A multi-task learning model for non-intrusive load monitoring based on discrete wavelet transform
JOURNAL OF SUPERCOMPUTING(2023)
摘要
Non-intrusive load monitoring (NILM) is an algorithm that can help to present the power consumption of each domestic appliance by analyzing the total household consumption data. With NILM, the house does not need an additional monitoring equipment and the costs can therefore be reduced. Considering the few input features (usually aggregate power only), we proposed to use sliding windows, extracted different frequency bands through discrete wavelet transform of the power in each sliding window, and characterized the features of the middle of the sliding window, so as to perform multi-dimensional features input. The multi-dimensional inputs are put into a neural network consisting of one-dimensional convolution and Bi-directional Long Short-Term Memory for prediction. Also, in consideration of the states of the device switches, we designed a classification network that serves as the auxiliary network in multi-task learning, and designed the optimized loss function that works for the binary classification problem. The final output of the neural network is the product of the losses of the two subnets to achieve information sharing. The proposed feature extraction method and deep neural network were applied to the real-world household energy dataset UK Domestic Appliance-Level Electricity. Furthermore, we selected the F 1-score, mean absolute error, and signal aggregate error as the main indicators of algorithm performance evaluation. It is found that the performance is better than what the previous researchers did with subtask gated networks, with two standard error metrics (mean absolute error and signal aggregate error), respectively, improved by 25.15% and 17.83%. In addition, the average F 1-score increased by nearly 20% and the average mean absolute error and signal aggregate error decreased by 37.86% and 25.24%, respectively.
更多查看译文
关键词
Non-intrusive load monitoring (NILM),Power disaggregation,Multi-task learning,Discrete wavelet transform,Focal loss
AI 理解论文
溯源树
样例
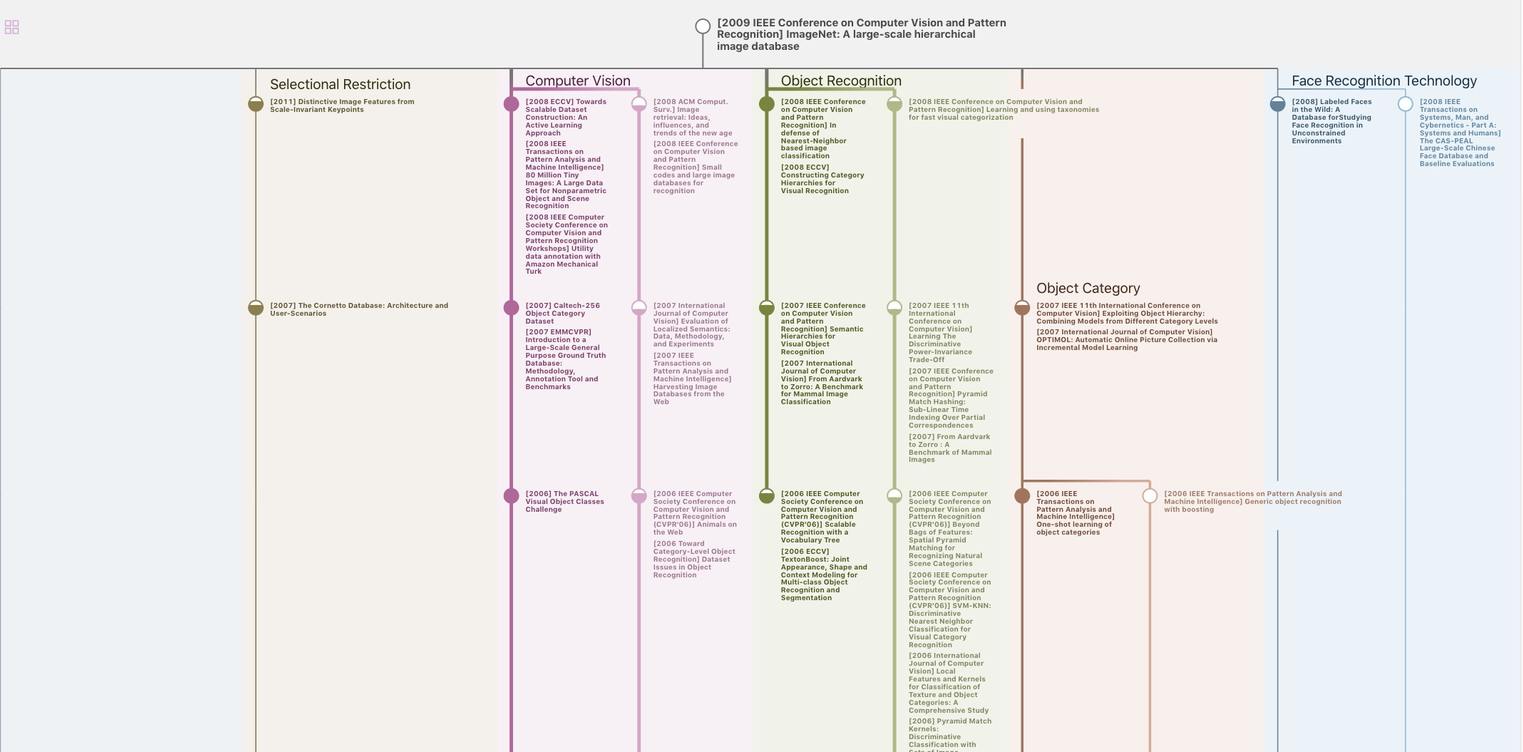
生成溯源树,研究论文发展脉络
Chat Paper
正在生成论文摘要