ECG-based Cross-Subject Mental Stress Detection via Discriminative Clustering Enhanced Adversarial Domain Adaptation
2022 16TH IEEE INTERNATIONAL CONFERENCE ON SIGNAL PROCESSING (ICSP2022), VOL 1(2022)
摘要
Mental stress detection based on Electrocardiogram (ECG) and deep learning has been widely researched recently. However, user-specific models trained on known subjects cannot precisely predict the mental stress conditions of new subjects because of the individual differences of ECG among various subjects. Unsupervised domain adaptation (UDA) tackles the problem by learning domain-invariant feature representations for the ECG data of both the known source subjects and the new target subjects. However, in the cross-subject mental stress detection scenario, these methods generally fail to deal with ambiguous target data that resides around the boundaries of different stress levels. To address the issue, we propose a novel ECG-based cross-subject mental stress detection method, named Discriminative Clustering Enhanced Adversarial Domain Adaptation (DC-ADA). Based on existing bi-classifier adversarial UDA methods, we propose to enhance the training step by incorporating a self-supervised loss on the target data. Specifically, we obtain the pseudo labels of target data through a clustering-based strategy, and leverage those labels as the supervision to encourage the ambiguous target data to be classified into correct stress levels. We compare our method with the leave-one-out methods and other UDA methods on three public mental stress datasets, and the experimental results validates the superiority of our method.
更多查看译文
关键词
Electrocardiogram,Mental Stress Detection,Unsupervised Domain Adaptation,Discriminative Clustering
AI 理解论文
溯源树
样例
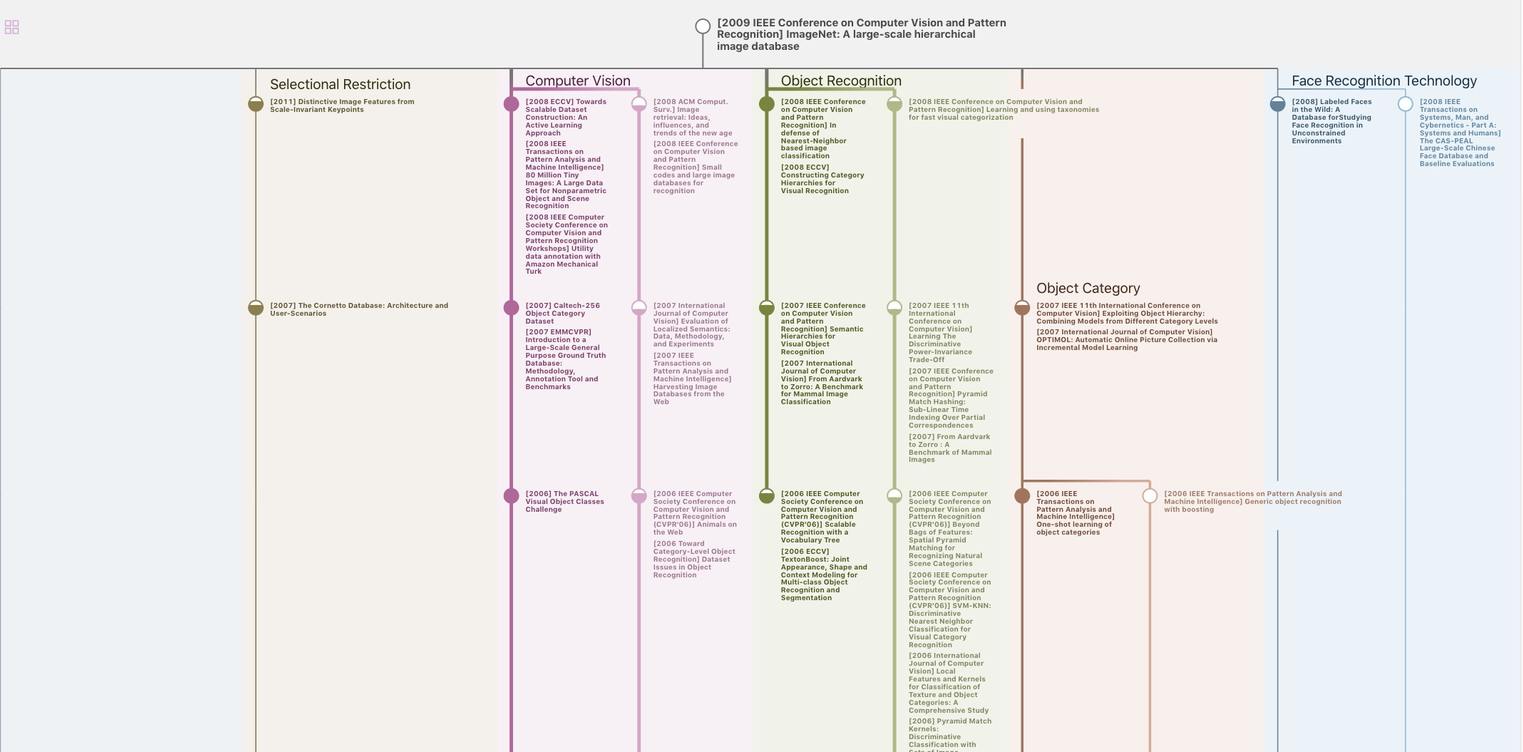
生成溯源树,研究论文发展脉络
Chat Paper
正在生成论文摘要