Learning from Noisy Crowd Labels with Logics
arxiv(2023)
摘要
This paper explores the integration of symbolic logic knowledge into deep neural networks for learning from noisy crowd labels. We introduce Logic-guided Learning from Noisy Crowd Labels (Logic-LNCL), an EM-alike iterative logic knowledge distillation framework that learns from both noisy labeled data and logic rules of interest. Unlike traditional EM methods, our framework contains a ``pseudo-E-step'' that distills from the logic rules a new type of learning target, which is then used in the ``pseudo-M-step'' for training the classifier. Extensive evaluations on two real-world datasets for text sentiment classification and named entity recognition demonstrate that the proposed framework improves the state-of-the-art and provides a new solution to learning from noisy crowd labels.
更多查看译文
关键词
noisy crowd labels,logics,learning
AI 理解论文
溯源树
样例
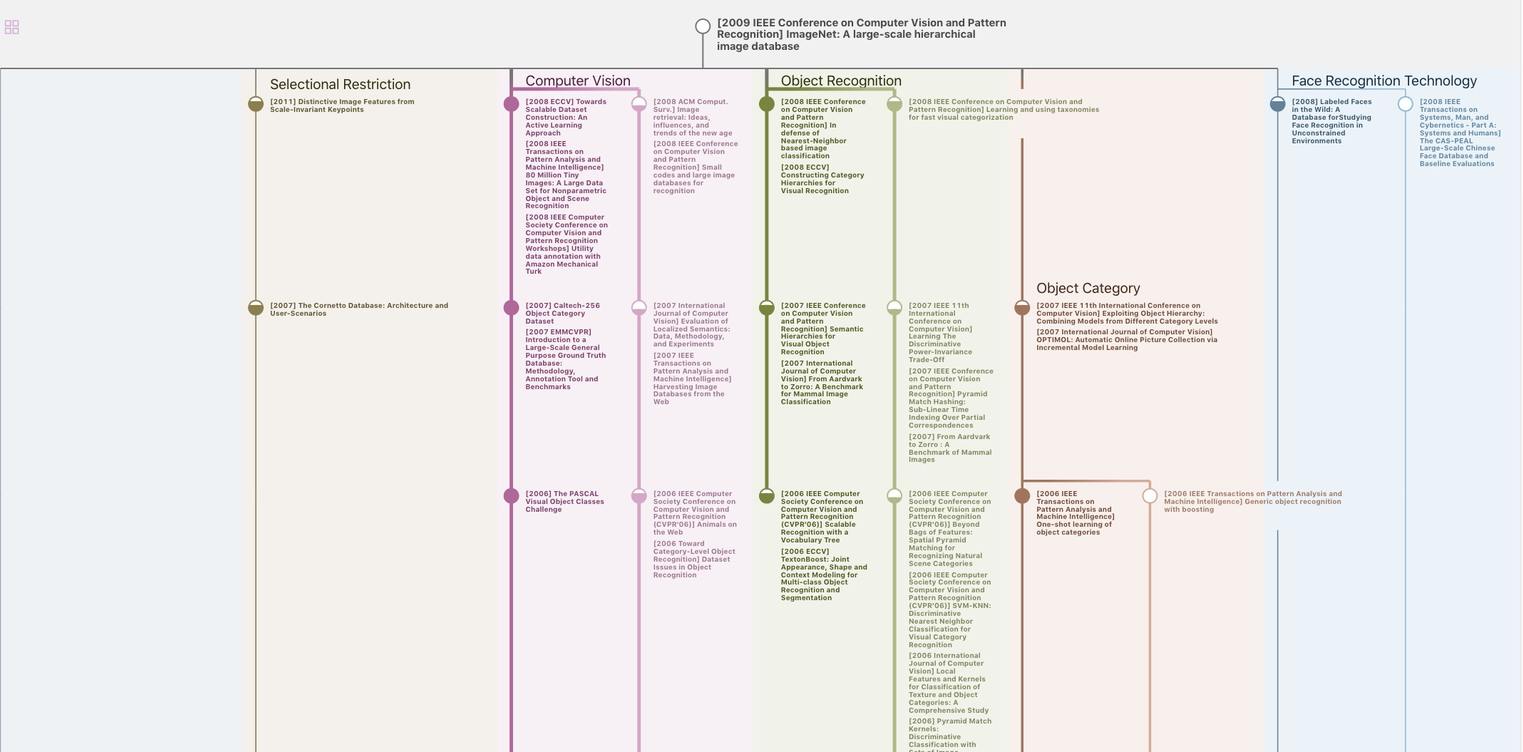
生成溯源树,研究论文发展脉络
Chat Paper
正在生成论文摘要