"Why is this misleading?": Detecting News Headline Hallucinations with Explanations
WWW 2023(2023)
Abstract
Automatic headline generation enables users to comprehend ongoing news events promptly and has recently become an important task in web mining and natural language processing. With the growing need for news headline generation, we argue that the hallucination issue, namely the generated headlines being not supported by the original news stories, is a critical challenge for the deployment of this feature in web-scale systems Meanwhile, due to the infrequency of hallucination cases and the requirement of careful reading for raters to reach the correct consensus, it is difficult to acquire a large dataset for training a model to detect such hallucinations through human curation. In this work, we present a new framework named ExHalder to address this challenge for headline hallucination detection. ExHalder adapts the knowledge from public natural language inference datasets into the news domain and learns to generate natural language sentences to explain the hallucination detection results. To evaluate the model performance, we carefully collect a dataset with more than six thousand labeled pairs. Extensive experiments on this dataset and another six public ones demonstrate that ExHalder can identify hallucinated headlines accurately and justifies its predictions with human-readable natural language explanations.
MoreTranslated text
Key words
news headline hallucinations
AI Read Science
Must-Reading Tree
Example
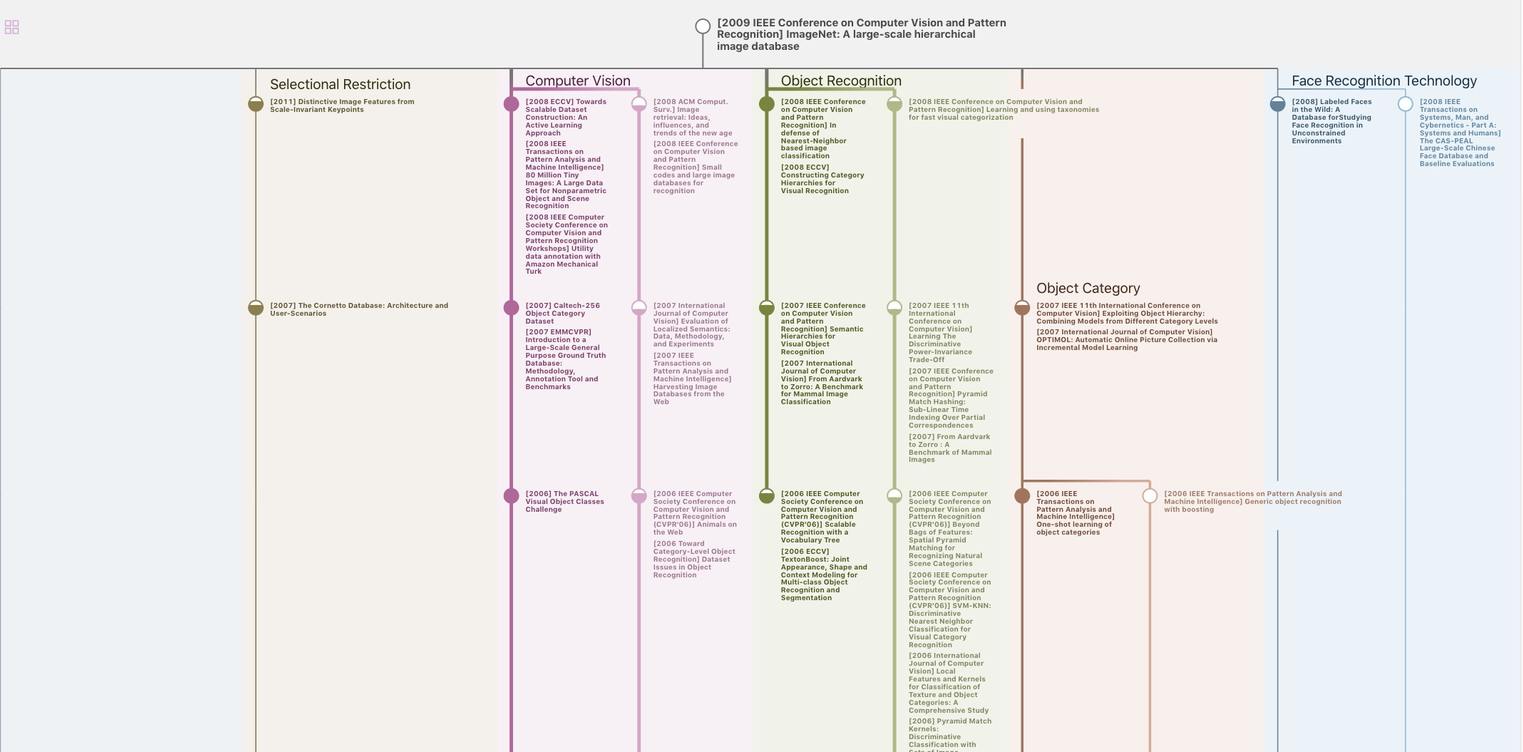
Generate MRT to find the research sequence of this paper
Chat Paper
Summary is being generated by the instructions you defined