A novel feature and sample joint transfer learning method with feature selection in semi-supervised scenarios for identifying the sequence of some species with less known genetic data
SOFT COMPUTING(2023)
摘要
When identifying the sequence of some species using fewer known gene training data (named target domain), the data of closely related species and unlabeled data of the species (named source domain) could be considered for auxiliary training. However, there are differences in the statistical distribution of the feature space comprising of genetic data of different species. Therefore, this paper proposes a feature and sample jointed transfer (FSJT) method for semi-supervised scenarios, consisting of two modules. In the first module, the distance between the sample probability distribution functions in the feature space is taken as the optimization objective, and a hybrid balanced distribution adaptation method is constructed to transform the feature space of the two domains to increase the similarity between the domains. In the second module, the confidence of the unlabeled data in the target domain is defined and a self-learning sample transfer method is proposed to reduce the impact of samples having large differences in source-domain training data. Simultaneously, to select the suitable source-domain samples and the target domain when the sample size between the fields is very different, the transferred Lasso and the nearest-neighbor (TLR) feature selection method is proposed using FSJT. Then, the whole framework and algorithm flow of the TLR-FSJT model is presented and verified using the transfer learning standard dataset and ribonucleic acid data from GenBank database by comparing it with three machine learning methods and the FSJT model. Results show that the TLR-FSJT model has the highest accuracy in semi-supervised scenarios.
更多查看译文
关键词
Distribution adaptation method,Semi-supervised transfer learning,Feature and sample joint transfer learning method,Self-learning sample transfer method
AI 理解论文
溯源树
样例
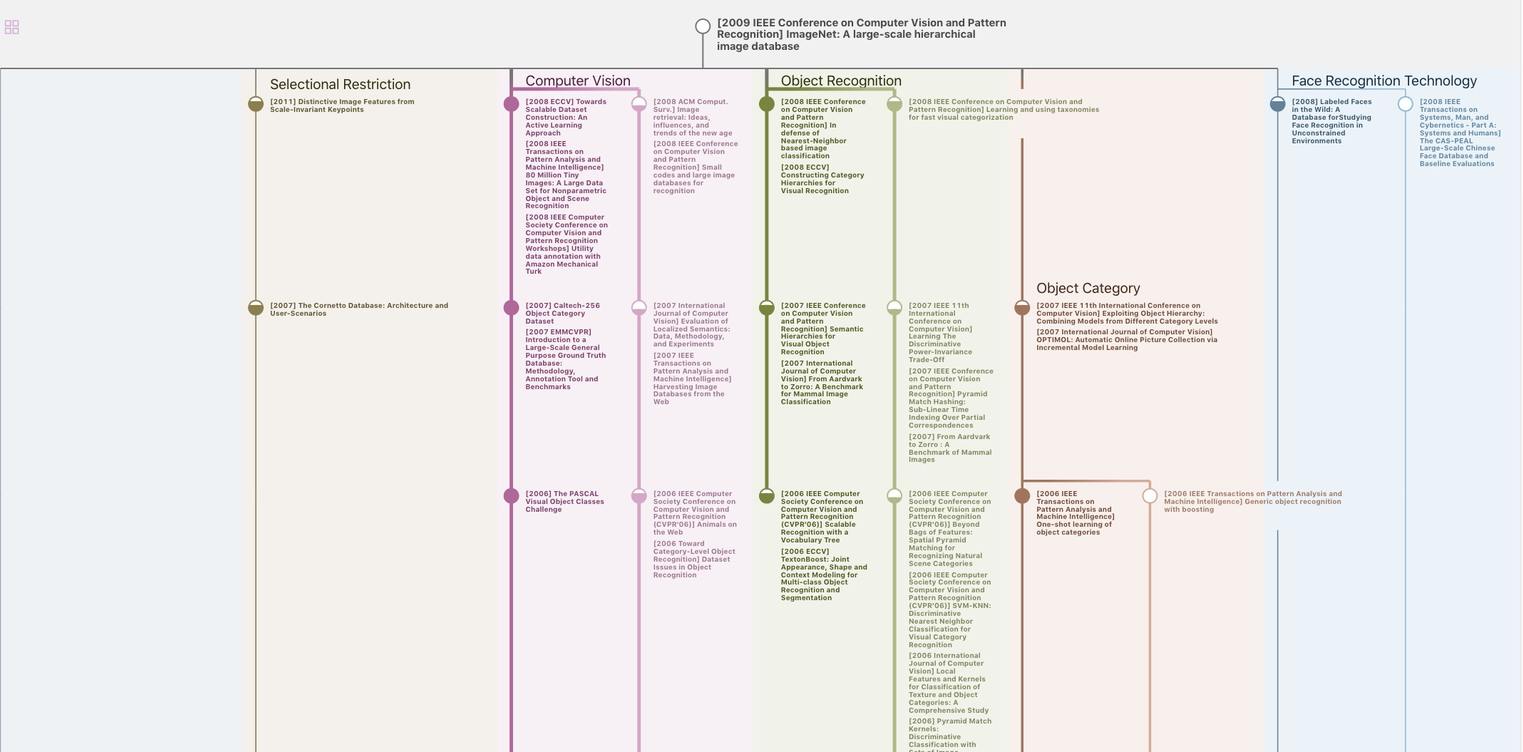
生成溯源树,研究论文发展脉络
Chat Paper
正在生成论文摘要