The use of artificial intelligence and time characteristics in the optimization of the structure of the volumetric percentage detection system independent of the scale value inside the pipe
APPLIED ARTIFICIAL INTELLIGENCE(2023)
摘要
When scale builds up in a transmission pipeline, it narrows the pipe's interior and causes losses in both power and efficiency. A noninvasive instrument based on gamma-ray attenuation is one of the most reliable diagnostic procedures for determining volumetric percentages in a variety of circumstances. A system with a NaI detector and dual-energy gamma generator simulations (Am-241 and (13)3 Ba radioisotopes) is recommended for simulating a volume percentage detection system utilizing Monte Carlo N particle (MCNP). Three-phase flow consisting of oil, water, and gas moves through a scaled pipe of variable wall thicknesses in a stratified flow regime with changing volume percentages. After gamma rays are emitted from one end of the pipe, a detector take in the photons coming from the other end. Four temporal features, including kurtosis and mean value of the square root (MSR), skewness, and waveform length (WL) picked up by the detector, were thus obtained. By training two GMDH neural networks with the aforementioned inputs, it is possible to forecast volumetric percentages with an RMSE of less than 0.90 and independently of scale thickness. The low error value, simplicity of the system, and reduction of design costs ensures the effectiveness of the suggested method and the advantages of employing this approach in the petroleum and petrochemical industries.
更多查看译文
关键词
volumetric percentage detection system,pipe,optimization,artificial intelligence
AI 理解论文
溯源树
样例
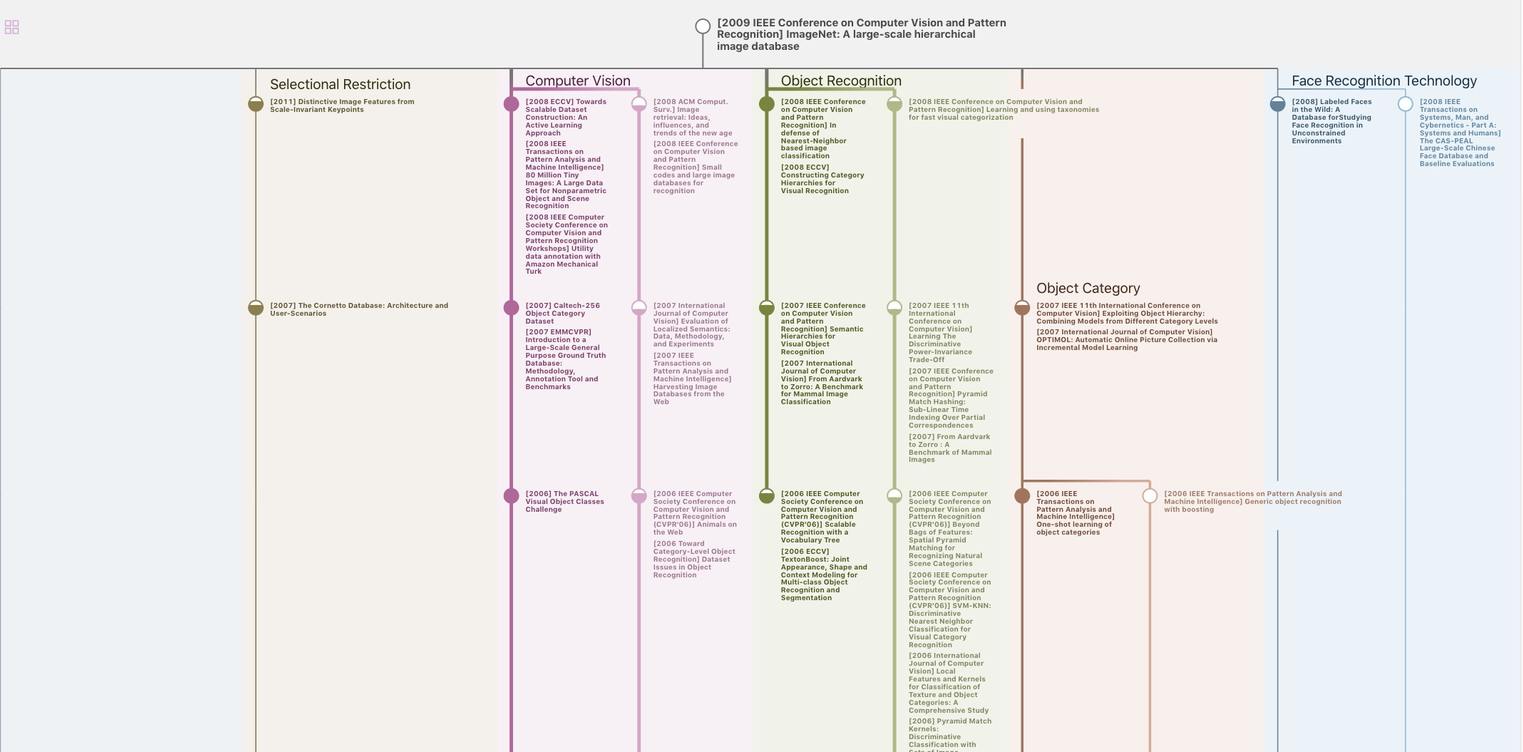
生成溯源树,研究论文发展脉络
Chat Paper
正在生成论文摘要