EnergyShield: Provably-Safe Offloading of Neural Network Controllers for Energy Efficiency
PROCEEDINGS OF THE 2023 ACM/IEEE 14TH INTERNATIONAL CONFERENCE ON CYBER-PHYSICAL SYSTEMS, WITH CPS-IOTWEEK 2023(2023)
摘要
To mitigate the high energy demand of Neural Network (NN) based Autonomous Driving Systems (ADSs), we consider the problem of offloading NN controllers from the ADS to nearby edge-computing infrastructure, but in such a way that formal vehicle safety properties are guaranteed. In particular, we propose the EnergyShield framework, which repurposes a controller "shield" as a low-power runtime safety monitor for the ADS vehicle. Specifically, the shield in EnergyShield provides not only safety interventions but also a formal, state-based quantification of the tolerable edge response time before vehicle safety is compromised. Using EnergyShield, an ADS can then save energy by wirelessly offloading NN computations to edge computers, while still maintaining a formal guarantee of safety until it receives a response (on-vehicle hardware provides a just-in-time fail safe). To validate the benefits of EnergyShield, we implemented and tested it in the Carla simulation environment. Our results show that EnergyShield maintains safe vehicle operation while providing significant energy savings compared to on-vehicle NN evaluation: from 24% to 54% less energy across a range of wireless conditions and edge delays.
更多查看译文
关键词
Formal Methods,Vehicular,Edge Computing,Autonomous Vehicles,Provable safety,Offloading,Autonomous Driving Systems
AI 理解论文
溯源树
样例
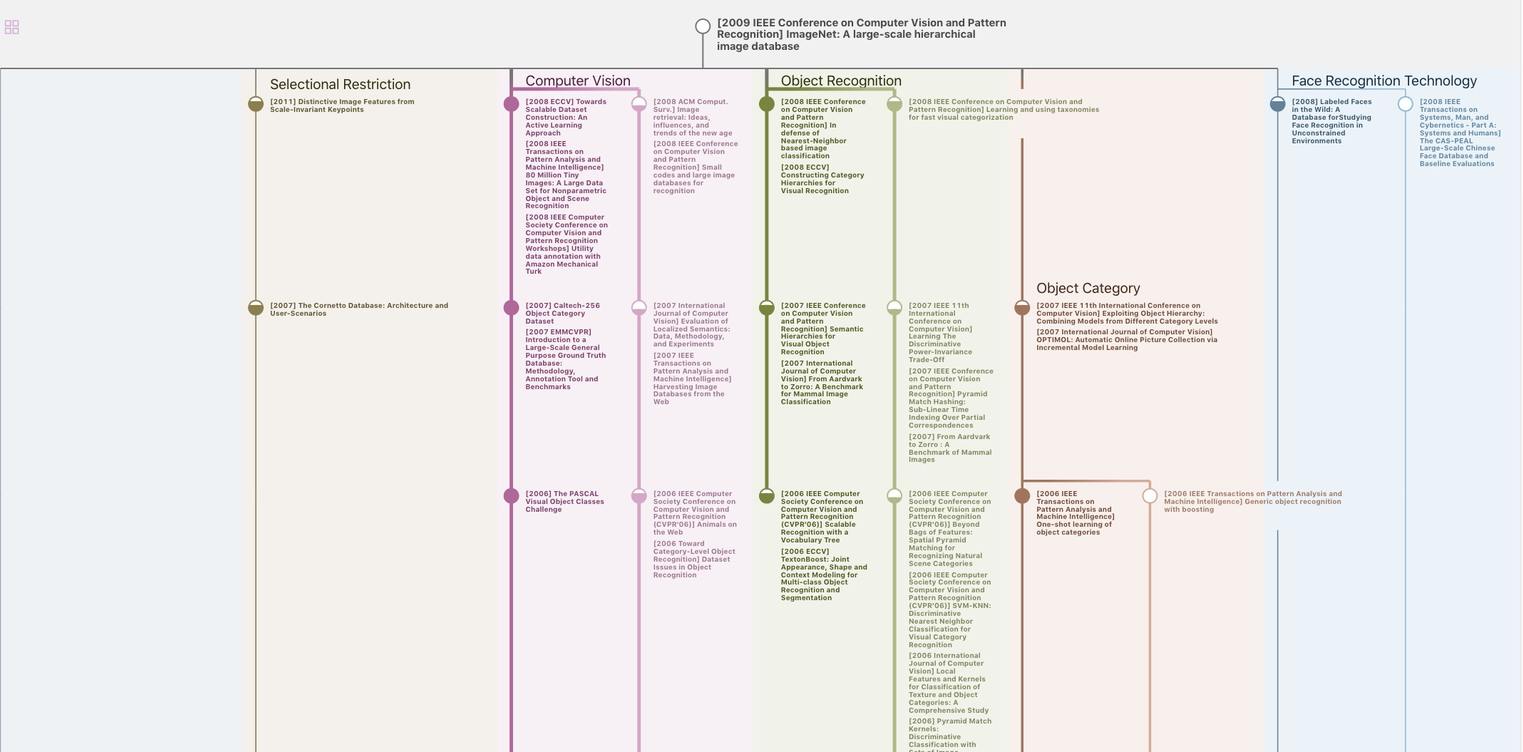
生成溯源树,研究论文发展脉络
Chat Paper
正在生成论文摘要