Transfer Learning for Bayesian Optimization: A Survey
arxiv(2023)
摘要
A wide spectrum of design and decision problems, including parameter tuning, A/B testing and drug design, intrinsically are instances of black-box optimization. Bayesian optimization (BO) is a powerful tool that models and optimizes such expensive "black-box" functions. However, at the beginning of optimization, vanilla Bayesian optimization methods often suffer from slow convergence issue due to inaccurate modeling based on few trials. To address this issue, researchers in the BO community propose to incorporate the spirit of transfer learning to accelerate optimization process, which could borrow strength from the past tasks (source tasks) to accelerate the current optimization problem (target task). This survey paper first summarizes transfer learning methods for Bayesian optimization from four perspectives: initial points design, search space design, surrogate model, and acquisition function. Then it highlights its methodological aspects and technical details for each approach. Finally, it showcases a wide range of applications and proposes promising future directions.
更多查看译文
关键词
bayesian optimization,transfer learning
AI 理解论文
溯源树
样例
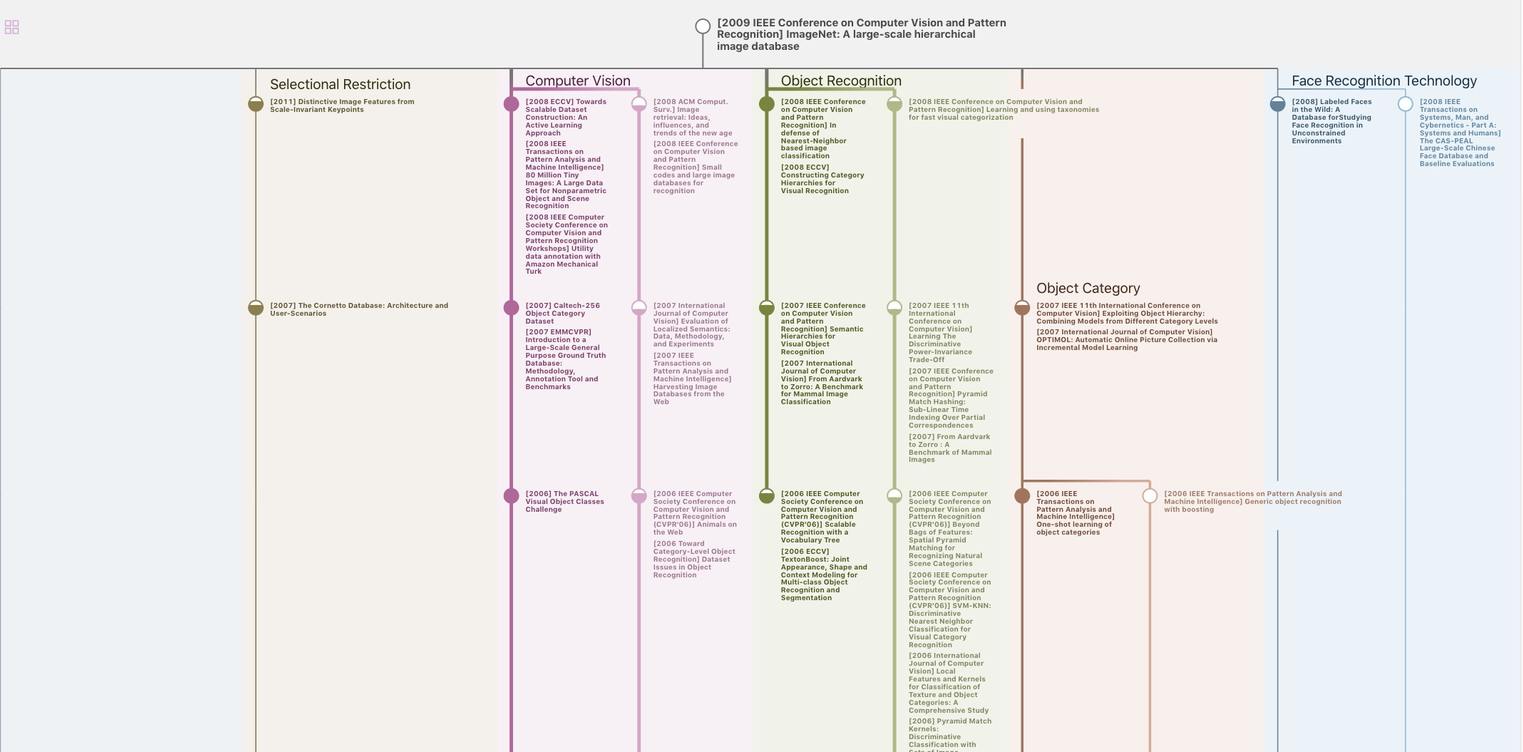
生成溯源树,研究论文发展脉络
Chat Paper
正在生成论文摘要