Mean Field Optimization Problem Regularized by Fisher Information
arxiv(2023)
摘要
Recently there is a rising interest in the research of mean field optimization, in particular because of its role in analyzing the training of neural networks. In this paper by adding the Fisher Information as the regularizer, we relate the regularized mean field optimization problem to a so-called mean field Schrodinger dynamics. We develop an energy-dissipation method to show that the marginal distributions of the mean field Schrodinger dynamics converge exponentially quickly towards the unique minimizer of the regularized optimization problem. Remarkably, the mean field Schrodinger dynamics is proved to be a gradient flow on the probability measure space with respect to the relative entropy. Finally we propose a Monte Carlo method to sample the marginal distributions of the mean field Schrodinger dynamics.
更多查看译文
关键词
fisher information,optimization
AI 理解论文
溯源树
样例
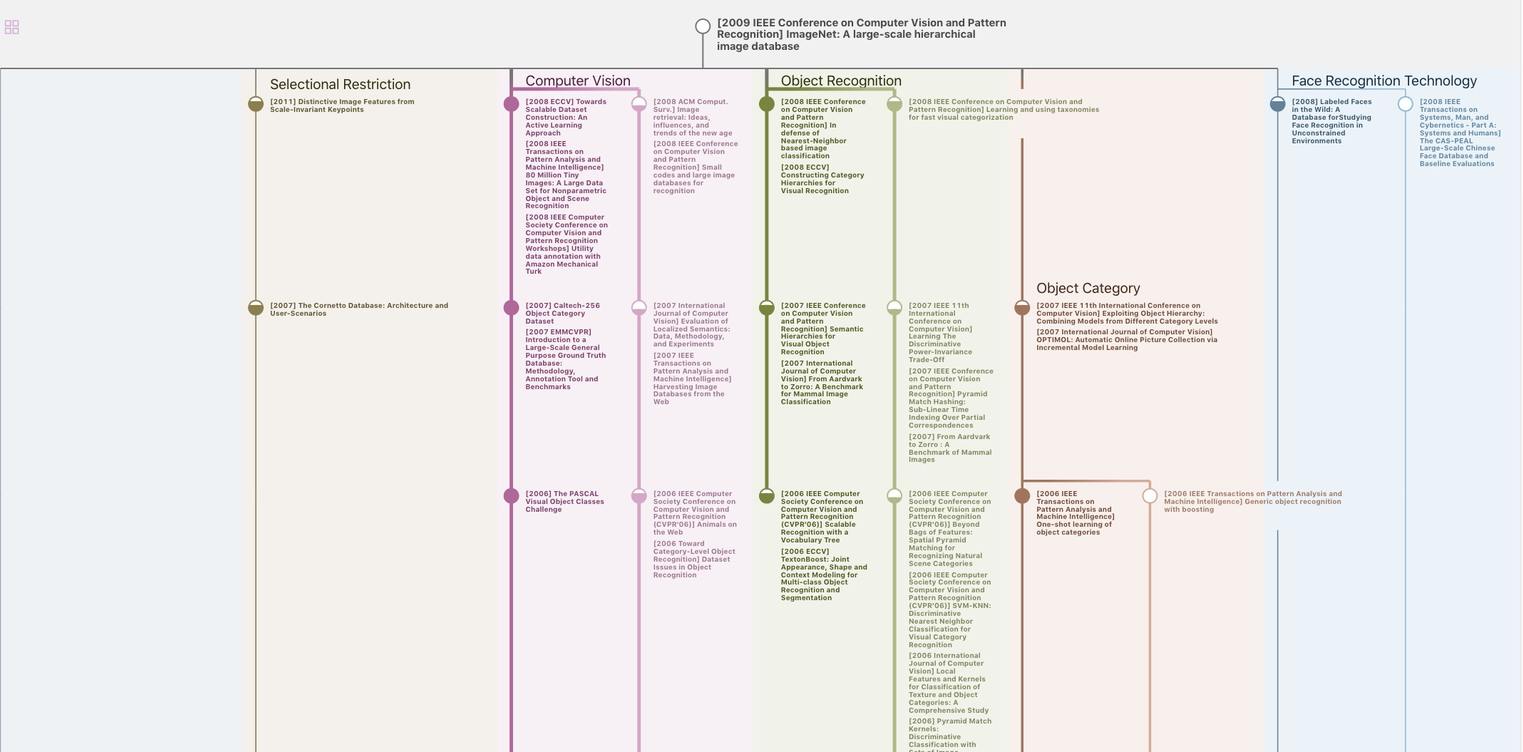
生成溯源树,研究论文发展脉络
Chat Paper
正在生成论文摘要