An energy-efficient and accuracy-aware edge computing framework for heart arrhythmia detection: A joint model selection and task offloading approach
JOURNAL OF SUPERCOMPUTING(2022)
摘要
IoT-based arrhythmia detection is one of the delay-sensitive applications that can benefit from edge computing to reduce the processing latency. Choosing the right offloading strategy in heterogeneous systems such as arrhythmia detection systems where the user’s health state, sensor’s battery status, and sensor/edges’ processing power may vary is very challenging and has a significant impact on the quality of service and the energy cost. In this paper, we introduce the problem of simultaneous computation offloading and detection model selection to trade-off the accuracy with the performance and the energy cost based on the current health state of the users and the processing and battery capacities of nodes. Experimental results indicate that the proposed method increases the percentage of users serviced by the system by 17.3%, 98.79%, and 1.21% compared to first-fit, edge-only, and cloud-only approaches and improves energy consumption by 6.9% and 47.9% compared to first-fit and cloud-only approaches, respectively.
更多查看译文
关键词
Internet of Things,Edge computing,Energy efficiency,Computation offloading,Heart arrhythmia detection
AI 理解论文
溯源树
样例
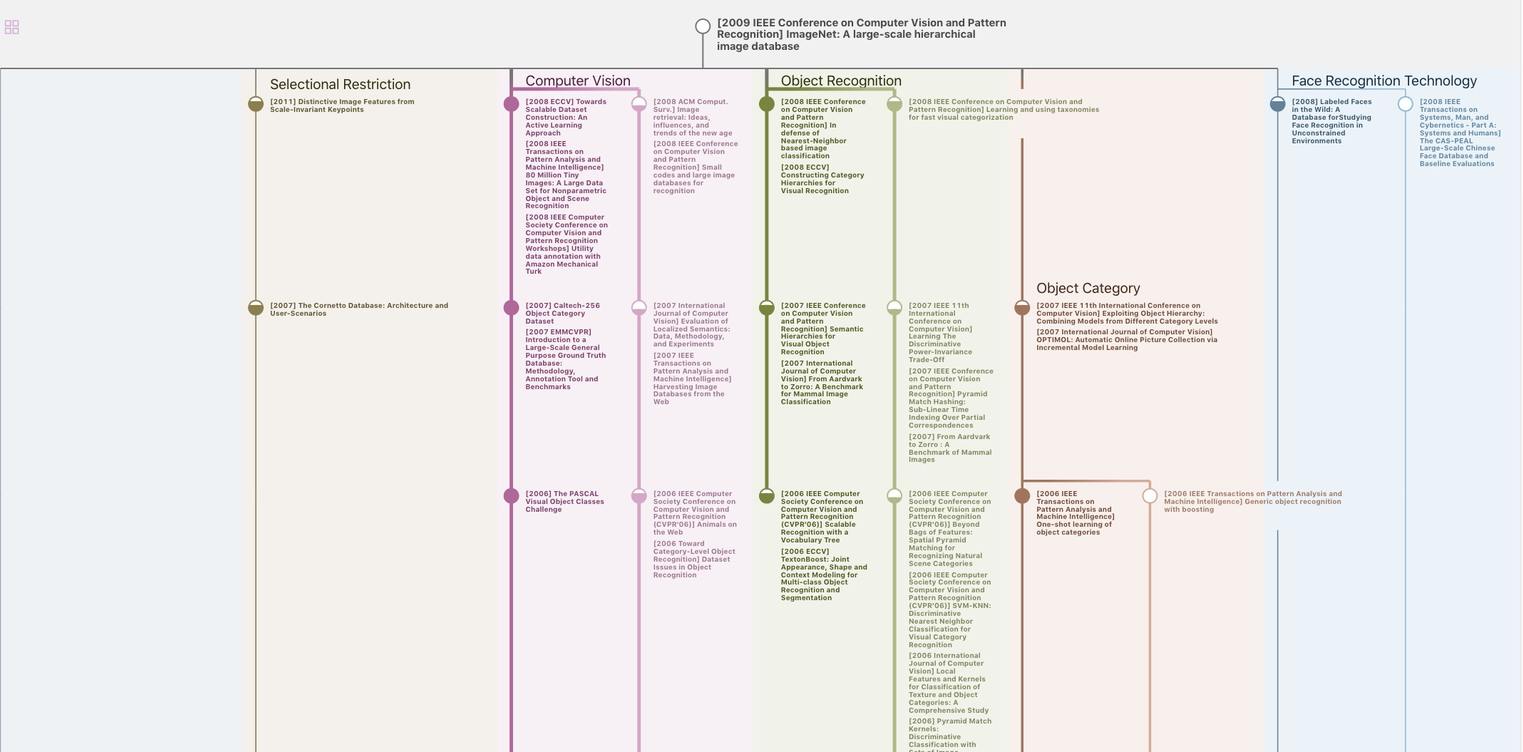
生成溯源树,研究论文发展脉络
Chat Paper
正在生成论文摘要