A framework for mediation analysis with massive data
Stat Comput(2023)
摘要
During the past few years, mediation analysis has gained increasing popularity across various research fields. The primary objective of mediation analysis is to examine the direct impact of exposure on outcome, as well as the indirect effects that occur along the pathways from exposure to outcome. There has been a great number of articles that applied mediation analysis to data from hundreds or thousands of individuals. With the rapid development of technology, the volume of avaliable data increases exponentially, which brings new challenges to researchers. Directly conducting statistical analysis for large datasets is often computationally infeasible. Nonetheless, there is a paucity of findings regarding mediation analysis in the context of big data. In this paper, we propose utilizing subsampled double bootstrap and divide-and-conquer algorithms to conduct statistical mediation analysis on large-scale datasets. The proposed algorithms offer a significant enhancement in computational efficiency over traditional bootstrap confidence interval and Sobel test, while simultaneously ensuring desirable confidence interval coverage and power. We conducted extensive numerical simulations to evaluate the performance of our method. The practical applicability of our approach is demonstrated through two real-world data examples.
更多查看译文
关键词
Big data, Divide-and-conquer, Mediation effects, Structural equation modeling, Subsampled double bootstrap
AI 理解论文
溯源树
样例
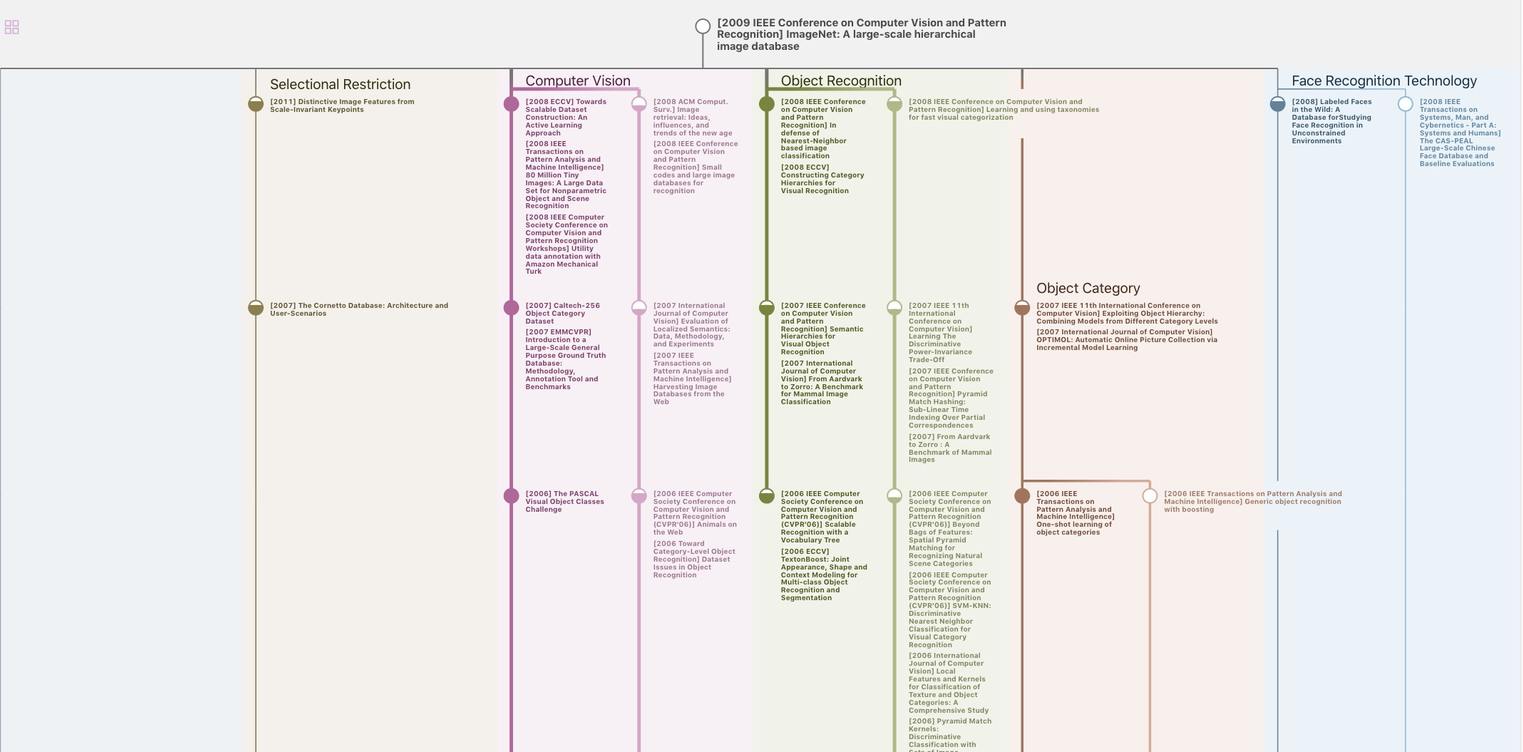
生成溯源树,研究论文发展脉络
Chat Paper
正在生成论文摘要