Parameters for > 300 million Gaia stars: Bayesian inference vs. machine learning
CoRR(2023)
摘要
The Gaia Data Release 3 (DR3), published in June 2022, delivers a diverse set of astrometric, photometric, and spectroscopic measurements for more than a billion stars. The wealth and complexity of the data makes traditional approaches for estimating stellar parameters for the full Gaia dataset almost prohibitive. We have explored different supervised learning methods for extracting basic stellar parameters as well as distances and line-of-sight extinctions, given spectro-photo-astrometric data (including also the new Gaia XP spectra). For training we use an enhanced high-quality dataset compiled from Gaia DR3 and ground-based spectroscopic survey data covering the whole sky and all Galactic components. We show that even with a simple neural-network architecture or tree-based algorithm (and in the absence of Gaia XP spectra), we succeed in predicting competitive results (compared to Bayesian isochrone fitting) down to faint magnitudes. We will present a new Gaia DR3 stellar-parameter catalogue obtained using the currently best-performing machine-learning algorithm for tabular data, XGBoost, in the near future.
更多查看译文
关键词
gaia stars,bayesian inference,machine
learning
AI 理解论文
溯源树
样例
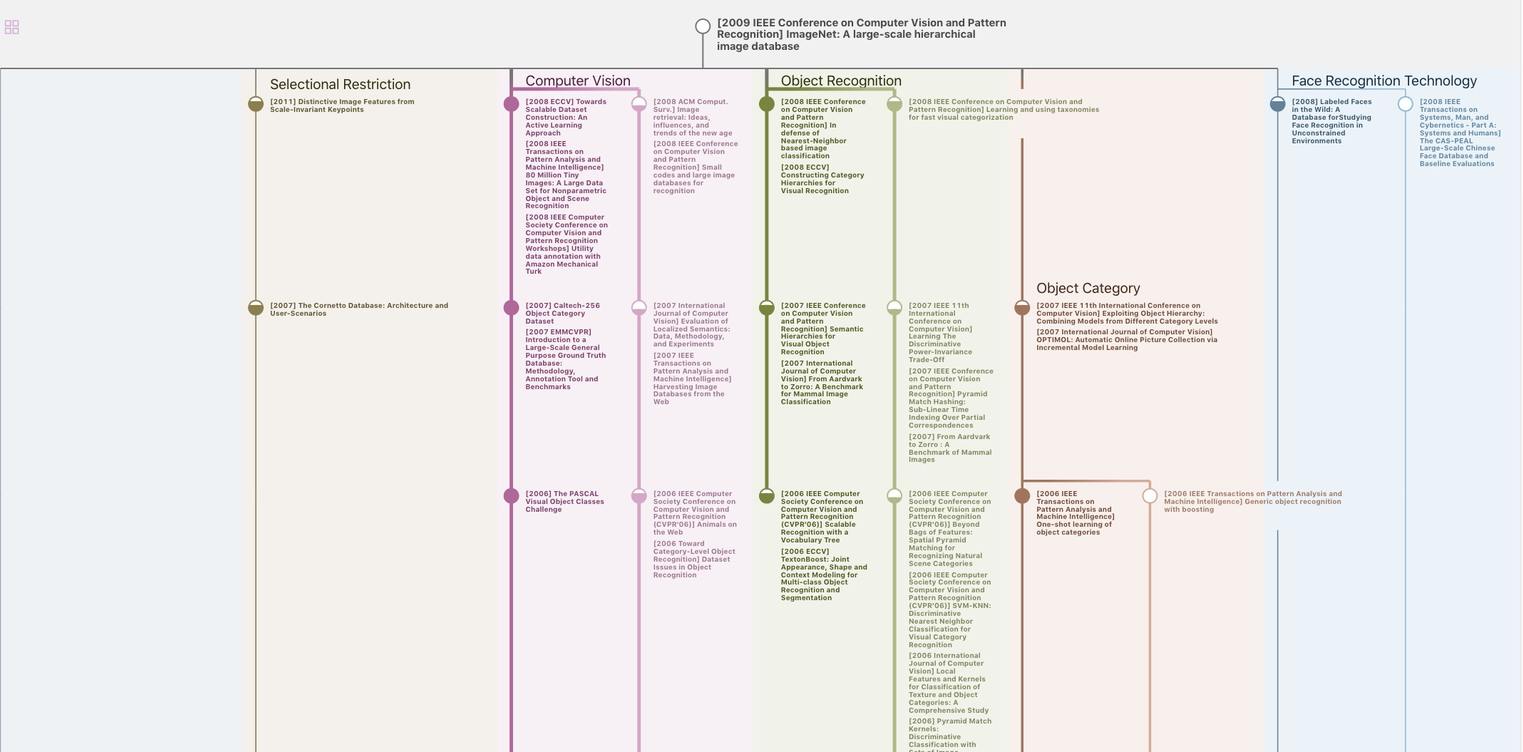
生成溯源树,研究论文发展脉络
Chat Paper
正在生成论文摘要