MarioGPT: Open-Ended Text2Level Generation through Large Language Models
CoRR(2023)
摘要
Procedural Content Generation (PCG) algorithms provide a technique to generate complex and diverse environments in an automated way. However, while generating content with PCG methods is often straightforward, generating meaningful content that reflects specific intentions and constraints remains challenging. Furthermore, many PCG algorithms lack the ability to generate content in an open-ended manner. Recently, Large Language Models (LLMs) have shown to be incredibly effective in many diverse domains. These trained LLMs can be fine-tuned, re-using information and accelerating training for new tasks. In this work, we introduce MarioGPT, a fine-tuned GPT2 model trained to generate tile-based game levels, in our case Super Mario Bros levels. We show that MarioGPT can not only generate diverse levels, but can be text-prompted for controllable level generation, addressing one of the key challenges of current PCG techniques. As far as we know, MarioGPT is the first text-to-level model. We also combine MarioGPT with novelty search, enabling it to generate diverse levels with varying play-style dynamics (i.e. player paths). This combination allows for the open-ended generation of an increasingly diverse range of content.
更多查看译文
关键词
text2level generation,language,models,open-ended
AI 理解论文
溯源树
样例
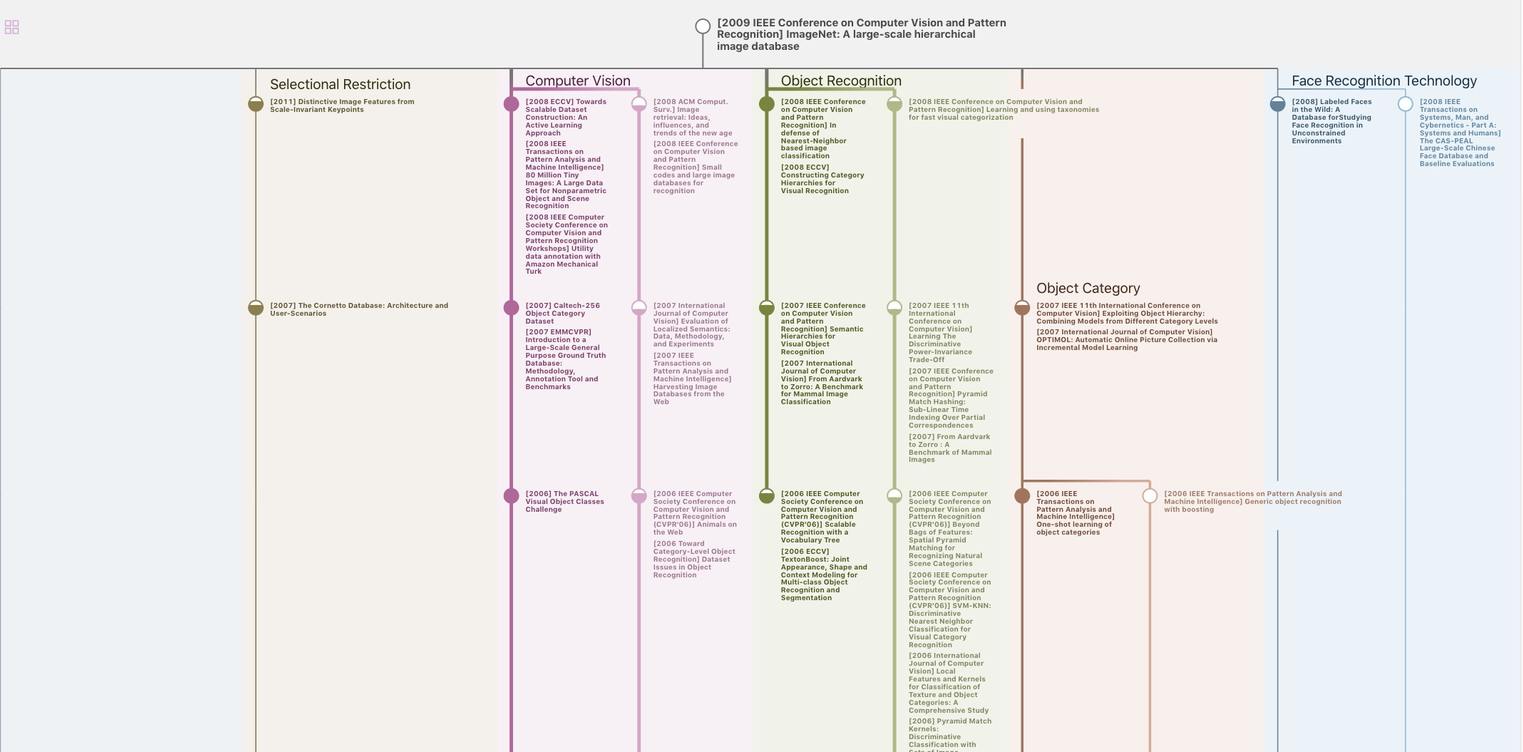
生成溯源树,研究论文发展脉络
Chat Paper
正在生成论文摘要