A novel milling parameter optimization method based on improved deep reinforcement learning considering machining cost
JOURNAL OF MANUFACTURING PROCESSES(2022)
摘要
The cutting parameters in the part machining process have a great impact on the energy efficiency and economy of the machining system. The cutting parameters in traditional machining are often selected according to the operator's experience, which lack attentions to energy saving and economy. Therefore, a milling process parameter optimization method based on deep reinforcement learning (DRL) is proposed in this paper. Taking the machining cost composed of cutting energy efficiency and machining time cost as the optimization goal, the spindle speed and feed speed under the combination of different cutting depth and cutting width parameters are optimized. Firstly, the machine tool energy consumption model is established by back propagation neural network (BPNN) regression method to realize the continuity of machine tool energy consumption state predic-tion, and the processing cost model is established as the optimization objective function. Then, the process parameter optimization problem is formally expressed as a Markov decision process (MDP), and the corre-sponding states, actions, reward functions and constraints are defined. Finally, combined with the machine tool power consumption model and machining cost model, the simulation environment is established, and the BP-TD3 method is proposed to solve the Markov decision problem of milling parameter optimization. Taking the machining center as an example, the aluminum alloy workpiece is milled. Compared with the classical opti-mization algorithm, the proposed method can save 95 % optimization calculation time, and ensure that the average processing cost after optimization is close to the minimum processing cost obtained by the classical optimization algorithm.
更多查看译文
关键词
Milling parameters optimization,Processing energy efficiency,Processing cost,Simulation environment,Deep reinforcement learning
AI 理解论文
溯源树
样例
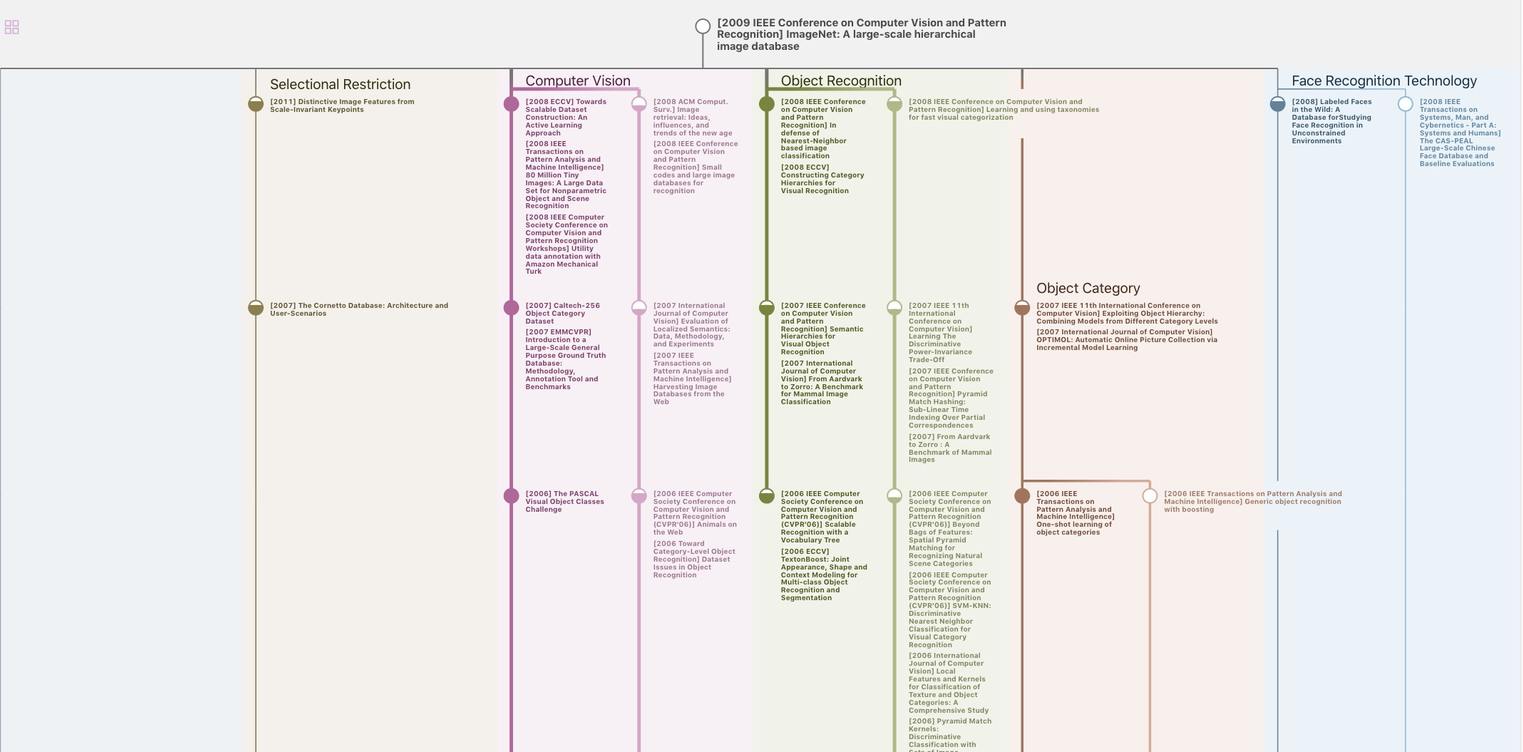
生成溯源树,研究论文发展脉络
Chat Paper
正在生成论文摘要