Partial least squares, steepest descent, and conjugate gradient for regularized predictive modeling
AICHE JOURNAL(2023)
摘要
In this article, we explore the connection of partial least squares (PLS) to other regularized regression algorithms including the Lasso and ridge regression, and consider a steepest descent alternative to the PLS algorithm. First, the PLS latent variable analysis is emphasized and formulated as a standalone procedure. The PLS connections to the conjugate gradient, Krylov space, and the Cayley-Hamilton theorem for matrix pseudo-inverse are explored based on known results in the literature. Comparison of PLS with the Lasso and ridge regression are given in terms of the different resolutions along the regularization paths, leading to an explanation of why PLS sometimes does not outperform the Lasso and ridge regression. As an attempt to increase resolutions along the regularization paths, a steepest descent PLS is formulated as a regularized regression alternative to PLS and is compared to other regularized algorithms via simulations and an industrial case study.
更多查看译文
关键词
conjugate gradient,latent variable analysis,partial least squares analysis,partial least squares regression,regularized regression,steepest descent
AI 理解论文
溯源树
样例
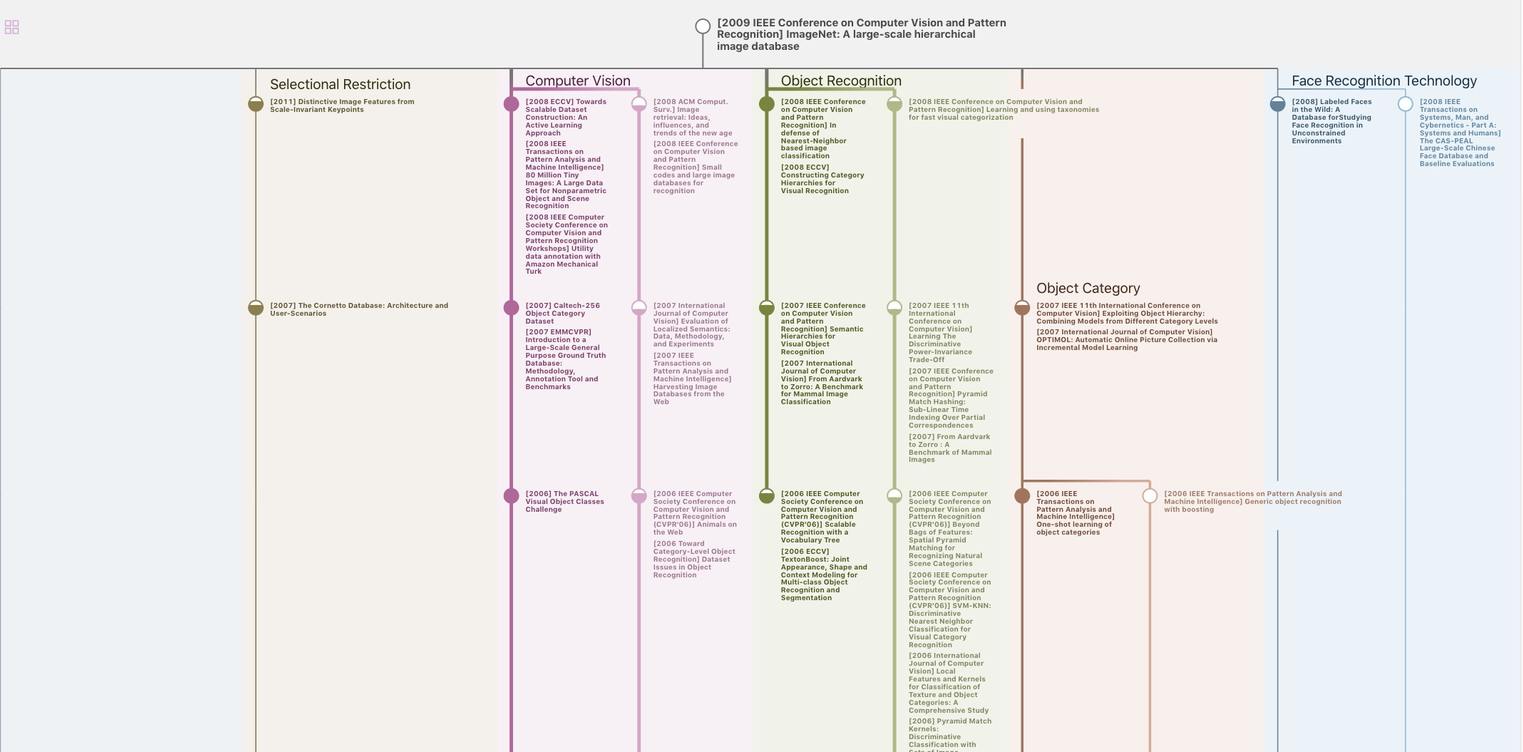
生成溯源树,研究论文发展脉络
Chat Paper
正在生成论文摘要