size $n$. We find that the limiting distribution of a U-statistic undergoes a phase transition from the non-degenerate Gaussian limit to the degenerate limit, regardless of its degeneracy and depending only on a moment ratio. A surprising consequence is that a non-degenerate U-statistic in high dimensions can have a non-Gaussian limit with a larger variance and asymmetric distribution. Our bounds are valid for any finite $n$ and $d$, independent of individual eigenvalues of the underlying function, and dimension-independent under a mild assumption. As an application, we apply our theory to two popular kernel-based distribution tests, MMD and KSD, whose high-dimensional performance has been challenging to study. In a simple empirical setting, our results correctly predict how the test power at a fixed threshold scales with $d$ and the bandwidth.
MoreTranslated text
Key words
testing,convergence,high-dimensional,u-statistics,kernel-based
AI Read Science
Must-Reading Tree
Example
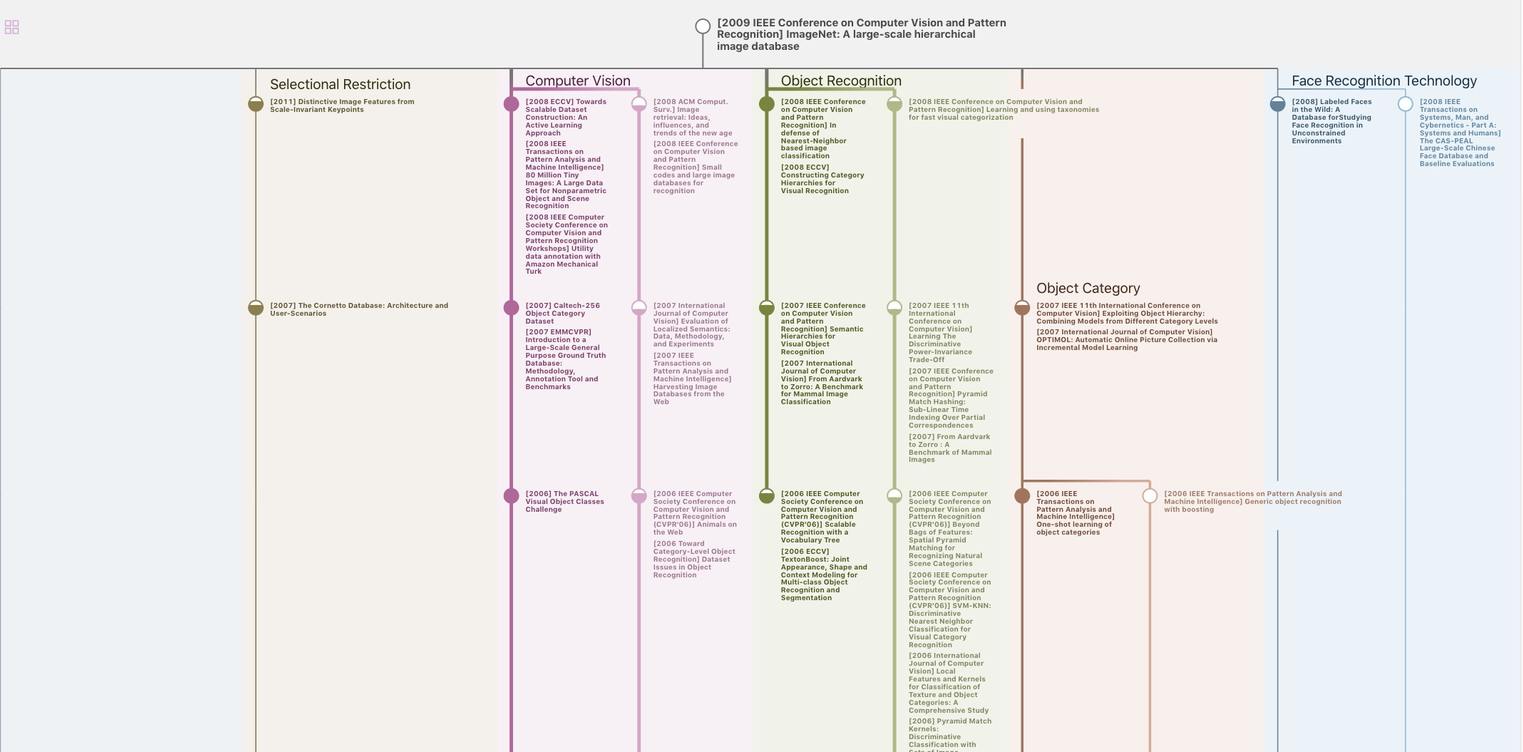
Generate MRT to find the research sequence of this paper
Chat Paper
Summary is being generated by the instructions you defined