Semiconductor Fab Scheduling with Self-Supervised and Reinforcement Learning
CoRR(2023)
摘要
Semiconductor manufacturing is a notoriously complex and costly multi-step process involving a long sequence of operations on expensive and quantity-limited equipment. Recent chip shortages and their impacts have highlighted the importance of semiconductors in the global supply chains and how reliant on those our daily lives are. Due to the investment cost, environmental impact, and time scale needed to build new factories, it is difficult to ramp up production when demand spikes. This work introduces a method to successfully learn to schedule a semiconductor manufacturing facility more efficiently using deep reinforcement and self-supervised learning. We propose the first adaptive scheduling approach to handle complex, continuous, stochastic, dynamic, modern semiconductor manufacturing models. Our method outperforms the traditional hierarchical dispatching strategies typically used in semiconductor manufacturing plants, substantially reducing each order's tardiness and time until completion. As a result, our method yields a better allocation of resources in the semiconductor manufacturing process.
更多查看译文
关键词
Self-supervised Learning,Semiconductor Fab,Manufacturing Process,Deep Reinforcement Learning,Semiconductor Industry,Hierarchical Strategy,Neural Network,Objective Function,Processing Time,Transition State,Attention Mechanism,Stochastic Gradient Descent,State Representation,Evolutionary Strategy,Electricity Consumption,Reward Function,Markov Decision Process,Setup Time,Current Operation,Policy Network,Job Shop Scheduling Problem,Work Centre,Machine Breakdown,Regularization Constant,Trained Agent,Critical Ratio,Resource Usage,Optimal Combination,Horizon,Reinforcement Learning Agent
AI 理解论文
溯源树
样例
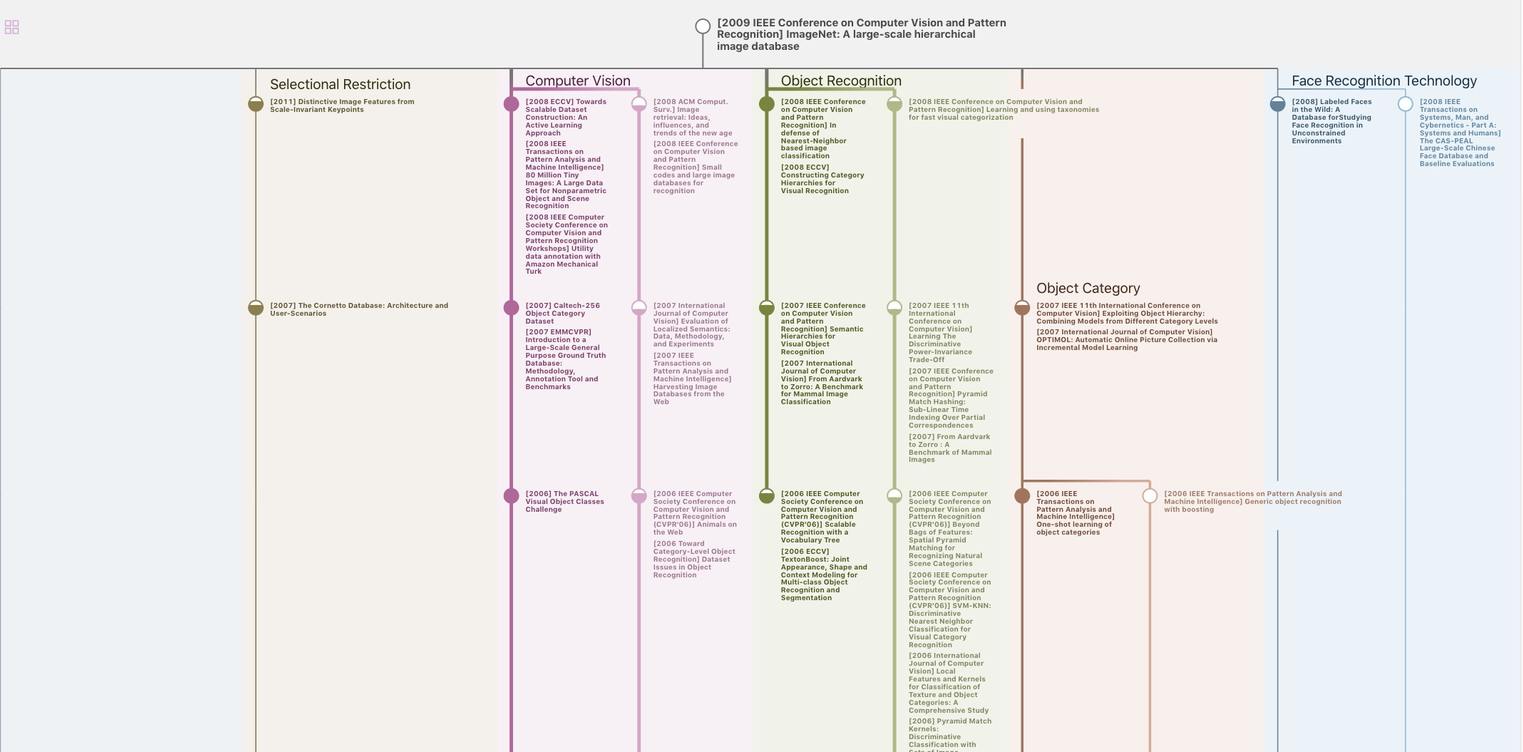
生成溯源树,研究论文发展脉络
Chat Paper
正在生成论文摘要